- お役立ち記事
- Basics of model predictive control technology and points for effective practical application
Basics of model predictive control technology and points for effective practical application
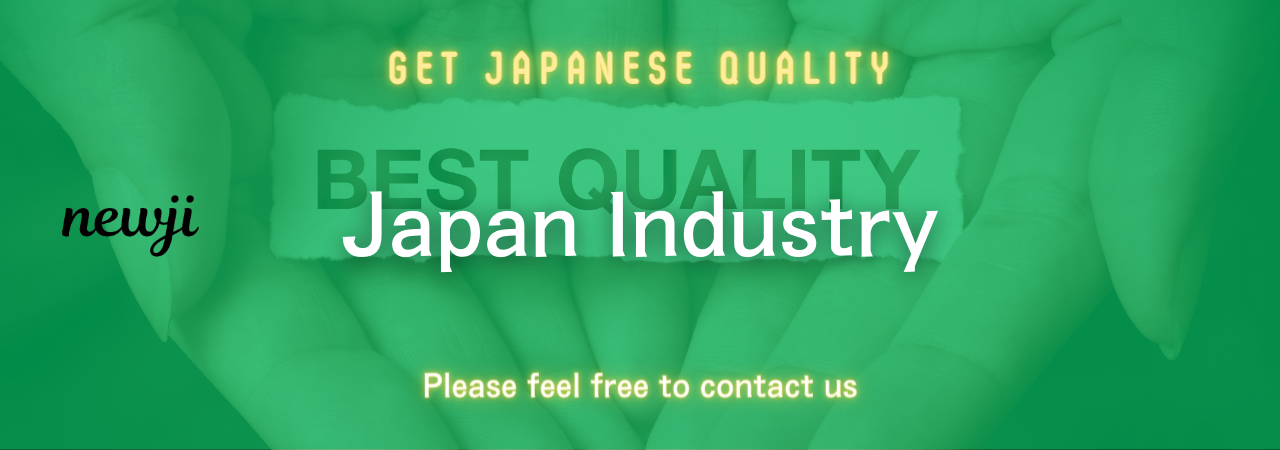
目次
Understanding Model Predictive Control (MPC)
Model Predictive Control (MPC) is an advanced control strategy that has gained popularity in industrial applications due to its ability to handle multivariable control problems effectively.
It is a type of control algorithm that makes decisions based on predictions of future behavior.
This is achieved by using a mathematical model of the process to predict the future outcomes of control actions.
MPC works by solving an optimization problem at each control step.
The optimization problem minimizes a cost function, usually comprising the difference between predicted and desired output, over a future time horizon.
The result is a sequence of control actions, but only the first action is implemented.
The process is then repeated at the next step, using new measurements and predictions.
Key Features of MPC
One of the main features of MPC is its ability to anticipate future events and take control actions accordingly.
This predictive nature allows it to handle time delays and process dynamics more effectively than traditional control methods.
Another feature is its ability to manage multiple input and output variables simultaneously.
This is particularly useful in complex systems where multiple factors influence the performance of a process.
MPC also incorporates constraints on input and output variables directly into the control law.
This capability is crucial for ensuring the safety and operability of industrial processes.
Benefits of Using MPC
MPC’s predictive ability leads to several benefits, especially in industries where process optimization is critical.
Some notable advantages include:
1. **Improved Efficiency**: By optimizing control actions over a future horizon, MPC can greatly enhance process efficiency, leading to reduced energy consumption and increased productivity.
2. **Better Constraint Handling**: The ability to include constraints in the control problem ensures processes operate within safe and legal limits, preventing equipment damage and safety breaches.
3. **Robust Performance**: MPC is inherently robust to disturbances and model uncertainties, providing stable control in fluctuating environments.
4. **Flexibility**: It can be tailored for a wide range of applications, from chemical processing to aerospace, making it a versatile tool for engineers.
Integrating MPC into Practical Applications
While the benefits of MPC are clear, its effective implementation requires careful consideration and understanding.
Here are key points for successful integration:
Model Accuracy
Accurate process models are essential for the effectiveness of MPC.
The model should capture the essential dynamics and constraints of the process.
Inaccurate models can lead to poor control performance and may jeopardize system stability.
Using data-driven modeling techniques, like system identification or machine learning, can enhance model accuracy.
Regular model updates and validation against real process data are also recommended to maintain precision over time.
Tuning and Optimization
MPC’s performance hinges on the proper tuning of its parameters, such as prediction horizon, control horizon, and weighting factors.
The prediction horizon should be long enough to capture essential future dynamics without overburdening computational resources.
The control horizon, typically shorter than the prediction horizon, should be set based on the speed of the system’s dynamics.
Weighting factors in the cost function should reflect the relative importance of control objectives and penalties on constraint violation.
These parameters often require iterative tuning and validation through simulations or trials to ensure optimal performance.
Computational Considerations
MPC involves solving a mathematical optimization problem at each control step, which can be computationally intensive.
Sufficient computational resources should be allocated to ensure real-time operation, especially in fast-moving processes.
In cases where resources are limited, various strategies can be employed, such as simplifying the process model, reducing the horizon lengths, or using approximate optimization algorithms.
Handling Non-linearity
Many real-world processes exhibit non-linear behaviors, which linear MPC might struggle to control effectively.
Non-linear MPC (NMPC) or linearizing the process around an operating point can be viable solutions.
NMPC involves using non-linear models in the predictive control framework, but requires more computational power.
Alternatively, gain-scheduling or piecewise linear models can approximate non-linear behaviors while simplifying computations.
Challenges and Considerations in Implementing MPC
Despite its advantages, MPC implementation can be challenging.
Complexity and Cost
The complexity of MPC design can be a barrier, especially for smaller companies with limited resources.
Designing, implementing, and maintaining an MPC system requires a significant investment in terms of time and expertise.
Training and Expertise
Specialized knowledge is needed to develop, tune, and maintain MPC systems effectively.
Training programs and hiring skilled personnel are essential, which might increase costs initially but are valuable in achieving long-term benefits.
Data Availability and Quality
High-quality data is crucial for model development and validation in MPC.
Poor data quality or limited data availability can affect the accuracy of the process model and degrade control performance.
Conclusion
Model Predictive Control (MPC) is a powerful tool in modern process control, offering advantages in efficiency, constraint management, and robustness.
To leverage its full potential, accurate modeling, proper tuning, and computational resources are necessary.
Despite challenges, such as complexity and the need for specialized expertise, the benefits of MPC in improving process performance and adapting to changing conditions make it a worthwhile investment.
By understanding these basics and applying them diligently, industries can harness MPC’s capabilities for effective and optimized control.
資料ダウンロード
QCD調達購買管理クラウド「newji」は、調達購買部門で必要なQCD管理全てを備えた、現場特化型兼クラウド型の今世紀最高の購買管理システムとなります。
ユーザー登録
調達購買業務の効率化だけでなく、システムを導入することで、コスト削減や製品・資材のステータス可視化のほか、属人化していた購買情報の共有化による内部不正防止や統制にも役立ちます。
NEWJI DX
製造業に特化したデジタルトランスフォーメーション(DX)の実現を目指す請負開発型のコンサルティングサービスです。AI、iPaaS、および先端の技術を駆使して、製造プロセスの効率化、業務効率化、チームワーク強化、コスト削減、品質向上を実現します。このサービスは、製造業の課題を深く理解し、それに対する最適なデジタルソリューションを提供することで、企業が持続的な成長とイノベーションを達成できるようサポートします。
オンライン講座
製造業、主に購買・調達部門にお勤めの方々に向けた情報を配信しております。
新任の方やベテランの方、管理職を対象とした幅広いコンテンツをご用意しております。
お問い合わせ
コストダウンが利益に直結する術だと理解していても、なかなか前に進めることができない状況。そんな時は、newjiのコストダウン自動化機能で大きく利益貢献しよう!
(Β版非公開)