- お役立ち記事
- Basics of multi-objective optimization design and practical application course
月間77,185名の
製造業ご担当者様が閲覧しています*
*2025年2月28日現在のGoogle Analyticsのデータより
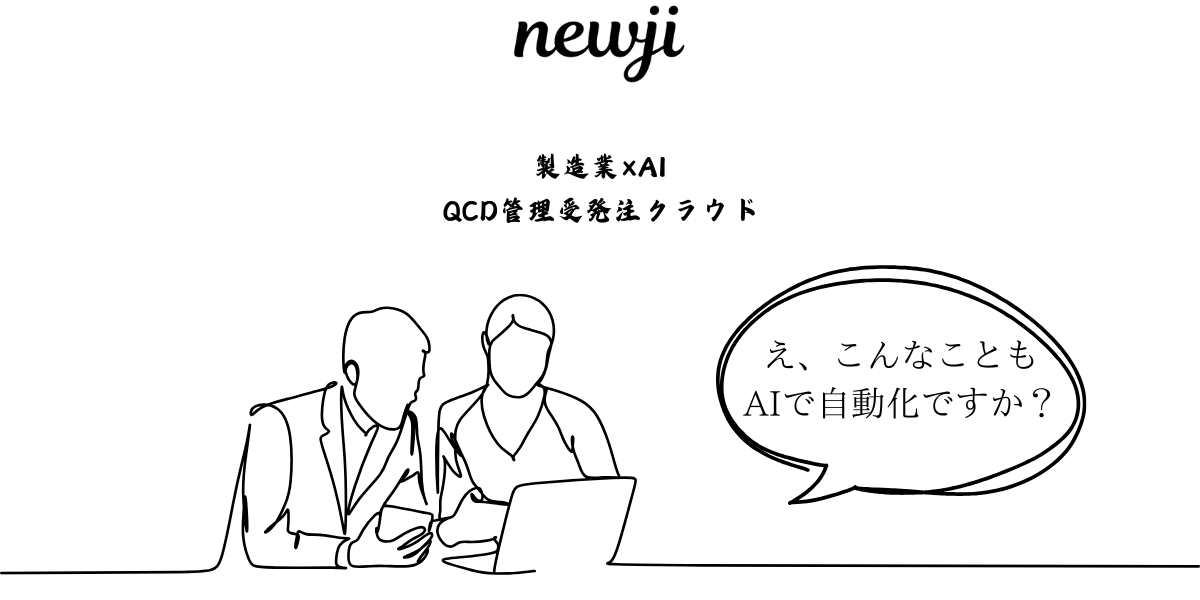
Basics of multi-objective optimization design and practical application course
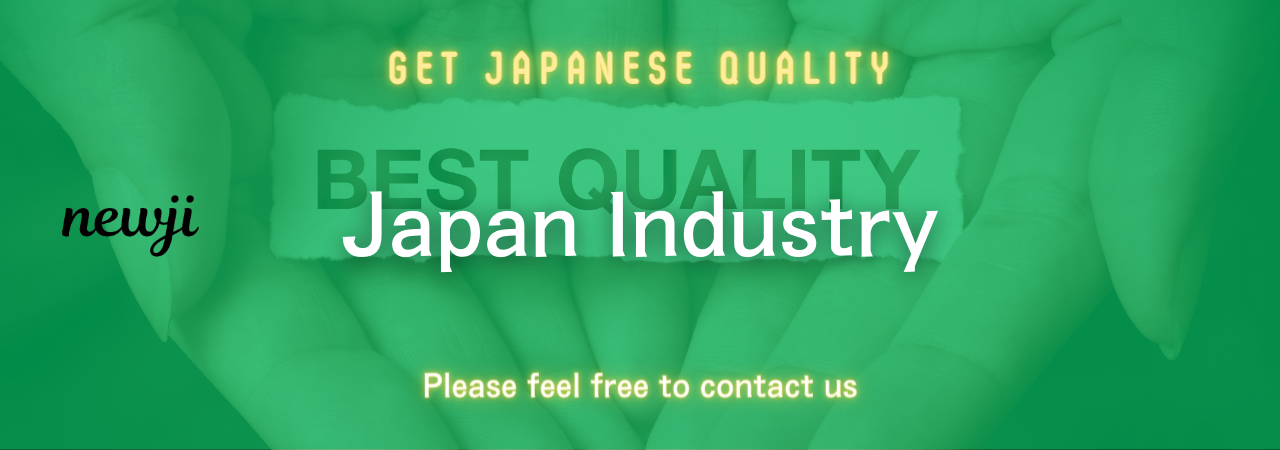
目次
Understanding Multi-Objective Optimization
Multi-objective optimization is a crucial concept in decision-making processes across various fields, ranging from engineering to economics.
Essentially, it involves optimizing two or more conflicting objectives simultaneously.
For example, in engineering design, one might need to balance cost, performance, and reliability, which often have competing demands.
Understanding the basics of multi-objective optimization can help practitioners make informed decisions that best satisfy all criteria.
At the heart of multi-objective optimization is the idea of trade-offs.
In most situations, improving one objective might lead to a decline in another.
For instance, enhancing the strength of a material might increase its cost.
Finding a suitable solution that offers an acceptable balance between these objectives is the primary goal.
Key Concepts in Multi-Objective Optimization
To navigate the complexities of multi-objective optimization, several key concepts are essential.
Pareto Optimality
One of the foundational concepts is Pareto Optimality.
A solution is considered Pareto Optimal if no other solution exists that can improve one objective without deteriorating another.
In simpler terms, it’s a state where making any one aspect better would make another worse.
The set of all Pareto Optimal solutions is known as the Pareto frontier or Pareto front.
Finding and analyzing this front is a significant task in multi-objective optimization.
Trade-Off Curves
Trade-off curves graphically represent the trade-offs involved between two objectives.
They allow decision-makers to visualize how one objective improves at the expense of the other.
These curves can be critical in understanding the degree of compromise needed and help in selecting solutions that align with overall goals and preferences.
Weighting Objectives
Another approach to managing multiple objectives is assigning weights to different objectives based on their importance.
This way, a composite objective function can be created, where each objective is multiplied by a corresponding weight and summed up.
The challenge here lies in choosing appropriate weights that reflect the relative importance of each objective accurately.
Methods for Solving Multi-Objective Optimization Problems
Several methods can tackle multi-objective optimization problems, each with its advantages and limitations.
Weighted Sum Method
The weighted sum method is one of the simplest approaches to solving multi-objective optimization problems.
In this method, each objective is given a weight, and the sum of these weighted objectives becomes the new single objective to optimize.
While simple, this method has drawbacks, such as potentially not identifying all Pareto Optimal solutions, especially when the Pareto front is non-convex.
Goal Programming
Goal programming involves setting specific target values for each objective.
The aim is to find solutions that achieve these target values as closely as possible.
This method is particularly useful when exact target values for objectives are known, allowing practitioners to focus on minimizing deviations from these targets.
Evolutionary Algorithms
Evolutionary algorithms, such as Genetic Algorithms, are popular for tackling complex multi-objective optimization problems.
These algorithms use mechanisms inspired by natural evolution, such as selection, mutation, and crossover, to explore potential solutions.
They are particularly effective in generating a diverse set of Pareto Optimal solutions, thus providing a comprehensive view of available trade-offs.
Practical Application of Multi-Objective Optimization
Applying multi-objective optimization concepts in real-world scenarios can significantly enhance decision-making processes.
Engineering Design
In engineering design, multi-objective optimization plays a critical role in developing products that meet various performance criteria.
For instance, when designing a vehicle, engineers must consider factors such as fuel efficiency, safety, and cost.
Balancing these objectives ensures a product that meets customer needs and regulatory standards effectively.
Environmental Management
Multi-objective optimization is equally valuable in environmental management, where trade-offs are frequently made between economic development and ecological preservation.
Decision-makers must evaluate strategies that fulfill economic goals while minimizing environmental impact.
Optimization tools can aid in developing sustainable practices that address both concerns.
Business Strategy
In business strategy, multi-objective optimization assists in creating plans that balance profitability, customer satisfaction, and social responsibility.
Companies often face conflicting objectives, like maximizing profits while maintaining high ethical standards.
Optimization can help businesses devise strategies that achieve a harmonious balance between these goals.
Challenges in Multi-Objective Optimization
Despite its benefits, multi-objective optimization also presents challenges.
Complexity and Computation
The complexity of calculating Pareto Optimal solutions increases with the number of objectives and constraints.
These computations can be resource-intensive and time-consuming, requiring sophisticated algorithms and high computational power.
Defining Objectives and Constraints
Clearly defining objectives and constraints can be difficult, especially when they aren’t easy to quantify.
Vague or poorly defined objectives can lead to suboptimal solutions, making it imperative to carefully analyze and articulate these elements from the onset.
Preference Articulation
Understanding and incorporating stakeholder preferences can be challenging, given their subjective nature.
Ensuring these preferences are accurately translated into the optimization process is vital for achieving the desired outcomes.
Conclusion
Multi-objective optimization is an invaluable tool for those navigating complex decision-making landscapes.
Whether in engineering, environmental management, or business, understanding how to balance competing objectives is crucial.
By leveraging techniques such as Pareto Optimality, evolutionary algorithms, and trade-off analysis, decision-makers can achieve solutions that address multiple goals effectively.
Though challenges exist, refining objectives and utilizing advanced computational methods can lead to optimal outcomes that satisfy various demands in real-world applications.
資料ダウンロード
QCD管理受発注クラウド「newji」は、受発注部門で必要なQCD管理全てを備えた、現場特化型兼クラウド型の今世紀最高の受発注管理システムとなります。
ユーザー登録
受発注業務の効率化だけでなく、システムを導入することで、コスト削減や製品・資材のステータス可視化のほか、属人化していた受発注情報の共有化による内部不正防止や統制にも役立ちます。
NEWJI DX
製造業に特化したデジタルトランスフォーメーション(DX)の実現を目指す請負開発型のコンサルティングサービスです。AI、iPaaS、および先端の技術を駆使して、製造プロセスの効率化、業務効率化、チームワーク強化、コスト削減、品質向上を実現します。このサービスは、製造業の課題を深く理解し、それに対する最適なデジタルソリューションを提供することで、企業が持続的な成長とイノベーションを達成できるようサポートします。
製造業ニュース解説
製造業、主に購買・調達部門にお勤めの方々に向けた情報を配信しております。
新任の方やベテランの方、管理職を対象とした幅広いコンテンツをご用意しております。
お問い合わせ
コストダウンが利益に直結する術だと理解していても、なかなか前に進めることができない状況。そんな時は、newjiのコストダウン自動化機能で大きく利益貢献しよう!
(β版非公開)