- お役立ち記事
- Basics of pattern recognition and effective data analysis methods and usage
Basics of pattern recognition and effective data analysis methods and usage
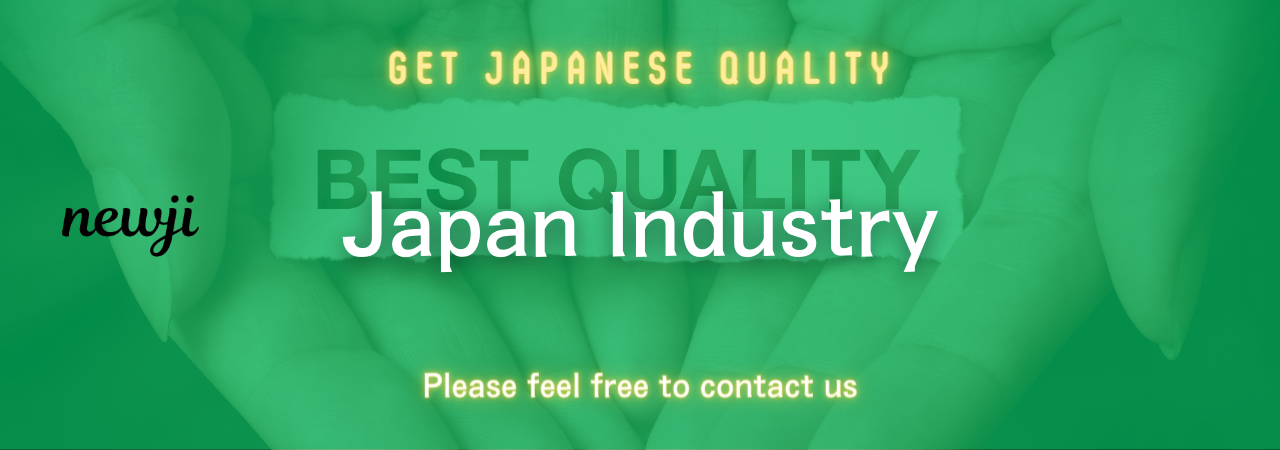
目次
Understanding Pattern Recognition
Pattern recognition is a crucial concept in the world of data analysis and artificial intelligence.
At its core, it involves identifying and categorizing patterns in data sets.
By recognizing these patterns, we can make predictions, uncover insights, and solve complex problems.
Pattern recognition allows machines to mimic human ability in recognizing regularities or irregularities in data.
There are two primary types of pattern recognition: supervised and unsupervised.
In supervised pattern recognition, the system is trained on a labeled dataset, helping it to learn the relationship between input data and the associated output labels.
Once training is complete, the system can recognize patterns in new, unseen data.
On the other hand, unsupervised pattern recognition does not involve labeled data.
The system identifies patterns or groups in the data without prior training.
Clustering and anomaly detection are common tasks in unsupervised pattern recognition.
The Importance of Data Analysis
Data analysis is the process of examining, cleaning, transforming, and modeling data to discover useful information and support decision-making.
It plays a pivotal role in various fields, including business, science, and healthcare.
Effective data analysis helps organizations and individuals understand trends, make informed decisions, and generate predictive insights.
By analyzing data, businesses can optimize their operations, improve customer experiences, and enhance overall efficiency.
Moreover, data analysis aids scientific research by identifying patterns and testing hypotheses, leading to groundbreaking discoveries.
In the medical field, data analysis can predict disease outbreaks, determine effective treatments, and improve patient care.
Effective Methods of Data Analysis
Several methods are pivotal in conducting effective data analysis.
Here are some of the most common techniques:
1. **Descriptive Analysis**: This method involves summarizing historical data to identify patterns.
It provides insights into what has happened over a specific time period, using metrics such as mean, median, mode, and standard deviation.
2. **Diagnostic Analysis**: This technique delves deeper into data to understand the reasons behind certain patterns or anomalies.
It involves comparing datasets to find correlations or trends that explain past outcomes.
3. **Predictive Analysis**: Using statistical models and machine learning algorithms, predictive analysis forecasts future outcomes based on historical data.
It is instrumental in risk assessment, sales forecasting, and customer behavior prediction.
4. **Prescriptive Analysis**: This advanced method suggests actions based on the outcomes of predictive models.
It not only predicts what might happen but also recommends possible courses of action to optimize results.
5. **Exploratory Data Analysis (EDA)**: EDA is a crucial step in data analysis, involving graphical representation and visual inspection of data.
It helps in identifying patterns, spotting anomalies, and testing assumptions.
Applications of Pattern Recognition and Data Analysis
In today’s data-driven world, pattern recognition and data analysis have numerous applications across various industries.
1. **Healthcare**: In the medical field, pattern recognition is used to diagnose diseases from medical images and lab results.
Data analysis predicts patient outcomes and identifies potential health risks.
2. **Finance**: Financial institutions use pattern recognition to detect fraudulent transactions and assess credit risk.
Data analysis helps in investment strategies and market predictions.
3. **Retail**: Businesses use data analysis to understand customer preferences and shopping behavior.
Pattern recognition in recommendation systems helps personalize customer experiences.
4. **Manufacturing**: Predictive maintenance relies on pattern recognition to forecast equipment failures.
Data analysis optimizes the supply chain by predicting demand and managing inventory levels.
5. **Social Media**: Platforms use pattern recognition to filter spam and enhance content recommendations.
Analyzing user engagement data helps improve ad targeting and social media strategies.
Challenges in Pattern Recognition and Data Analysis
Despite its benefits, pattern recognition and data analysis come with their own set of challenges.
– **Data Quality**: Inaccurate or incomplete data can lead to erroneous conclusions.
Ensuring data quality and integrity is crucial for reliable analysis.
– **Data Privacy**: With increasing data collection, maintaining privacy and complying with regulations like GDPR is a significant concern.
Organizations must balance the utility of data with the need to protect sensitive information.
– **Complexity and Computation Cost**: Analyzing large datasets requires significant computational resources and time.
Efficient algorithms and advanced hardware can mitigate these issues but may involve substantial investment.
– **Interpreting Results**: Extracting actionable insights from data is not always straightforward.
Analysts must have domain expertise and an understanding of statistical methods to interpret results accurately.
Future Trends in Pattern Recognition and Data Analysis
As technology evolves, so does the landscape of pattern recognition and data analysis.
– **Artificial Intelligence and Machine Learning**: AI and machine learning will continue to advance, leading to more sophisticated pattern recognition algorithms.
These technologies will enable faster and more accurate predictions.
– **Big Data**: With the advent of the Internet of Things (IoT) and digital platforms, the volume of data generated will grow exponentially.
Handling and analyzing big data will remain a top priority for organizations seeking a competitive advantage.
– **Real-Time Analytics**: The demand for real-time data analysis will increase, enabling businesses to respond swiftly to emerging trends and potential issues.
In conclusion, mastering pattern recognition and effective data analysis is essential in navigating the modern world.
These practices transform raw data into meaningful insights and drive strategic decisions, fueling innovation and progress across industries.
Understanding the fundamentals and overcoming challenges will empower individuals and businesses to harness the full potential of their data.
資料ダウンロード
QCD調達購買管理クラウド「newji」は、調達購買部門で必要なQCD管理全てを備えた、現場特化型兼クラウド型の今世紀最高の購買管理システムとなります。
ユーザー登録
調達購買業務の効率化だけでなく、システムを導入することで、コスト削減や製品・資材のステータス可視化のほか、属人化していた購買情報の共有化による内部不正防止や統制にも役立ちます。
NEWJI DX
製造業に特化したデジタルトランスフォーメーション(DX)の実現を目指す請負開発型のコンサルティングサービスです。AI、iPaaS、および先端の技術を駆使して、製造プロセスの効率化、業務効率化、チームワーク強化、コスト削減、品質向上を実現します。このサービスは、製造業の課題を深く理解し、それに対する最適なデジタルソリューションを提供することで、企業が持続的な成長とイノベーションを達成できるようサポートします。
オンライン講座
製造業、主に購買・調達部門にお勤めの方々に向けた情報を配信しております。
新任の方やベテランの方、管理職を対象とした幅広いコンテンツをご用意しております。
お問い合わせ
コストダウンが利益に直結する術だと理解していても、なかなか前に進めることができない状況。そんな時は、newjiのコストダウン自動化機能で大きく利益貢献しよう!
(Β版非公開)