- お役立ち記事
- Basics of reliability data analysis and effective use of Weibull analysis
月間76,176名の
製造業ご担当者様が閲覧しています*
*2025年3月31日現在のGoogle Analyticsのデータより
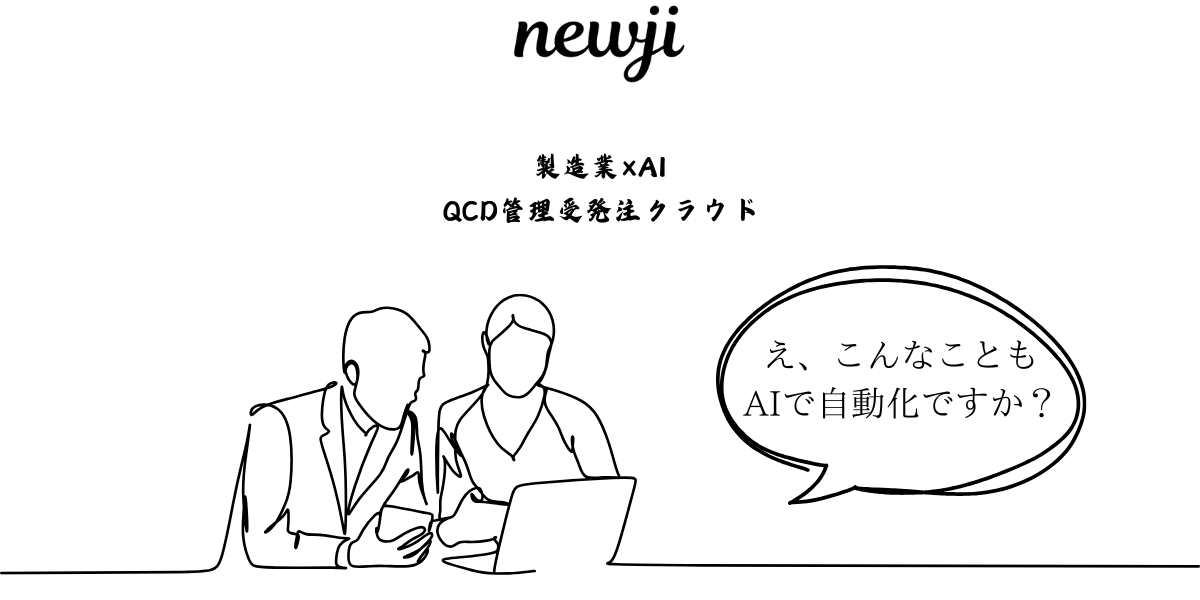
Basics of reliability data analysis and effective use of Weibull analysis
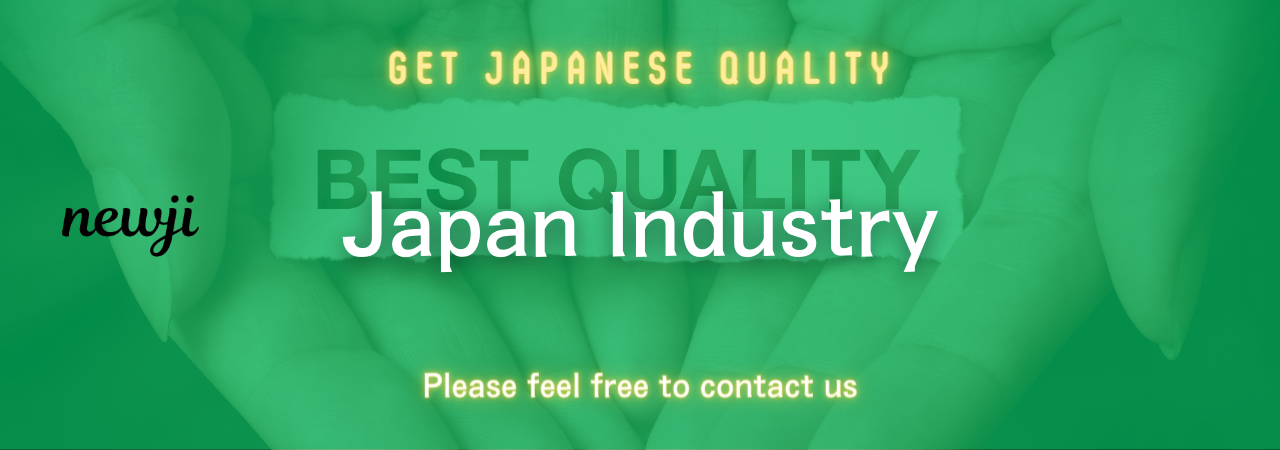
目次
Understanding Reliability Data Analysis
Reliability data analysis is a crucial aspect of product development and maintenance.
It helps organizations predict product performance over time and enhance quality while minimizing failures.
By analyzing reliability data, businesses can make informed decisions that improve product design, reduce costs, and ensure customer satisfaction.
Reliability data analysis involves collecting and interpreting data related to the lifespan and failure rates of products.
This information is vital for understanding how long a product will perform its intended function under specific conditions.
There are several key steps involved in reliability data analysis, each designed to provide insights into product behavior and performance over time.
Data Collection and Preparation
The first step in reliability data analysis is data collection.
This involves gathering information about the product or system, including its usage conditions, performance, and any failures that occur.
Data is typically collected through testing, field reports, customer feedback, and historical records.
Once collected, the data must be cleaned and prepared for analysis.
This involves checking for errors, inconsistencies, and missing values, and organizing the data in a way that facilitates analysis.
Proper data preparation ensures that the analysis results are accurate and reliable.
Data Analysis Techniques
There are several techniques used in reliability data analysis to interpret the collected data.
One of the most common methods is statistical analysis, which includes the use of probability distributions, regression analysis, and life data analysis.
These tools help identify trends, patterns, and relationships within the data.
Statistical process control (SPC) is another technique used to monitor and control processes, ensuring that they remain stable and within predefined limits.
SPC can help identify potential issues before they lead to product failures.
In addition to statistical methods, reliability engineers may use visual tools such as charts and graphs to represent data trends and relationships.
These visual tools help communicate analysis results more effectively, enabling better decision-making.
Introducing Weibull Analysis
Weibull analysis is a powerful tool used in reliability engineering to analyze life data and model failure times.
Named after Wallodi Weibull, the Weibull distribution is highly flexible and can model a variety of failure behaviors.
Why Use Weibull Analysis?
Weibull analysis provides several advantages over other methods of reliability analysis.
It can model a wide range of life data, from infant mortality (early failures) to wear-out (end-of-life failures).
This flexibility makes it ideal for analyzing complex systems and products with varying failure modes.
The Weibull distribution is also easy to understand and interpret, allowing engineers to communicate reliability insights to non-technical stakeholders effectively.
Components of Weibull Analysis
Weibull analysis involves several key components, including:
– Shape Parameter (β): This parameter indicates the failure rate behavior.
A β value less than 1 suggests decreasing failure rates (infant mortality), while a β greater than 1 indicates increasing failure rates (wear-out).
A β value close to 1 suggests a constant failure rate (random failures).
– Scale Parameter (η): This parameter, also known as the characteristic life, represents the time by which 63.2% of the population is expected to fail.
It helps estimate the product’s expected lifespan.
– Location Parameter (γ): Though less commonly used, this parameter shifts the distribution along the time axis, indicating a minimum lifespan before failures begin.
Steps in Performing Weibull Analysis
Performing Weibull analysis involves several steps:
1. **Data Collection**: Collect failure times and other relevant data to form the basis for analysis.
2. **Plotting Data**: Create a Weibull plot, a graphical representation of the data.
This plot helps visualize the failure trend and estimate the parameters of the Weibull distribution.
3. **Estimating Parameters**: Use statistical methods to estimate the shape, scale, and, if necessary, location parameters.
This step often involves fitting the Weibull distribution to the data using techniques such as maximum likelihood estimation (MLE) or least squares estimation (LSE).
4. **Interpreting Results**: Analyze the estimated parameters and the Weibull plot to gain insights into failure behavior.
Determine if the product exhibits infant mortality, random failures, or wear-out.
5. **Making Informed Decisions**: Use the insights gained from Weibull analysis to improve product design, optimize maintenance schedules, and enhance overall reliability.
Practical Applications of Reliability and Weibull Analysis
Reliability and Weibull analysis have practical applications across various industries, including electronics, automotive, aerospace, and manufacturing.
Predictive Maintenance
Organizations can use reliability analysis to implement predictive maintenance strategies.
By understanding when failures are likely to occur, maintenance can be scheduled proactively, reducing downtime and extending the equipment’s lifespan.
Product Development and Design
During product development, reliability data analysis provides valuable feedback on design performance.
By identifying potential weaknesses early, teams can make data-driven decisions to enhance product reliability and reduce warranty claims.
Quality Assurance
Reliability analysis plays a key role in quality assurance.
By analyzing failure data, quality control teams can pinpoint problems in manufacturing processes and implement corrective actions to enhance product quality.
Warranty and Risk Management
Understanding product reliability helps organizations manage warranty costs and risks.
Weibull analysis estimates warranty period failures and guides warranty extension decisions.
Conclusion
Reliability data analysis, including Weibull analysis, is essential for predicting and improving product performance.
By understanding product behavior over time, organizations can make better decisions, optimize maintenance, and improve design.
The insights gained through reliability analysis help reduce costs, enhance customer satisfaction, and ensure product success in a competitive market.
資料ダウンロード
QCD管理受発注クラウド「newji」は、受発注部門で必要なQCD管理全てを備えた、現場特化型兼クラウド型の今世紀最高の受発注管理システムとなります。
ユーザー登録
受発注業務の効率化だけでなく、システムを導入することで、コスト削減や製品・資材のステータス可視化のほか、属人化していた受発注情報の共有化による内部不正防止や統制にも役立ちます。
NEWJI DX
製造業に特化したデジタルトランスフォーメーション(DX)の実現を目指す請負開発型のコンサルティングサービスです。AI、iPaaS、および先端の技術を駆使して、製造プロセスの効率化、業務効率化、チームワーク強化、コスト削減、品質向上を実現します。このサービスは、製造業の課題を深く理解し、それに対する最適なデジタルソリューションを提供することで、企業が持続的な成長とイノベーションを達成できるようサポートします。
製造業ニュース解説
製造業、主に購買・調達部門にお勤めの方々に向けた情報を配信しております。
新任の方やベテランの方、管理職を対象とした幅広いコンテンツをご用意しております。
お問い合わせ
コストダウンが利益に直結する術だと理解していても、なかなか前に進めることができない状況。そんな時は、newjiのコストダウン自動化機能で大きく利益貢献しよう!
(β版非公開)