- お役立ち記事
- Basics of reliability data analysis, reliability analysis methods, and practical points for life prediction
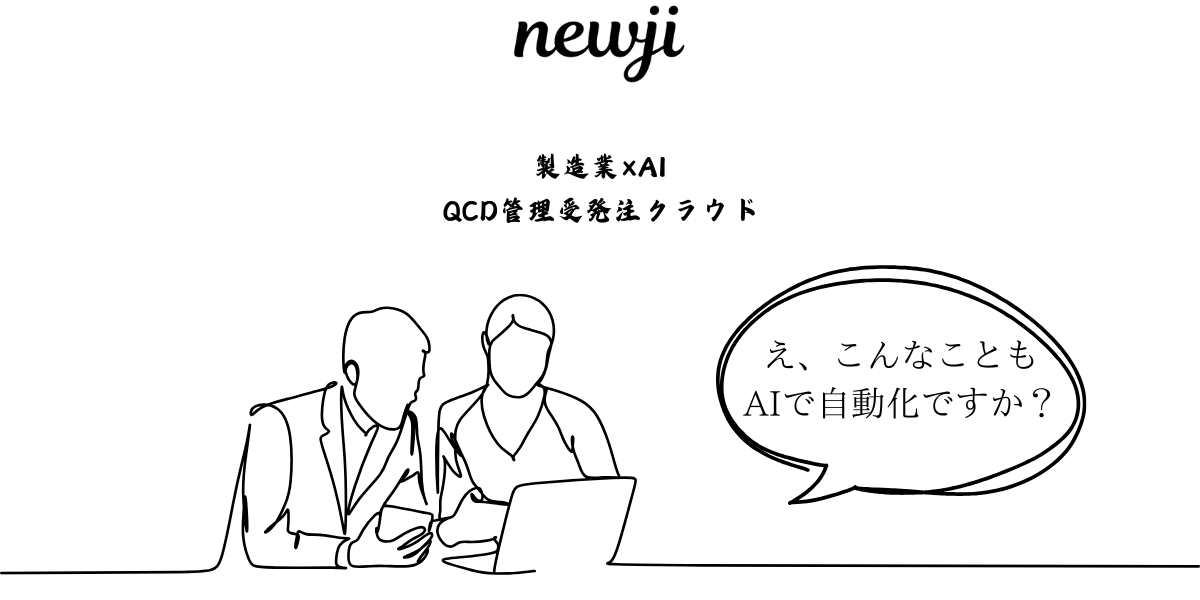
Basics of reliability data analysis, reliability analysis methods, and practical points for life prediction
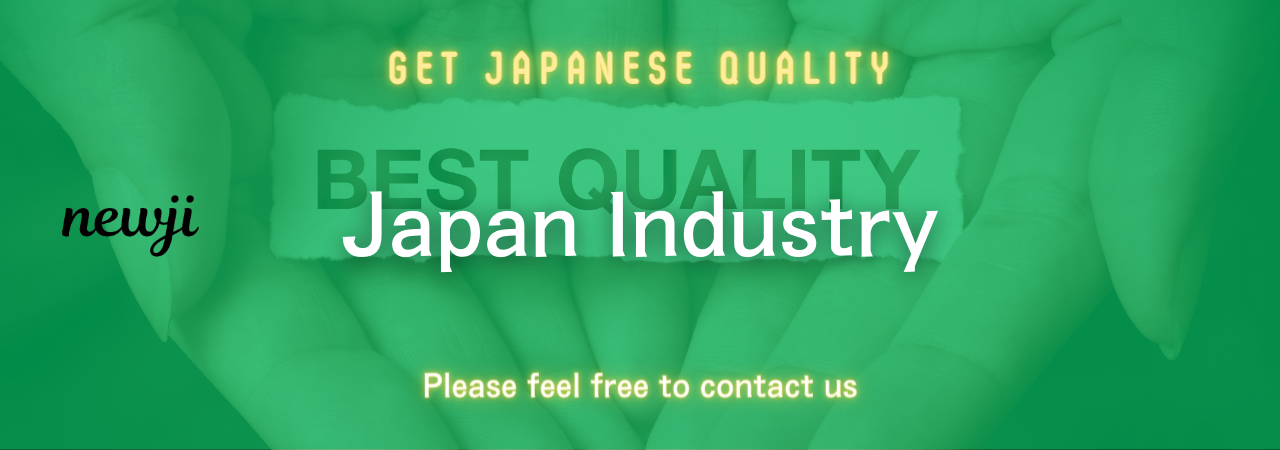
目次
Understanding Reliability Data Analysis
Reliability data analysis is a crucial part of ensuring that products and systems operate as expected over their intended lifespan.
It involves collecting, analyzing, and interpreting data related to the performance and durability of a product.
The aim is to predict how long a product will function under certain conditions and to identify areas for improvement.
Reliability data can come from various sources, including manufacturing processes, field data, and testing.
This data allows engineers and manufacturers to understand failure modes and mechanisms, helping them to design more reliable products.
By comprehensively understanding this data, companies can make informed decisions that enhance product quality and customer satisfaction.
Importance of Collecting Reliance Data
Collecting reliability data is essential for a few significant reasons.
Firstly, it provides insights into how products perform in real-world scenarios.
This information helps manufacturers identify potential weaknesses in their designs or materials used.
Secondly, it assists in developing maintenance schedules.
By understanding when and how products are likely to fail, companies can create effective maintenance plans that minimize downtime and extend the lifespan of their products.
Moreover, reliability data analysis is valuable for risk assessment.
By predicting failure rates, businesses can assess the potential impact of a failure and implement measures to mitigate these risks.
This process not only protects the end-user but also influences the company’s reputation positively.
Methods of Reliability Analysis
Reliability analysis employs several methods to determine how long a product will last and how frequently it will fail.
These methods help in making accurate predictions and setting priorities for product improvement.
Life Data Analysis
Life data analysis, also known as Weibull analysis, is a widely used method for reliability analysis.
It involves analyzing time-to-failure data to model the life characteristics of a product.
The Weibull distribution is commonly used in this method due to its flexibility in modeling various types of failure rates.
In this approach, data is collected until a certain number of failures occur, or testing is stopped after a predetermined time.
From this, parameters like reliability function, hazard rate, and mean time to failure can be extracted.
Such analysis is indispensable for predicting a product’s life expectancy and making data-driven decisions.
Accelerated Life Testing (ALT)
Accelerated Life Testing (ALT) is another common technique.
It involves subjecting a product to higher stress levels than what they would typically experience in normal operation.
The idea is to expedite the failure process and gather data in a shorter period.
This method is particularly useful for products with long life spans, where waiting for failures under normal conditions is impractical.
Predictive models from ALT can significantly reduce testing times and bring products to market more quickly, benefiting both manufacturers and consumers.
Reliability Block Diagrams (RBD)
Reliability Block Diagrams (RBD) provide a visual representation of a system’s reliability structure.
They help in understanding how different components contribute to the overall system reliability.
This method is especially useful for complex systems with many interdependent parts.
By identifying critical components that affect system performance, engineers can focus their efforts on improving these areas.
RBDs also facilitate scenario analysis, where various configurations and improvements can be evaluated to enhance system reliability.
Practical Points for Life Prediction
Accurate life prediction is critical for manufacturers to deliver dependable products.
Several practical points should be considered to ensure effective life prediction through reliability analysis.
Comprehensive Data Collection
Thorough data collection is the backbone of any reliability analysis.
Capturing all relevant data, such as environmental conditions, usage patterns, and failure modes, is vital.
This comprehensive data set provides a robust basis for analysis, leading to more accurate predictions.
Additionally, data should be gathered from different sources and stages of the product lifecycle.
This variety ensures that all potential reliability issues are considered during the analysis.
Choosing the Right Analysis Method
Selecting the appropriate analysis method is essential for reliable life prediction.
Different products and systems require different approaches based on their complexity, usage conditions, and expected life span.
For example, simple consumer electronics might benefit from life data analysis using the Weibull distribution.
In contrast, more complex systems, such as automotive components, might require a combination of methods, including RBD and ALT, to achieve accurate predictions.
Continuous Improvement and Iteration
Life prediction is not a one-time activity.
It’s an iterative process that benefits greatly from continuous improvement.
As new data becomes available or when products undergo design changes, the analysis should be updated to reflect these changes.
Feedback mechanisms should be in place to assess the accuracy of previous predictions.
This feedback helps refine future analyses and improve the overall reliability of the product.
Conclusion
Reliability data analysis is a fundamental aspect of product development and lifecycle management.
By understanding and applying various reliability analysis methods, manufacturers can make informed decisions to enhance product reliability.
Collecting comprehensive data, selecting suitable analysis methods, and continuously improving predictions are crucial steps in delivering products that meet customer expectations and withstand the test of time.
Ultimately, such efforts lead to higher customer satisfaction and a stronger market reputation.
資料ダウンロード
QCD調達購買管理クラウド「newji」は、調達購買部門で必要なQCD管理全てを備えた、現場特化型兼クラウド型の今世紀最高の購買管理システムとなります。
ユーザー登録
調達購買業務の効率化だけでなく、システムを導入することで、コスト削減や製品・資材のステータス可視化のほか、属人化していた購買情報の共有化による内部不正防止や統制にも役立ちます。
NEWJI DX
製造業に特化したデジタルトランスフォーメーション(DX)の実現を目指す請負開発型のコンサルティングサービスです。AI、iPaaS、および先端の技術を駆使して、製造プロセスの効率化、業務効率化、チームワーク強化、コスト削減、品質向上を実現します。このサービスは、製造業の課題を深く理解し、それに対する最適なデジタルソリューションを提供することで、企業が持続的な成長とイノベーションを達成できるようサポートします。
オンライン講座
製造業、主に購買・調達部門にお勤めの方々に向けた情報を配信しております。
新任の方やベテランの方、管理職を対象とした幅広いコンテンツをご用意しております。
お問い合わせ
コストダウンが利益に直結する術だと理解していても、なかなか前に進めることができない状況。そんな時は、newjiのコストダウン自動化機能で大きく利益貢献しよう!
(Β版非公開)