- お役立ち記事
- Basics of self-position estimation technology and target route tracking technology in autonomous driving and application to driving support systems
Basics of self-position estimation technology and target route tracking technology in autonomous driving and application to driving support systems
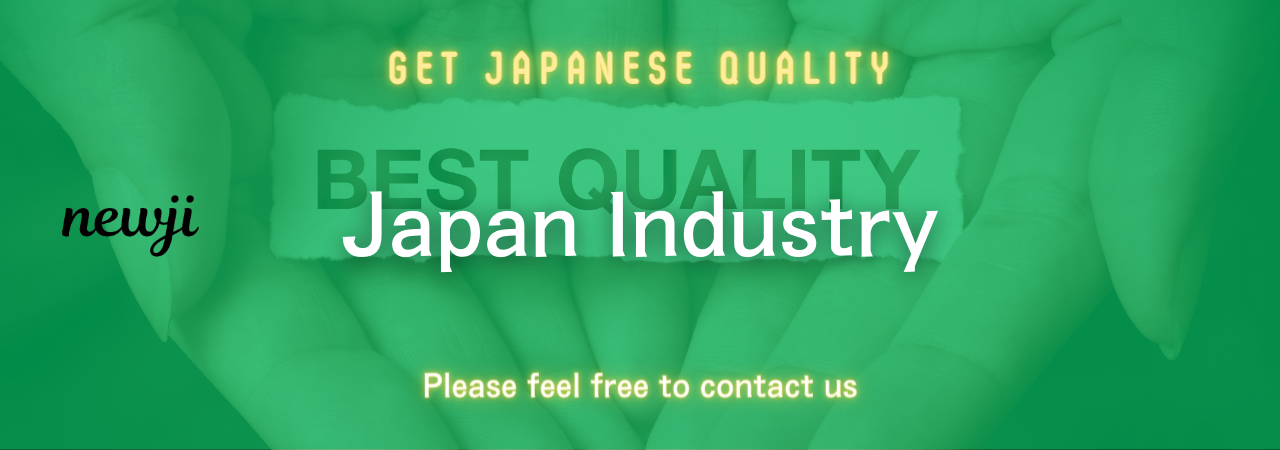
目次
Introduction to Self-Position Estimation in Autonomous Vehicles
Self-position estimation is a fundamental technology used in autonomous driving.
This technology enables vehicles to determine their exact location on the road.
It is essential for navigation and ensuring safety.
By using self-position estimation, autonomous vehicles can effectively track their routes and make informed driving decisions.
Understanding the precise location helps these vehicles avoid obstacles and adhere to traffic regulations.
Various sensors and algorithms work together to achieve accurate self-position estimation.
GPS, LiDAR, cameras, and inertial measurement units (IMUs) are integral components of this process.
The Role of GPS in Self-Position Estimation
Global Positioning System (GPS) technology plays a pivotal role in providing location data.
GPS satellites orbiting the Earth transmit signals to receivers in autonomous vehicles.
These receivers calculate the vehicle’s position based on the time it takes for the signals to reach them.
GPS provides a general location but can be limited by its accuracy, typically several meters off.
To enhance this, autonomous vehicles employ additional technologies.
Differential GPS (DGPS) and Real-Time Kinematic (RTK) positioning are methods that improve GPS accuracy.
These systems provide corrections to standard GPS signals, reducing errors and providing centimeter-level accuracy.
LiDAR and Its Importance in Positioning
Light Detection and Ranging (LiDAR) uses lasers to measure distances to surrounding objects and structures.
This technology generates a detailed 3D map of the vehicle’s environment.
By comparing this map with pre-stored high-definition maps, an autonomous vehicle can pinpoint its position with high precision.
LiDAR’s ability to function in various weather and lighting conditions makes it a reliable component of self-position estimation.
However, LiDAR can be expensive and consume significant power, which are considerations for its widespread use.
Camera Systems and Visual Odometry
Cameras are another crucial component in self-position estimation.
They capture images of the vehicle’s surroundings, which are processed to understand the environment.
Visual odometry is a technique used to estimate the vehicle’s movement by analyzing these images.
By tracking changes between consecutive frames, the vehicle can determine its movement and position.
Cameras are relatively cost-effective and provide rich data, but they can struggle in poor lighting or adverse weather conditions.
Inertial Measurement Units (IMUs) and Sensor Fusion
Inertial Measurement Units (IMUs) are devices that measure acceleration and rotational movements.
They provide data on the vehicle’s orientation and are especially useful when GPS signals are weak or unavailable, such as in tunnels or urban canyons.
Sensor fusion is the process of integrating data from multiple sources, including GPS, LiDAR, cameras, and IMUs.
By combining these data streams, autonomous vehicles achieve a more accurate and reliable estimate of their position.
This redundancy ensures that even if one system fails, others can compensate, maintaining the vehicle’s situational awareness.
Target Route Tracking in Autonomous Vehicles
Target route tracking is another essential aspect of autonomous driving.
This capability enables vehicles to follow a predetermined path or reach a specific destination.
Route tracking involves continuous monitoring of the vehicle’s position and making adjustments to stay on course.
Path Planning and Control
Path planning is the process of determining the most efficient route from one point to another.
This involves considering various factors such as traffic, road conditions, and obstacles.
Once a path is established, the vehicle uses control algorithms to follow it accurately.
Control algorithms ensure the vehicle maintains its lane, adjusts speed, and navigates turns.
These algorithms are informed by real-time data from onboard sensors and external traffic information.
Adaptive control systems can respond to changes in the environment, such as sudden stops or detours.
Lane Keeping and Obstacle Avoidance
Lane keeping ensures that the autonomous vehicle remains in the correct lane while driving.
Cameras, LiDAR, and radar systems provide data on lane markings and nearby vehicles.
With this information, the vehicle can make precise adjustments to its steering to maintain lane discipline.
Obstacle avoidance is another critical capability.
Autonomous vehicles must detect and navigate around obstacles such as pedestrians, other vehicles, and road debris.
This involves a combination of sensor data interpretation and rapid decision-making to execute safe maneuvers.
Application in Driving Support Systems
The technologies used for self-position estimation and route tracking are essential for autonomous vehicles.
They also have significant applications in driving support systems for traditional vehicles.
Advanced Driver-Assistance Systems (ADAS)
Advanced Driver-Assistance Systems (ADAS) use similar technologies to enhance the safety and convenience of human-driven vehicles.
These systems provide features like adaptive cruise control, lane departure warning, and automatic emergency braking.
By incorporating self-position estimation and route tracking technologies, ADAS improves vehicle safety.
Drivers receive real-time feedback and alerts, helping them make informed decisions on the road.
Navigation and Traffic Management
Accurate positioning and route tracking technologies enhance navigation systems, offering precise directions and updated traffic information.
Drivers can rely on these systems for efficient route planning and avoiding congestion.
In traffic management, these technologies help optimize flow and reduce bottlenecks.
Smart traffic systems use data from connected vehicles to adjust signal timings and manage road usage dynamically.
Conclusion
Self-position estimation and target route tracking are vital technologies in the realm of autonomous driving.
They enable vehicles to navigate accurately and safely, providing the foundation for advanced autonomous and assisted driving systems.
As these technologies continue to evolve, they will spur further innovations, enhancing both autonomous capabilities and driving support systems for all vehicles on the road.
資料ダウンロード
QCD調達購買管理クラウド「newji」は、調達購買部門で必要なQCD管理全てを備えた、現場特化型兼クラウド型の今世紀最高の購買管理システムとなります。
ユーザー登録
調達購買業務の効率化だけでなく、システムを導入することで、コスト削減や製品・資材のステータス可視化のほか、属人化していた購買情報の共有化による内部不正防止や統制にも役立ちます。
NEWJI DX
製造業に特化したデジタルトランスフォーメーション(DX)の実現を目指す請負開発型のコンサルティングサービスです。AI、iPaaS、および先端の技術を駆使して、製造プロセスの効率化、業務効率化、チームワーク強化、コスト削減、品質向上を実現します。このサービスは、製造業の課題を深く理解し、それに対する最適なデジタルソリューションを提供することで、企業が持続的な成長とイノベーションを達成できるようサポートします。
オンライン講座
製造業、主に購買・調達部門にお勤めの方々に向けた情報を配信しております。
新任の方やベテランの方、管理職を対象とした幅広いコンテンツをご用意しております。
お問い合わせ
コストダウンが利益に直結する術だと理解していても、なかなか前に進めることができない状況。そんな時は、newjiのコストダウン自動化機能で大きく利益貢献しよう!
(Β版非公開)