- お役立ち記事
- Basics of sensing data analysis and anomaly detection technology
Basics of sensing data analysis and anomaly detection technology
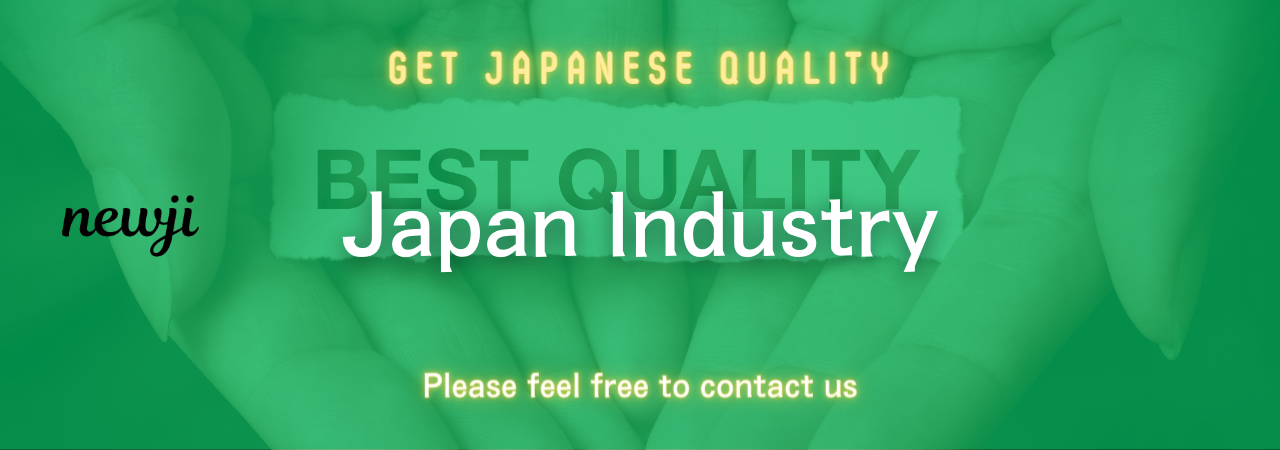
目次
Understanding the Basics of Sensing Data Analysis
Sensing data analysis is a fascinating area of technology that continues to evolve and transform the way we interact with the world.
At its core, sensing data analysis involves collecting data from various sensors to understand and interpret what is happening in an environment.
This data can come from a multitude of sources, like temperature sensors, motion detectors, and even complex biosensors.
The purpose of this analysis is to glean actionable insights that can help in monitoring, control, and decision-making processes.
Whether you’re an engineer or simply curious about technology, understanding the basics of sensing data analysis is essential.
Sensing data can be vast and complex.
This data can come from small-scale sensors monitoring an individual’s health to large-scale sensors tracking climate changes.
In processing this data, analysts aim to identify patterns, trends, and correlations that can be used to predict future outcomes.
This makes sensing data analysis incredibly valuable across various industries, including healthcare, manufacturing, and agriculture.
How Sensing Data is Collected
The process begins with data collection.
Sensors are often deployed in environments to capture specific types of data.
For instance, a temperature sensor in a warehouse might track climate conditions to ensure goods are stored properly.
Similarly, motion detectors in a security system can pinpoint movements, thus alerting security personnel of potential intruders.
The data collected by these sensors can be structured or unstructured.
Structured data follows a consistent format, making it easier to process.
Conversely, unstructured data might require more sophisticated tools for handling and analysis.
Examples of structured data include spreadsheets and databases, while unstructured data could be images or audio files.
The Role of Data Analysis Tools
After data collection, analysis is the next step.
Typically, this involves using analytical tools and software to transform raw data into meaningful insights.
These tools help extract valuable information by identifying patterns and anomalies within the collected data.
There are a variety of techniques employed depending on the complexity of the data.
For example, machine learning algorithms are commonly used to analyze large datasets as they can quickly identify intricate patterns that might be invisible to the naked eye.
Additionally, visualization tools like graphs and charts can help make data easier to understand and interpret.
Anomaly Detection in Sensing Data
Anomaly detection is a critical element of sensing data analysis.
Essentially, this process involves identifying data points, patterns, or events that do not conform to a ‘normal’ behavior or pattern.
Anomalies can indicate significant but rare events, such as a fault in a system or a security breach.
In many cases, anomalies need immediate attention.
For example, a significant temperature drop detected by a sensor in a greenhouse might suggest a malfunctioning heater.
Identifying such anomalies promptly ensures that corrective actions can be taken quickly to prevent further issues.
Types of Anomalies
Anomalies can generally be classified into three main types.
First, we have point anomalies, where a single data instance is significantly different from others.
For instance, a temperature sensor recording an extreme temperature that deviates drastically from normal readings.
Second, contextual anomalies occur when a data point is normal in one context but anomalous in another.
For example, an increase in e-commerce sales might be expected during the holiday season, but an unusual surge at an off-peak time could be suspect.
Lastly, collective anomalies occur when a group of related data points deviate from the norm.
These are common in network traffic analysis, where an unusual spike in data packets might suggest a distributed denial of service (DDoS) attack.
Methods of Anomaly Detection
Detection of anomalies requires deploying various methodologies, with each suited to the specific nature of the data and the industry.
Statistical methods are traditional techniques that involve defining thresholds and using classical hypothesis tests to locate anomalies.
Machine learning methods are increasingly popular, utilizing algorithms trained to recognize normal patterns and detect deviations from these learned patterns.
These models can adapt and improve over time, becoming more accurate at spotting anomalies.
Moreover, hybrid methods that combine statistical approaches with machine learning can offer more robust solutions, leveraging the strengths of both to efficiently identify anomalies.
Applications of Sensing Data Analysis and Anomaly Detection
The practical applications of sensing data analysis and anomaly detection are vast.
In healthcare, biosensors can monitor patient vital signs in real time, alerting to any deviations that could signal health issues.
In manufacturing, sensors can detect equipment malfunctions, thereby preventing costly downtime by enabling quick repairs.
In agriculture, sensors monitor soil moisture and weather, optimizing water usage and increasing crop yield.
Meanwhile, in cybersecurity, anomaly detection algorithms help in identifying suspicious activities and protecting against potential threats.
The Future of Sensing Data Analysis
As technology progresses, the potential for sensing data analysis and anomaly detection will grow exponentially.
With advances in sensor technology and data analysis tools, we can expect more precise and efficient systems in the future.
The integration of AI and IoT (Internet of Things) technologies will further enhance the accuracy and breadth of sensing data analysis.
This will allow businesses to make smarter decisions and optimize their operations like never before, ultimately leading to more innovative and secure environments.
In conclusion, understanding the basics of sensing data analysis and anomaly detection is becoming more crucial as we move further into a data-driven world.
Equipped with this knowledge, you can appreciate how these technologies contribute to advancements across various fields and continue to shape our future.
資料ダウンロード
QCD調達購買管理クラウド「newji」は、調達購買部門で必要なQCD管理全てを備えた、現場特化型兼クラウド型の今世紀最高の購買管理システムとなります。
ユーザー登録
調達購買業務の効率化だけでなく、システムを導入することで、コスト削減や製品・資材のステータス可視化のほか、属人化していた購買情報の共有化による内部不正防止や統制にも役立ちます。
NEWJI DX
製造業に特化したデジタルトランスフォーメーション(DX)の実現を目指す請負開発型のコンサルティングサービスです。AI、iPaaS、および先端の技術を駆使して、製造プロセスの効率化、業務効率化、チームワーク強化、コスト削減、品質向上を実現します。このサービスは、製造業の課題を深く理解し、それに対する最適なデジタルソリューションを提供することで、企業が持続的な成長とイノベーションを達成できるようサポートします。
オンライン講座
製造業、主に購買・調達部門にお勤めの方々に向けた情報を配信しております。
新任の方やベテランの方、管理職を対象とした幅広いコンテンツをご用意しております。
お問い合わせ
コストダウンが利益に直結する術だと理解していても、なかなか前に進めることができない状況。そんな時は、newjiのコストダウン自動化機能で大きく利益貢献しよう!
(Β版非公開)