- お役立ち記事
- Basics of statistical acoustic signal processing technology and applied technology using deep learning
月間77,185名の
製造業ご担当者様が閲覧しています*
*2025年2月28日現在のGoogle Analyticsのデータより
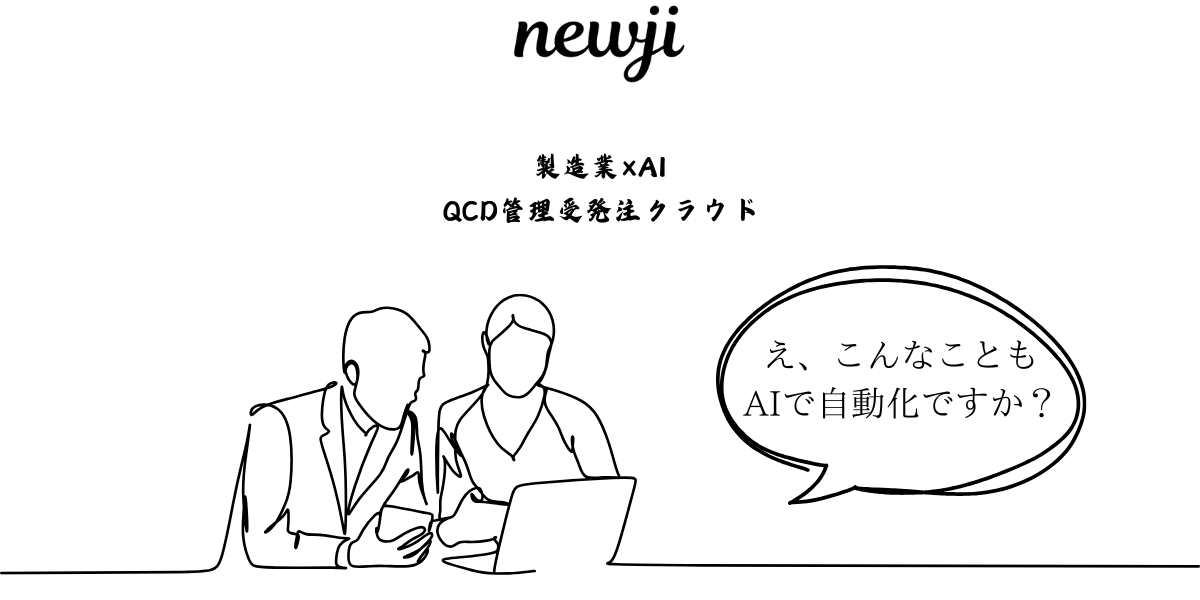
Basics of statistical acoustic signal processing technology and applied technology using deep learning
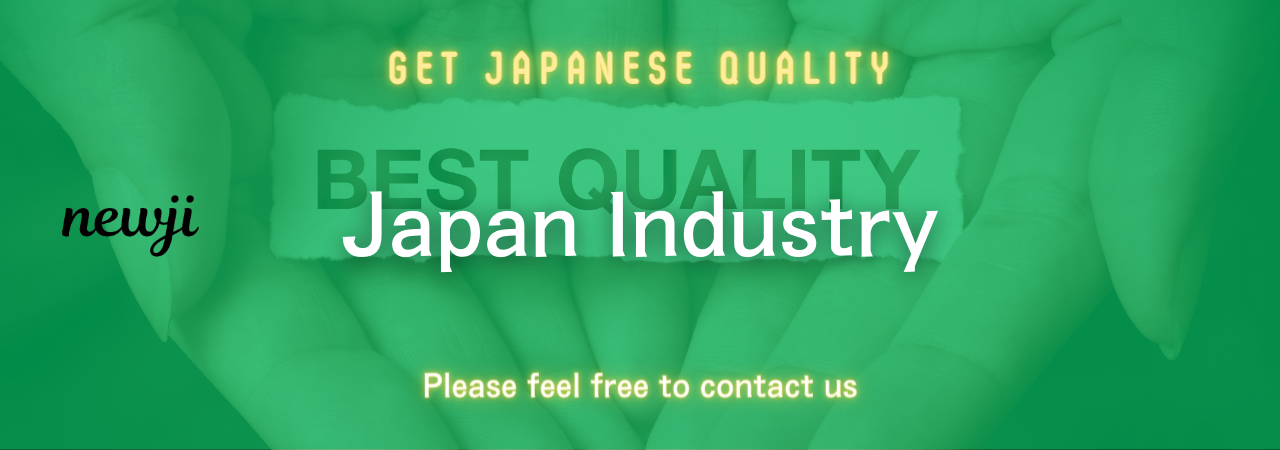
Statistical acoustic signal processing technology is an area of study that plays a vital role in understanding and manipulating sound data.
This technology is used in various applications such as noise reduction, speech recognition, and audio enhancement.
With the rise of deep learning, these applications have become even more sophisticated, allowing for improvements in accuracy and efficiency.
In this article, we will explore the basics of statistical acoustic signal processing technology and how it is enhanced by deep learning techniques.
We will also look into some of the practical applications that benefit from these technologies.
目次
Understanding Statistical Acoustic Signal Processing
Statistical acoustic signal processing is a field that involves the analysis and manipulation of sound waves using statistical methods.
The goal is to extract meaningful information from audio signals, which can then be used for various purposes.
This technology is crucial for reducing noise, enhancing audio quality, and enabling speech recognition systems to function more effectively.
The process usually starts with capturing audio signals, which can be represented as a series of data points over time.
Statistical methods are then applied to these data sets to achieve tasks such as noise reduction, sound source localization, and audio separation.
The Importance of Statistical Methods
Statistical methods are essential in acoustic signal processing because they provide tools to model and analyze sound data accurately.
They allow for the detection of patterns and anomalies in audio signals, which is important for tasks such as distinguishing speech from background noise.
For instance, models such as the Gaussian mixture model (GMM) are used to represent complex sound environments.
These models help in breaking down audio data into manageable parts, making it easier to process and interpret.
Deep Learning in Acoustic Signal Processing
Deep learning has made a significant impact on the field of acoustic signal processing by introducing advanced algorithms that can learn from vast amounts of data.
Neural networks, particularly convolutional neural networks (CNNs) and recurrent neural networks (RNNs), have been pivotal in improving the accuracy and efficiency of acoustic signal processing applications.
Advantages of Deep Learning
Deep learning methods provide several advantages over traditional statistical methods in acoustic signal processing.
They can automatically learn features from raw data, eliminating the need for manual feature extraction.
Deep learning models are also highly scalable, which means they can be applied to large datasets and continue to improve their performance as more data becomes available.
This scalability is particularly beneficial in applications where large volumes of audio data are processed, such as in virtual assistants and automated transcription services.
Integration with Acoustic Signal Processing
One of the key benefits of integrating deep learning with statistical acoustic signal processing is the ability to create more robust and precise models.
Deep learning algorithms can handle the variability and complexity of audio signals better than traditional methods.
For example, in speech recognition, deep learning models can recognize words and phrases in different accents and speaking styles more accurately than classical models.
This capability is due to their ability to learn from diverse datasets that encompass a wide range of audio scenarios.
Applications of Acoustic Signal Processing and Deep Learning
The combination of statistical acoustic signal processing and deep learning has paved the way for numerous applications across various fields.
Voice Assistants and Speech Recognition
Voice assistants like Siri, Alexa, and Google Assistant rely heavily on these technologies to understand and respond to user commands.
Deep learning models enhance the ability of these assistants to recognize speech patterns, even in noisy environments, making them more reliable and efficient.
Noise Reduction in Audio Devices
In the realm of consumer electronics, noise-canceling headphones and audio enhancement software utilize these technologies to provide clear and high-quality audio experiences.
Deep learning algorithms help in distinguishing unwanted noise from useful signals, effectively reducing or eliminating background noise.
Healthcare Applications
Acoustic signal processing is also critical in healthcare, particularly in developing hearing aids that adapt to various sound environments.
Deep learning enhances these devices by enabling more accurate sound classification and personalized audio tuning.
Security Systems
Security applications use acoustic signal processing to identify specific audio traits, such as gunshots, alarms, and breaking glass.
With deep learning, these systems can learn and recognize different sound profiles, leading to quicker detection and response times.
Future Trends and Challenges
While the advancements in statistical acoustic signal processing and deep learning are impressive, several challenges remain.
These include the need for large labeled datasets to train deep learning models, the computational power required to process these datasets, and ensuring privacy in audio data handling.
Overcoming Challenges
Researchers are actively working on developing new algorithms and approaches to mitigate these challenges.
Techniques such as synthetic data generation and transfer learning are being explored to reduce the dependency on large labeled datasets.
Furthermore, the use of cloud-based processing and improvements in hardware capabilities are helping to manage the computational demands.
Future Developments
Looking into the future, we can expect further integration of deep learning with acoustic signal processing, leading to even more powerful applications.
Innovations such as real-time sound recognition and adaptive audio environments promise to enhance user experiences across different domains.
As technology continues to evolve, the potential for creating smarter and more sensitive audio systems remains vast.
In conclusion, statistical acoustic signal processing technology and deep learning offer exciting opportunities to transform how we interact with sound data.
Their applications are already making significant impacts in everyday life, and future advancements are sure to bring even more groundbreaking capabilities.
資料ダウンロード
QCD管理受発注クラウド「newji」は、受発注部門で必要なQCD管理全てを備えた、現場特化型兼クラウド型の今世紀最高の受発注管理システムとなります。
ユーザー登録
受発注業務の効率化だけでなく、システムを導入することで、コスト削減や製品・資材のステータス可視化のほか、属人化していた受発注情報の共有化による内部不正防止や統制にも役立ちます。
NEWJI DX
製造業に特化したデジタルトランスフォーメーション(DX)の実現を目指す請負開発型のコンサルティングサービスです。AI、iPaaS、および先端の技術を駆使して、製造プロセスの効率化、業務効率化、チームワーク強化、コスト削減、品質向上を実現します。このサービスは、製造業の課題を深く理解し、それに対する最適なデジタルソリューションを提供することで、企業が持続的な成長とイノベーションを達成できるようサポートします。
製造業ニュース解説
製造業、主に購買・調達部門にお勤めの方々に向けた情報を配信しております。
新任の方やベテランの方、管理職を対象とした幅広いコンテンツをご用意しております。
お問い合わせ
コストダウンが利益に直結する術だと理解していても、なかなか前に進めることができない状況。そんな時は、newjiのコストダウン自動化機能で大きく利益貢献しよう!
(β版非公開)