- お役立ち記事
- Bayesian decision theory and predictive distributions
月間76,176名の
製造業ご担当者様が閲覧しています*
*2025年3月31日現在のGoogle Analyticsのデータより
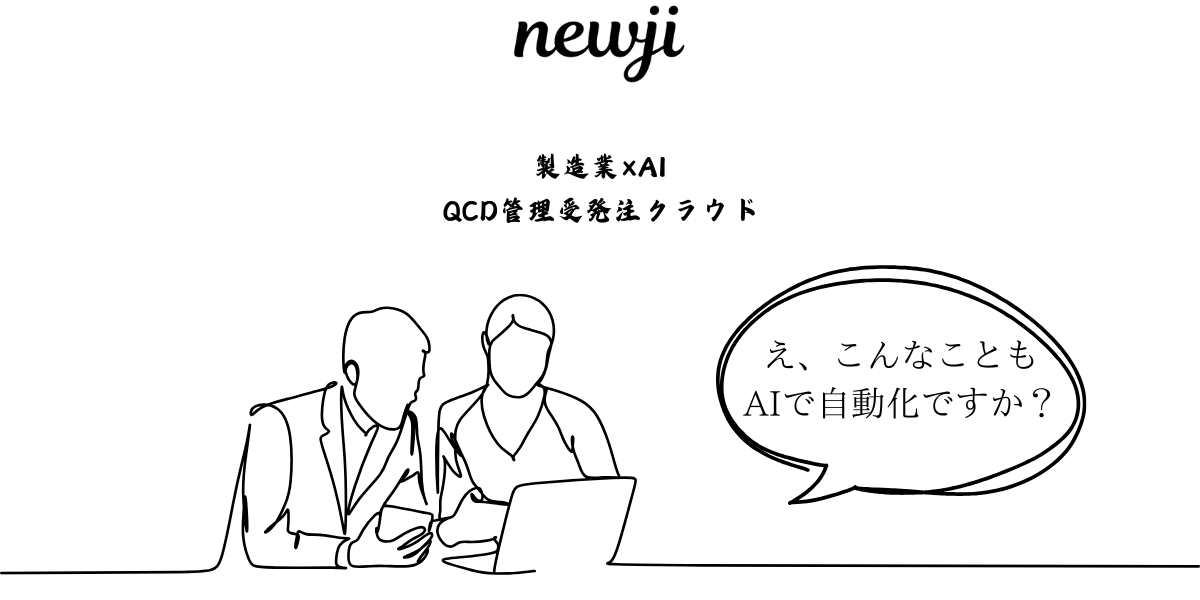
Bayesian decision theory and predictive distributions
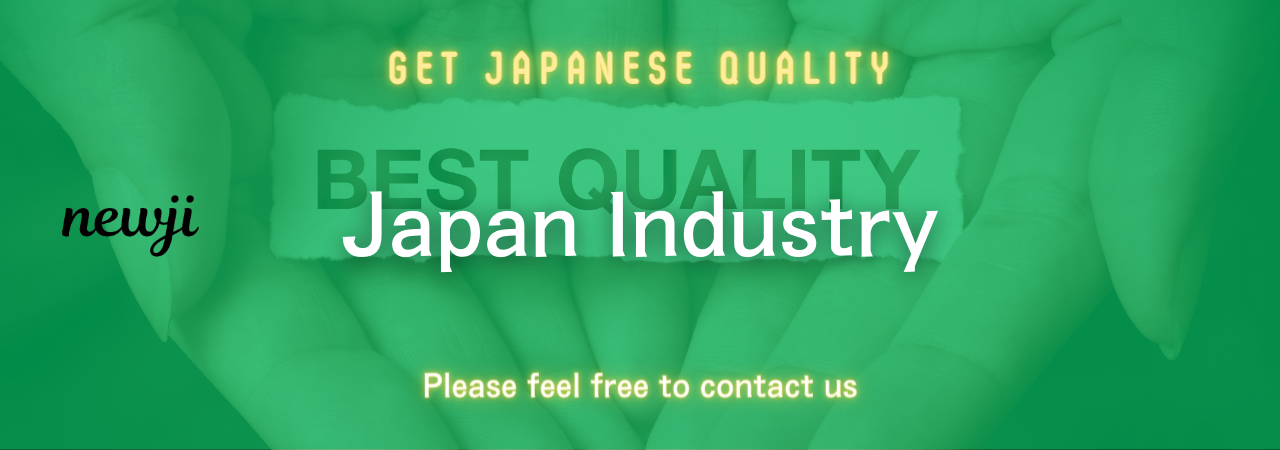
目次
Understanding Bayesian Decision Theory
Bayesian decision theory is a fundamental concept in the field of statistics and machine learning.
It provides a mathematical framework for making decisions in uncertain conditions.
This theory relies on Bayes’ theorem, which offers a way to update our beliefs based on new evidence.
Bayesian decision theory effectively combines prior knowledge with current data to make informed decisions.
Imagine you are trying to decide whether to carry an umbrella.
If the weather forecast says there might be rain, you will consider your prior experiences with similar forecasts and decide accordingly.
This is a simple application of Bayesian decision theory in everyday life.
In more formal terms, the theory evaluates the probabilities of different outcomes and chooses the one that minimizes potential losses or maximizes expected benefits.
The decision-maker uses a loss function, which quantifies the cost associated with different decisions and outcomes.
By integrating this with probabilistic information, Bayesian decision theory helps in choosing the optimal decision under uncertainty.
The Role of Predictive Distributions
Predictive distributions are crucial in Bayesian decision theory.
They provide a statistical foundation for anticipating future events based on available data.
By using predictive distributions, we estimate the likelihood of different outcomes, which aids in the decision-making process.
Predictive distributions come into play after defining a probabilistic model of the phenomenon we are interested in.
For instance, consider predicting the demand for a product.
With a probabilistic model in place, we can use past sales data to generate a predictive distribution.
This helps us estimate the likely demand in the future, allowing businesses to manage inventory effectively.
In Bayesian statistics, the predictive distribution is derived from the posterior distribution, which represents our updated beliefs after considering new data.
This update is critical because it enables us to refine our predictions as new information becomes available.
In this way, predictive distributions help us manage uncertainty about future events and guide better decision-making.
Application of Bayesian Decision Theory
Bayesian decision theory finds applications in a variety of fields, owing to its robust approach in handling uncertainty.
Let’s explore some of these applications to understand its practical significance.
Medical Diagnosis
In healthcare, Bayesian decision theory plays a crucial role in diagnostic processes.
Doctors often face uncertainty when diagnosing diseases, as symptoms can be indicative of multiple conditions.
By integrating prior medical knowledge with patient-specific data, Bayesian decision theory helps doctors make more accurate decisions.
For instance, if a patient arrives with a set of symptoms, the doctor can use Bayesian decision theory to estimate the probabilities of different diseases.
They can then weigh these probabilities against the risks of potential treatment outcomes, choosing the option that best balances these considerations.
Financial Decision Making
In the realm of finance, investment decisions are often made under uncertain conditions.
Bayesian decision theory assists financial analysts in evaluating potential investments by taking into account both historical data and market trends.
By doing so, investors can make more informed decisions that optimize their expected returns while minimizing risk.
For example, portfolio managers use Bayesian inference to update their beliefs about the returns of different assets as new market data becomes available.
This approach allows them to adjust their portfolios dynamically, balancing potential gains against the risk of loss.
Machine Learning
Bayesian methods are fundamental to various machine learning models, especially in areas like classification and regression.
By incorporating prior distributions with observed data, Bayesian machine learning models can improve predictions and account for uncertainty more effectively.
For classifiers, Bayesian decision theory helps in deciding which class an observation belongs to by evaluating the posterior probabilities of each class.
This allows for a more nuanced classification, as decisions are made based on the likelihood of various outcomes rather than just a single prediction.
Overcoming Challenges with Bayesian Decision Theory
While Bayesian decision theory offers numerous benefits, it does come with its own set of challenges.
One major challenge is computational complexity, as Bayesian methods often require integrating over large spaces of parameters, which can be computationally intensive.
Moreover, defining appropriate prior distributions can be subjective and challenging.
The choice of priors significantly influences the posterior distribution and, consequently, the decision-making process.
Therefore, it is vital to choose priors wisely, balancing between being informative and not overly biased.
Despite these challenges, advances in computational techniques and growing data availability have made Bayesian decision theory more accessible and practical.
Methods like Markov Chain Monte Carlo (MCMC) and Variational Inference have significantly reduced the computational burden, allowing for widespread application in complex models.
Conclusion
Bayesian decision theory is a potent tool for navigating uncertainty and making informed decisions.
By integrating prior information with new data through predictive distributions, it offers a structured approach to evaluate potential outcomes and optimize decision-making.
As we continue to deal with increasingly complex data environments and uncertainties, the relevance and applicability of Bayesian decision theory will only grow.
Its ability to improve decision-making in fields ranging from medicine to finance and machine learning makes it an indispensable part of the modern analytical toolkit.
In summary, by understanding and applying Bayesian decision theory, individuals and organizations can better manage the uncertainty inherent in various aspects of life, leading to more informed and rational decisions.
資料ダウンロード
QCD管理受発注クラウド「newji」は、受発注部門で必要なQCD管理全てを備えた、現場特化型兼クラウド型の今世紀最高の受発注管理システムとなります。
ユーザー登録
受発注業務の効率化だけでなく、システムを導入することで、コスト削減や製品・資材のステータス可視化のほか、属人化していた受発注情報の共有化による内部不正防止や統制にも役立ちます。
NEWJI DX
製造業に特化したデジタルトランスフォーメーション(DX)の実現を目指す請負開発型のコンサルティングサービスです。AI、iPaaS、および先端の技術を駆使して、製造プロセスの効率化、業務効率化、チームワーク強化、コスト削減、品質向上を実現します。このサービスは、製造業の課題を深く理解し、それに対する最適なデジタルソリューションを提供することで、企業が持続的な成長とイノベーションを達成できるようサポートします。
製造業ニュース解説
製造業、主に購買・調達部門にお勤めの方々に向けた情報を配信しております。
新任の方やベテランの方、管理職を対象とした幅広いコンテンツをご用意しております。
お問い合わせ
コストダウンが利益に直結する術だと理解していても、なかなか前に進めることができない状況。そんな時は、newjiのコストダウン自動化機能で大きく利益貢献しよう!
(β版非公開)