- お役立ち記事
- Bayesian estimation and Markov random fields
月間76,176名の
製造業ご担当者様が閲覧しています*
*2025年3月31日現在のGoogle Analyticsのデータより
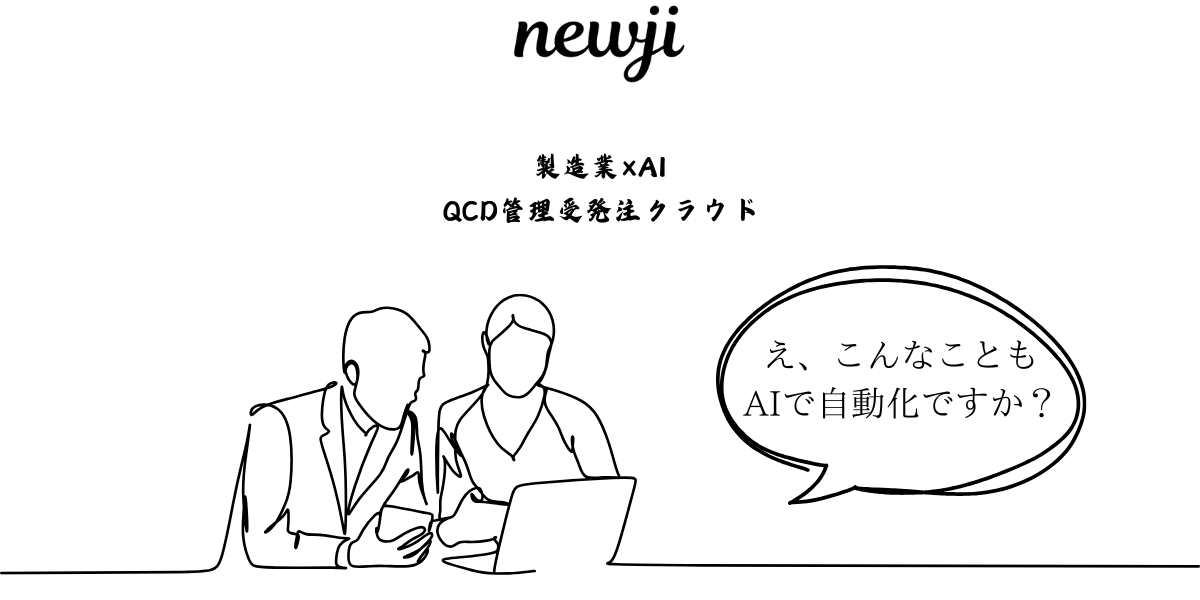
Bayesian estimation and Markov random fields
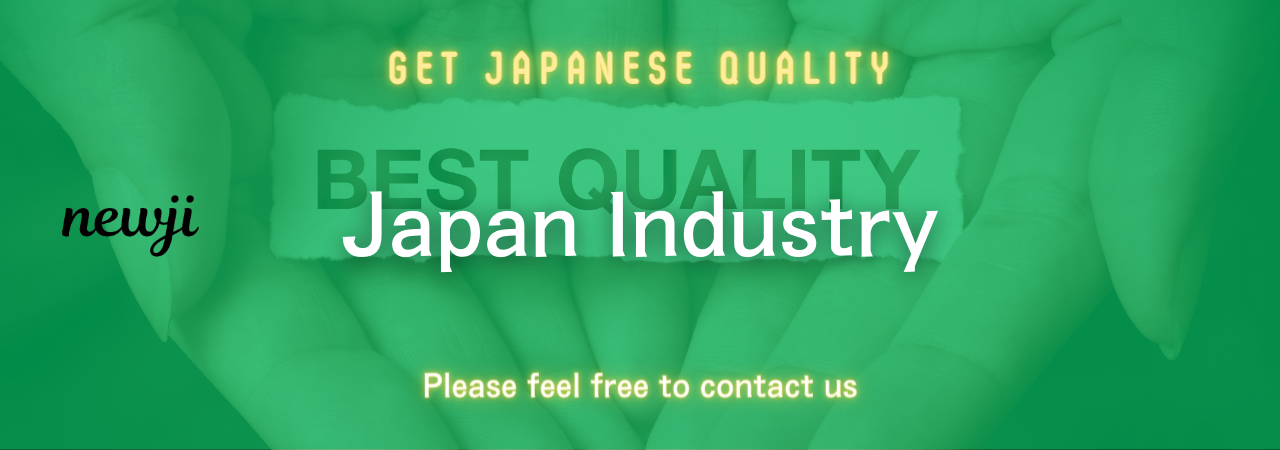
目次
Introduction to Bayesian Estimation
Bayesian estimation is a statistical technique that has gained popularity due to its ability to incorporate prior knowledge into the estimation process.
It’s named after Thomas Bayes, an 18th-century statistician, whose work laid the groundwork for this probabilistic approach.
This technique combines prior information with current data to provide a more comprehensive understanding of uncertainty.
The Basics of Bayesian Estimation
At its core, Bayesian estimation revolves around the Bayes’ Theorem.
The theorem provides a way to update the probability of a hypothesis, based on new evidence.
In a mathematical form, it’s expressed as:
\[ P(H|E) = \frac{P(E|H) \cdot P(H)}{P(E)} \]
Where:
– \(P(H|E)\) is the posterior probability.
– \(P(E|H)\) is the likelihood.
– \(P(H)\) is the prior probability.
– \(P(E)\) is the evidence probability.
This simple yet powerful formula allows statisticians and researchers to update their beliefs in light of new data.
Applications of Bayesian Estimation
Bayesian estimation is widely used in various fields, ranging from economics to machine learning.
In economics, for example, it helps economists make predictions about market trends by incorporating both prior market conditions and current data.
In the field of machine learning, Bayesian algorithms are utilized to improve model predictions by adjusting weights in light of new data.
One of the compelling attributes of Bayesian estimation is its flexibility.
It accommodates different types of data and complex models, which makes it especially useful in areas with high uncertainty or limited data.
Understanding Markov Random Fields
Markov Random Fields (MRFs) are a set of models used in statistical mechanics and image processing.
They represent a collection of random variables having a Markov property described by an undirected graph.
Markov Random Fields are adept at modeling the spatial dependencies of variables, which is why they’re prevalent in image analysis and computer vision.
The Markov Property
The Markov property is a key component in understanding Markov Random Fields.
A random field satisfies the Markov property if each variable is conditionally independent of all others, given its neighbors.
In other words, the future state is independent of the past states, given the present state.
This assumption simplifies the modelling of complex interactions between variables.
Applications of Markov Random Fields
Markov Random Fields have been successfully applied in various applications.
In image processing, for instance, they help in tasks like image segmentation, denoising, and texture recognition.
By modeling the pixels of an image as nodes of an MRF, researchers can effectively analyze the image’s structure and properties.
Beyond image processing, MRFs are also used in spatial statistics.
They help model the spatial distribution of landscapes or weather patterns, taking into account local dependencies and variations.
Bayesian Estimation and Markov Random Fields Combined
When Bayesian estimation is combined with Markov Random Fields, the result is a powerful tool for probabilistic modeling and inference.
This combination leverages the strengths of both techniques: the ability to incorporate prior information through Bayesian approaches and the capability to model spatial dependencies via Markov Random Fields.
Applications of the Combined Approach
A common application is in the field of computer vision, where both approaches are used to improve image analysis.
For instance, in image segmentation, prior knowledge about the likely shapes and positions of objects can be combined with local pixel data to produce more accurate segmentations.
This is achieved by framing the segmentation task as an optimization problem, where both the Markov Random Field model and Bayesian estimation play critical roles in finding the solution.
Furthermore, this combination finds its use in medical imaging, helping in noisy data where uncertainty and spatial dependencies are intrinsic.
Challenges and Future Directions
While the combination of Bayesian estimation and Markov Random Fields offers immense benefits, challenges remain.
Computational complexity is one such challenge, as working with large datasets and complex models requires substantial computational resources.
Recently, advances in computational power and algorithm efficiency have been mitigating these issues.
Future developments in machine learning techniques, like deep learning, may provide novel ways to harness these models’ full potential.
As researchers continue to develop more efficient algorithms, we can expect the application of Bayesian estimation and Markov Random Fields to expand into more diverse fields, such as finance, robotics, and environmental science.
Conclusion
Bayesian estimation and Markov Random Fields are pivotal tools in the realm of probabilistic modeling.
Individually, they offer robust frameworks for incorporating prior knowledge and modeling spatial dependencies.
When combined, they provide a comprehensive approach to complex data analysis tasks.
Despite challenges such as computational demands, the future is promising as ongoing research and technological improvements continue to advance these techniques.
Mastering these concepts opens the door to innovative applications across an array of scientific domains.
資料ダウンロード
QCD管理受発注クラウド「newji」は、受発注部門で必要なQCD管理全てを備えた、現場特化型兼クラウド型の今世紀最高の受発注管理システムとなります。
ユーザー登録
受発注業務の効率化だけでなく、システムを導入することで、コスト削減や製品・資材のステータス可視化のほか、属人化していた受発注情報の共有化による内部不正防止や統制にも役立ちます。
NEWJI DX
製造業に特化したデジタルトランスフォーメーション(DX)の実現を目指す請負開発型のコンサルティングサービスです。AI、iPaaS、および先端の技術を駆使して、製造プロセスの効率化、業務効率化、チームワーク強化、コスト削減、品質向上を実現します。このサービスは、製造業の課題を深く理解し、それに対する最適なデジタルソリューションを提供することで、企業が持続的な成長とイノベーションを達成できるようサポートします。
製造業ニュース解説
製造業、主に購買・調達部門にお勤めの方々に向けた情報を配信しております。
新任の方やベテランの方、管理職を対象とした幅広いコンテンツをご用意しております。
お問い合わせ
コストダウンが利益に直結する術だと理解していても、なかなか前に進めることができない状況。そんな時は、newjiのコストダウン自動化機能で大きく利益貢献しよう!
(β版非公開)