- お役立ち記事
- Calculating Bayesian estimators and utilizing Monte Carlo methods
Calculating Bayesian estimators and utilizing Monte Carlo methods
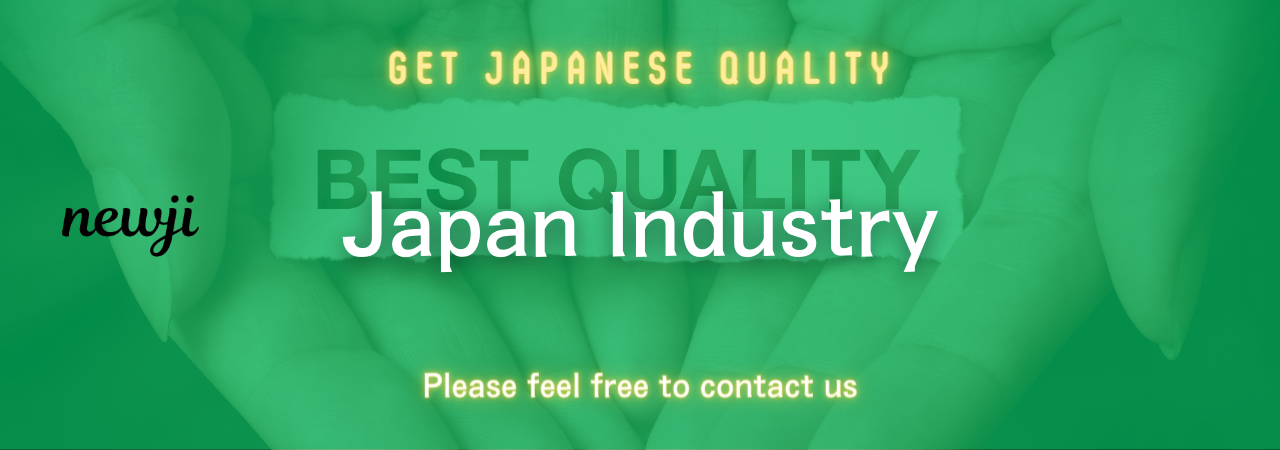
目次
Understanding Bayesian Estimators
Bayesian estimators are a fundamental concept in statistics and data science.
They are used to update the probability of a hypothesis as new evidence or information becomes available.
The Bayesian approach provides a mathematical framework to incorporate prior knowledge along with new data to make informed predictions or decisions.
The foundation of Bayesian estimation is Bayes’ Theorem.
This theorem describes the way to update the probabilities of hypotheses based on the collection of new evidence.
In simple terms, it combines prior probability with the likelihood of current data to form a posterior probability.
This posterior probability is then used as the updated belief about the parameters or hypotheses in question.
Components of Bayesian Estimation
1. **Prior Distribution**: This represents the initial belief about the parameter before any evidence is taken into account.
It is based on previous knowledge or assumptions and is often chosen subjectively.
2. **Likelihood Function**: This indicates the probability of the observed data under different parameter values.
The likelihood function is derived from the statistical model that describes how data is generated.
3. **Posterior Distribution**: This is the updated probability distribution of the parameters after considering the new data.
The posterior is a combination of the prior distribution and the likelihood function.
4. **Bayes’ Theorem**: It mathematically combines the prior and the likelihood to produce the posterior.
The theorem is expressed as:
\[ Posterior \propto Likelihood \times Prior \]
Monte Carlo Methods in Bayesian Estimation
Monte Carlo methods are a group of computational algorithms that rely on repeated random sampling to obtain numerical results.
These methods are particularly useful in Bayesian estimation when dealing with complex models or where analytical solutions are intractable.
Why Use Monte Carlo Methods?
1. **Integration Over Parameter Space**: Bayesian inference involves integration across complex multidimensional spaces, which can be computationally challenging.
Monte Carlo methods approximate these integrals by sampling from probability distributions.
2. **Flexibility**: Monte Carlo methods can handle a wide range of models and data types.
They are not limited by the mathematical tractability of the problem.
3. **Scalability**: These methods can be scaled for high-dimensional data sets and large parameter spaces, making them suitable for modern data science applications.
Common Monte Carlo Techniques
1. **Markov Chain Monte Carlo (MCMC)**: This is one of the most popular Monte Carlo methods used in Bayesian analysis.
MCMC generates samples from the posterior distribution by constructing a Markov Chain.
The chain moves through the sample space in such a way that its stationary distribution matches the target posterior distribution.
Variants of MCMC, like the Metropolis-Hastings and Gibbs sampling algorithms, are frequently used.
2. **Importance Sampling**: This method approximates the posterior distribution by sampling from an importance distribution.
The samples are weighted according to their likelihood under the target distribution.
Importance sampling is efficient when the importance distribution is a close match to the target distribution.
3. **Sequential Monte Carlo (SMC)**: Also known as particle filters, SMC methods are used to estimate changing systems that evolve over time.
They work by propagating a set of samples, called particles, through time, updating them with new data.
Steps to Calculate Bayesian Estimators with Monte Carlo Methods
To calculate Bayesian estimators using Monte Carlo methods, follow these general steps:
Define the Prior
Begin by defining your prior distribution.
This should reflect any previous knowledge you have about the parameters you are estimating.
Choose a conjugate prior if available, as this simplifies calculations.
Establish the Likelihood Function
Define the likelihood function based on your statistical model.
This function captures the probability of the observed data given different parameter values.
Select a Monte Carlo Method
Choose the appropriate Monte Carlo method for your problem.
For many Bayesian problems, MCMC is the go-to method due to its flexibility and effectiveness.
Generate Samples
Use your chosen Monte Carlo method to generate samples from the posterior distribution.
In the case of MCMC, this involves running the Markov Chain for a sufficiently long time to ensure convergence to the stationary distribution.
Estimate the Parameters
Once you have the samples, use them to estimate the parameters of interest.
The mean, median, or mode of the sampled distribution can serve as point estimates for the parameters.
Uncertainty in the estimates can be assessed by analyzing the spread of the samples.
Applications of Bayesian Estimators and Monte Carlo Methods
Bayesian estimators and Monte Carlo methods have widespread applications across various fields:
1. **Finance**: Estimating the volatility of stock prices and risk assessment.
2. **Machine Learning**: Improving the accuracy of predictive models by incorporating prior knowledge.
3. **Healthcare**: Analyzing medical data to evaluate the efficacy of treatments.
4. **Environmental Science**: Modeling and predicting climate changes.
Understanding and utilizing Bayesian estimators and Monte Carlo methods equip data scientists and statisticians with powerful tools to make informed, probabilistic decisions.
These techniques allow for a flexible approach to modeling uncertainty, accommodating both subjectivity and empirical data.
As technology and data continue to evolve, the relevance and application of these methods are set to expand even further.
資料ダウンロード
QCD調達購買管理クラウド「newji」は、調達購買部門で必要なQCD管理全てを備えた、現場特化型兼クラウド型の今世紀最高の購買管理システムとなります。
ユーザー登録
調達購買業務の効率化だけでなく、システムを導入することで、コスト削減や製品・資材のステータス可視化のほか、属人化していた購買情報の共有化による内部不正防止や統制にも役立ちます。
NEWJI DX
製造業に特化したデジタルトランスフォーメーション(DX)の実現を目指す請負開発型のコンサルティングサービスです。AI、iPaaS、および先端の技術を駆使して、製造プロセスの効率化、業務効率化、チームワーク強化、コスト削減、品質向上を実現します。このサービスは、製造業の課題を深く理解し、それに対する最適なデジタルソリューションを提供することで、企業が持続的な成長とイノベーションを達成できるようサポートします。
オンライン講座
製造業、主に購買・調達部門にお勤めの方々に向けた情報を配信しております。
新任の方やベテランの方、管理職を対象とした幅広いコンテンツをご用意しております。
お問い合わせ
コストダウンが利益に直結する術だと理解していても、なかなか前に進めることができない状況。そんな時は、newjiのコストダウン自動化機能で大きく利益貢献しよう!
(Β版非公開)