- お役立ち記事
- Collaborative technology of CAE, machine learning, and data assimilation and its applications
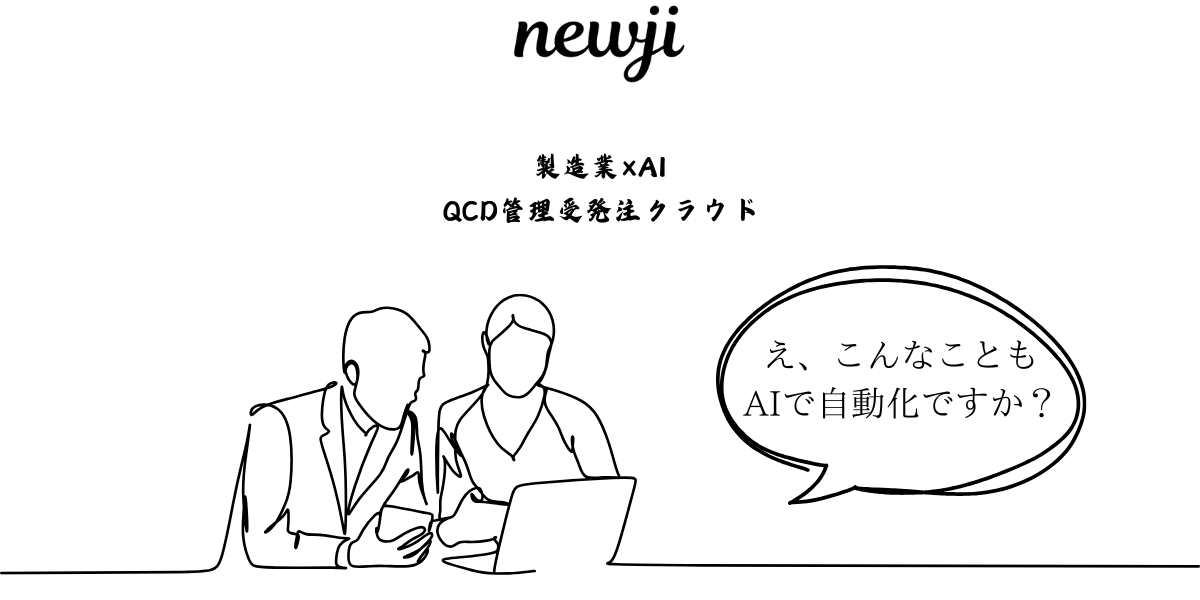
Collaborative technology of CAE, machine learning, and data assimilation and its applications
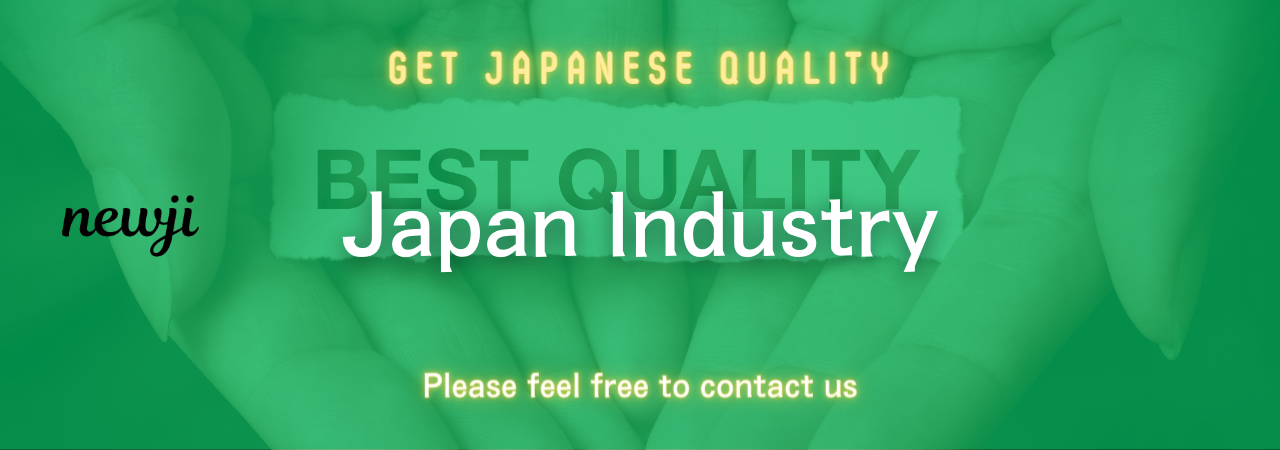
目次
Introduction to Collaborative Technology
Collaborative technology is at the forefront of today’s engineering and computing innovations.
Its key components—Computer-Aided Engineering (CAE), machine learning, and data assimilation—are transforming multiple sectors.
These technologies work together to solve complex problems, making processes more efficient and improving decision-making capabilities.
Let’s dive deeper into how these components operate individually and collaboratively, their applications, and how they’re changing the landscape in various industries.
What is Computer-Aided Engineering (CAE)?
Computer-Aided Engineering (CAE) refers to the use of computer software to simulate performance in order to improve product designs.
It encompasses a broad range of applications, including simulation, validation, and optimization of product engineering designs.
CAE tools enable engineers to evaluate the functionality of a design idea, allowing them to optimize even before physical prototypes are created.
CAE is used across various industries, from automotive to aerospace and consumer electronics.
The main advantage of CAE is its ability to predict how products will perform, which helps reduce prototyping costs and accelerate time-to-market.
Understanding Machine Learning
Machine learning is a branch of artificial intelligence that builds algorithms to learn from data, identify patterns, and make decisions with minimal human intervention.
In the context of collaborative technology, machine learning is integral in processing large datasets and predicting outcomes based on historical information.
Machine learning models are capable of analyzing complex datasets faster and more accurately than traditional methods.
This technology is used extensively in sectors such as finance, healthcare, and retail to automate and refine processes.
Exploring Data Assimilation
Data assimilation involves the integration of real-world data with computational models.
The goal is to improve the accuracy of predictions by adjusting models with observed data.
This approach is crucial in fields like meteorology, where prediction accuracy is vital.
In collaborative technology, data assimilation helps improve the reliability of machine predictions by feeding real-time data back into the models.
This results in a dynamic system that adapts and evolves based on incoming data.
Collaborative Technology: A Powerful Trio
When CAE, machine learning, and data assimilation come together, they form a powerful trifecta.
This integration allows for enhanced predictive capabilities and optimization, transforming the design and decision-making processes in engineering and technology industries.
Benefits of Integration
1. **Enhanced Predictive Accuracy**: Combining machine learning with CAE models allows for more precise predictions.
Data assimilation fine-tunes these predictions by incorporating real-world data, leading to improved accuracy.
2. **Optimized Design Process**: With CAE, engineers can quickly assess various design options.
Machine learning algorithms can identify the most efficient design based on past data.
Incorporating live data through data assimilation can further refine these solutions.
3. **Reduced Time and Cost**: The synergy of these technologies reduces the need for extensive physical testing, lowering costs and speeding up the design process.
This efficiency is a major advantage in competitive industries.
Applications in Various Industries
1. **Automotive Industry**: Automotive manufacturers use collaborative technology to enhance vehicle design and performance.
CAE tools help in simulating crash tests and aerodynamics, while machine learning algorithms predict maintenance needs.
Data assimilation ensures these predictions stay accurate by incorporating real-time data from vehicles.
2. **Healthcare Sector**: In healthcare, these technologies help improve diagnosis and treatment plans.
Machine learning models analyze patient data to predict outcomes.
CAE simulations are used in device design and surgical planning, and data assimilation keeps these predictions relevant with real-world patient data.
3. **Aerospace Engineering**: Here, CAE simulates aerodynamic properties and structural integrity of aircraft.
Machine learning optimizes design elements, and data assimilation aids in predicting weather conditions affecting flights.
This integration ensures safety and efficiency in operations.
4. **Energy and Resources**: For energy companies, forecasting energy consumption and optimizing resource distribution are critical.
These technologies collaborate to balance supply and demand efficiently, integrate renewable energy sources, and predict equipment failures.
5. **Consumer Electronics**: CAE helps in the design and durability testing of gadgets.
Machine learning enhances user experience through personalized interfaces.
Data assimilation improves product performance by integrating usage data into the design loop.
Challenges and Future Directions
While the integration of CAE, machine learning, and data assimilation offers numerous benefits, certain challenges persist.
1. **Data Privacy and Security**: Managing large datasets raises concerns over data protection.
Companies must ensure robust security measures to guard sensitive information.
2. **Skill Gap**: Implementing these technologies requires specialized skills.
Businesses must invest in training their workforce to ensure they can leverage these tools effectively.
3. **Interoperability**: Combining different systems and tools can lead to compatibility issues.
Developing industry standards could enhance the seamless integration of these technologies.
Looking Ahead
The future of collaborative technology is bright, with opportunities for further innovation on the horizon.
As computational capabilities advance, expect even more sophisticated simulations, smarter algorithms, and improved accuracy in predictions.
Industries will continue exploring these technologies to remain competitive and drive progress.
Conclusion
The collaborative technology of CAE, machine learning, and data assimilation marks a transformative evolution in how industries operate.
The synergy between these components facilitates efficient processes, accurate predictions, and optimized designs.
While challenges remain, the potential benefits and applications across various sectors underscore the importance of continuing to refine and integrate these technologies.
With continued advancements, this innovative triad promises to shape the future of design, engineering, and beyond.
資料ダウンロード
QCD調達購買管理クラウド「newji」は、調達購買部門で必要なQCD管理全てを備えた、現場特化型兼クラウド型の今世紀最高の購買管理システムとなります。
ユーザー登録
調達購買業務の効率化だけでなく、システムを導入することで、コスト削減や製品・資材のステータス可視化のほか、属人化していた購買情報の共有化による内部不正防止や統制にも役立ちます。
NEWJI DX
製造業に特化したデジタルトランスフォーメーション(DX)の実現を目指す請負開発型のコンサルティングサービスです。AI、iPaaS、および先端の技術を駆使して、製造プロセスの効率化、業務効率化、チームワーク強化、コスト削減、品質向上を実現します。このサービスは、製造業の課題を深く理解し、それに対する最適なデジタルソリューションを提供することで、企業が持続的な成長とイノベーションを達成できるようサポートします。
オンライン講座
製造業、主に購買・調達部門にお勤めの方々に向けた情報を配信しております。
新任の方やベテランの方、管理職を対象とした幅広いコンテンツをご用意しております。
お問い合わせ
コストダウンが利益に直結する術だと理解していても、なかなか前に進めることができない状況。そんな時は、newjiのコストダウン自動化機能で大きく利益貢献しよう!
(Β版非公開)