- お役立ち記事
- Controller parameter tuning technology using Bayesian optimization and its applications
月間76,176名の
製造業ご担当者様が閲覧しています*
*2025年3月31日現在のGoogle Analyticsのデータより
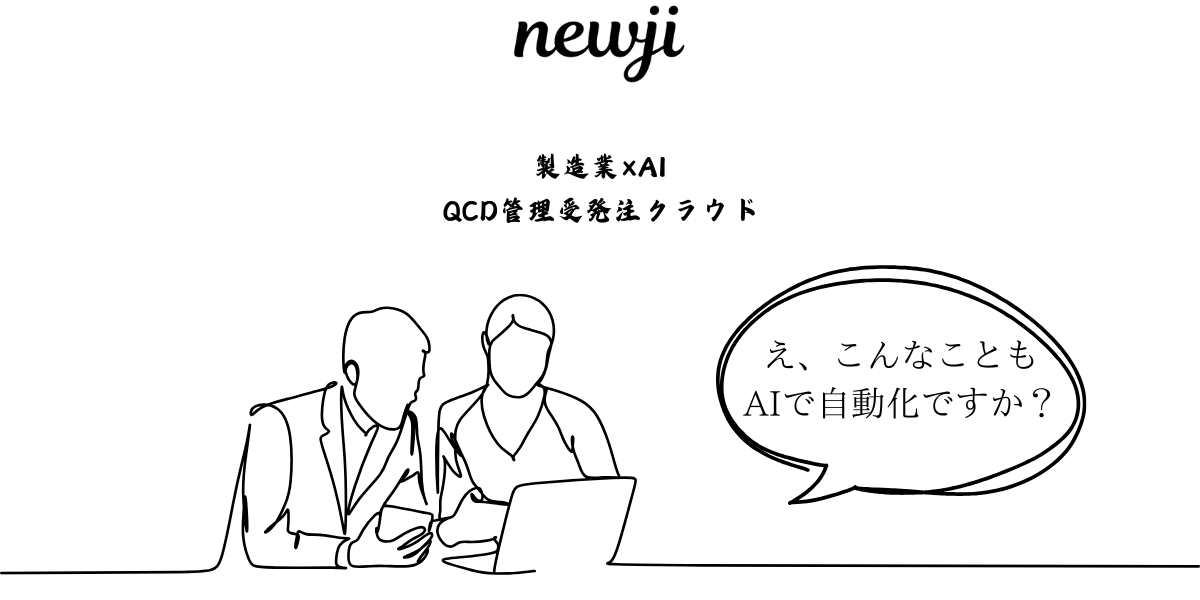
Controller parameter tuning technology using Bayesian optimization and its applications
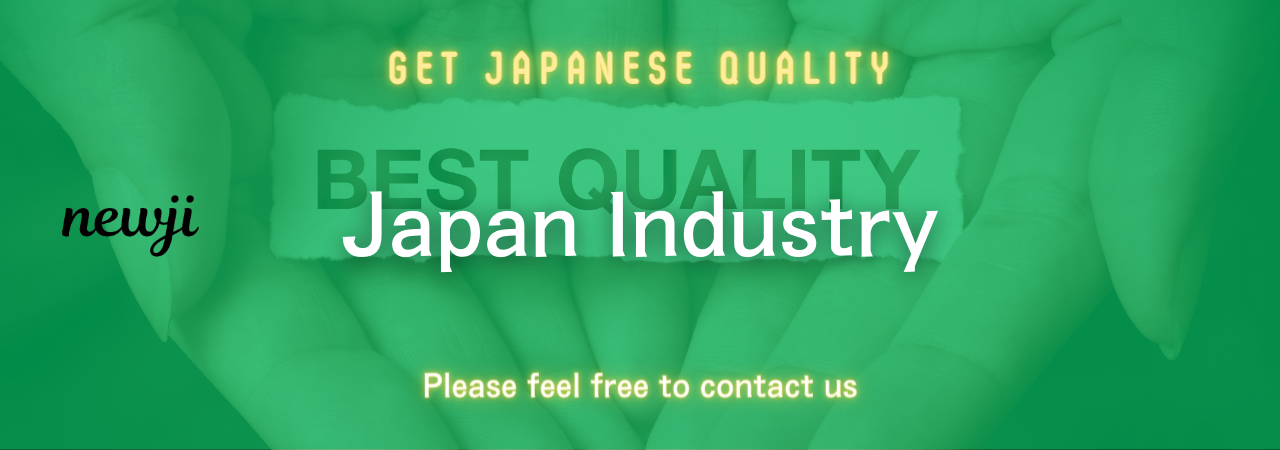
目次
Introduction to Controller Parameter Tuning
Controller parameter tuning is a critical process in control systems, influencing the overall performance and efficiency of various engineering applications.
In essence, it involves adjusting the parameters of a controller to achieve desired system performance characteristics such as stability, responsiveness, and accuracy.
Traditional methods of tuning controllers often involve trial and error or heuristic techniques which can be time-consuming and inefficient.
Fortunately, advances in computational technologies have led to the development of more sophisticated methods, such as Bayesian optimization, to enhance this process.
Understanding Bayesian Optimization
Bayesian optimization is a statistical method aimed at optimizing objective functions that are often expensive to evaluate.
It is particularly suited for problems where the function lacks an analytical formula, making it a perfect fit for tuning controller parameters.
The approach involves building a probabilistic model, usually through Gaussian processes, of the objective function and using it to predict the performance of untested settings.
One of the critical advantages of Bayesian optimization is its sample efficiency.
It seeks to find the global optimum in fewer iterations, which is extremely beneficial when each function evaluation is costly or time-consuming.
This feature is particularly valuable in controller parameter tuning, where testing a new set of parameters might involve running extensive simulations or live experiments, especially in systems like robotics or aerospace.
How Bayesian Optimization Works in Tuning
Bayesian optimization uses a surrogate model to approximate the objective function.
The process typically follows these steps:
1. **Initial Sampling**: Begin with a small set of sample points, evaluated at different parameter settings.
2. **Model Fitting**: Fit a Gaussian process model to these initial samples to form a posterior distribution of the objective function.
3. **Acquisition Function**: Use an acquisition function to decide the next points to sample.
This function balances exploration (testing unknown settings) and exploitation (refining known good settings).
4. **Iterative Tuning**: Evaluate the selected parameters from the acquisition function, update the surrogate model, and repeat the process until convergence or a predefined budget is exhausted.
Advantages in Controller Tuning
The benefits of applying Bayesian optimization to controller parameter tuning are manifold:
– **Efficiency**: Reduces the number of trials needed to find optimal parameters, saving time and resources.
– **Global Search**: Capable of escaping local optima to find the best possible parameter settings.
– **Robustness**: Less sensitive to the noise in measurements, which is common in real-world systems.
– **Adaptability**: Easily applicable to various types of controllers and systems without requiring deep domain knowledge.
Applications of Bayesian Optimization in Industry
Bayesian optimization is making waves in numerous industries due to its flexible and powerful capabilities.
Here are some key applications:
Automotive and Aerospace
In automotive and aerospace industries, the precise tuning of controller parameters is crucial for vehicle dynamics and stability control systems.
Bayesian optimization allows engineers to effectively fine-tune parameters related to braking systems, suspension settings, and flight controls, among others.
This contributes to improved safety, efficiency, and performance.
Robotics
Robots often operate in dynamic environments where they must adapt to changing conditions.
Bayesian optimization is used to fine-tune the control parameters of robots to ensure they respond accurately to their surroundings.
From robotic arms in manufacturing to autonomous drones, this technique helps achieve reliable and efficient operations.
Manufacturing
In manufacturing systems, controller tuning is essential for precise automation and process control.
Bayesian optimization facilitates the tuning of PID controllers, for instance, ensuring product quality, reducing waste, and increasing throughput.
This technology can be applied to diverse processes including chemical production, food processing, and electronics fabrication.
Renewable Energy
For renewable energy systems like wind turbines and solar panels, optimal controller parameter tuning is vital for maximizing energy capture and system longevity.
Bayesian optimization helps in adjusting the control settings to cater to varying environmental conditions, thereby enhancing the efficiency and reliability of energy conversion processes.
Challenges and Future Prospects
Despite its advantages, implementing Bayesian optimization comes with challenges.
The computational demand can be significant, especially for high-dimensional parameter spaces.
Moreover, the quality of results heavily depends on the chosen acquisition function and surrogate model.
Looking forward, ongoing research is focused on overcoming these challenges.
Developments in parallel computing and machine learning are poised to streamline Bayesian optimization processes even further.
Also, integrating this technology with real-time adaptive systems could open new horizons in automatic and on-the-fly tuning of controllers.
Conclusion
Bayesian optimization represents a powerful and efficient strategy for tuning controller parameters across various applications.
Its ability to find optimal solutions with fewer evaluations makes it an attractive alternative to traditional tuning methods.
As industries continue to automate and improve their systems, the role of Bayesian optimization is expected to grow, driving innovations and enhancing the performance of control systems worldwide.
資料ダウンロード
QCD管理受発注クラウド「newji」は、受発注部門で必要なQCD管理全てを備えた、現場特化型兼クラウド型の今世紀最高の受発注管理システムとなります。
ユーザー登録
受発注業務の効率化だけでなく、システムを導入することで、コスト削減や製品・資材のステータス可視化のほか、属人化していた受発注情報の共有化による内部不正防止や統制にも役立ちます。
NEWJI DX
製造業に特化したデジタルトランスフォーメーション(DX)の実現を目指す請負開発型のコンサルティングサービスです。AI、iPaaS、および先端の技術を駆使して、製造プロセスの効率化、業務効率化、チームワーク強化、コスト削減、品質向上を実現します。このサービスは、製造業の課題を深く理解し、それに対する最適なデジタルソリューションを提供することで、企業が持続的な成長とイノベーションを達成できるようサポートします。
製造業ニュース解説
製造業、主に購買・調達部門にお勤めの方々に向けた情報を配信しております。
新任の方やベテランの方、管理職を対象とした幅広いコンテンツをご用意しております。
お問い合わせ
コストダウンが利益に直結する術だと理解していても、なかなか前に進めることができない状況。そんな時は、newjiのコストダウン自動化機能で大きく利益貢献しよう!
(β版非公開)