- お役立ち記事
- Data analysis and implementation examples
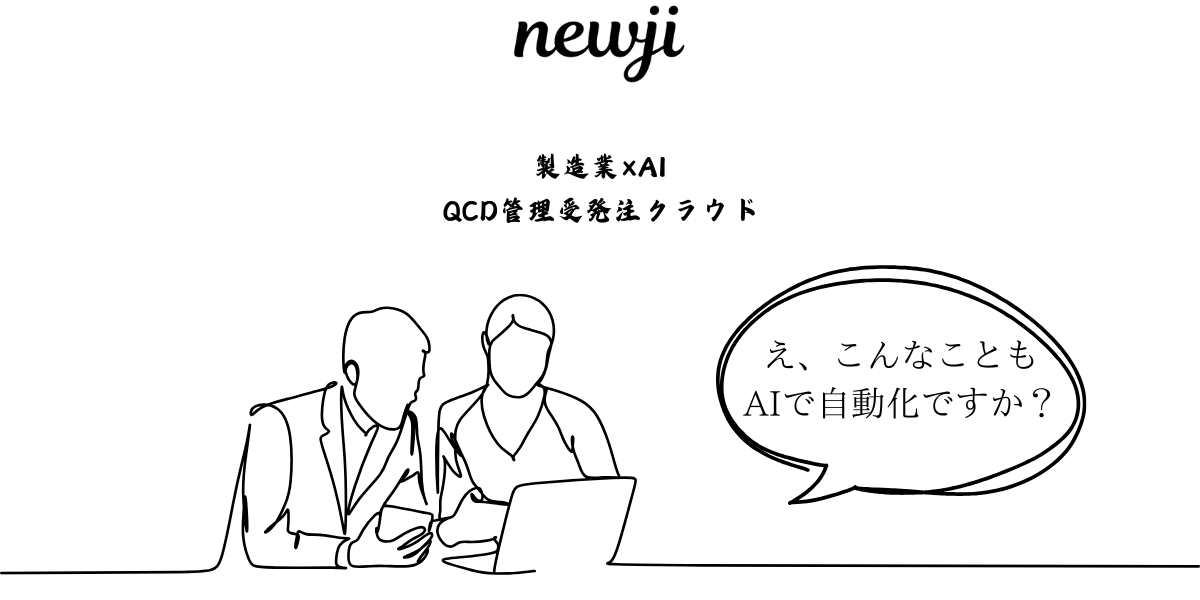
Data analysis and implementation examples
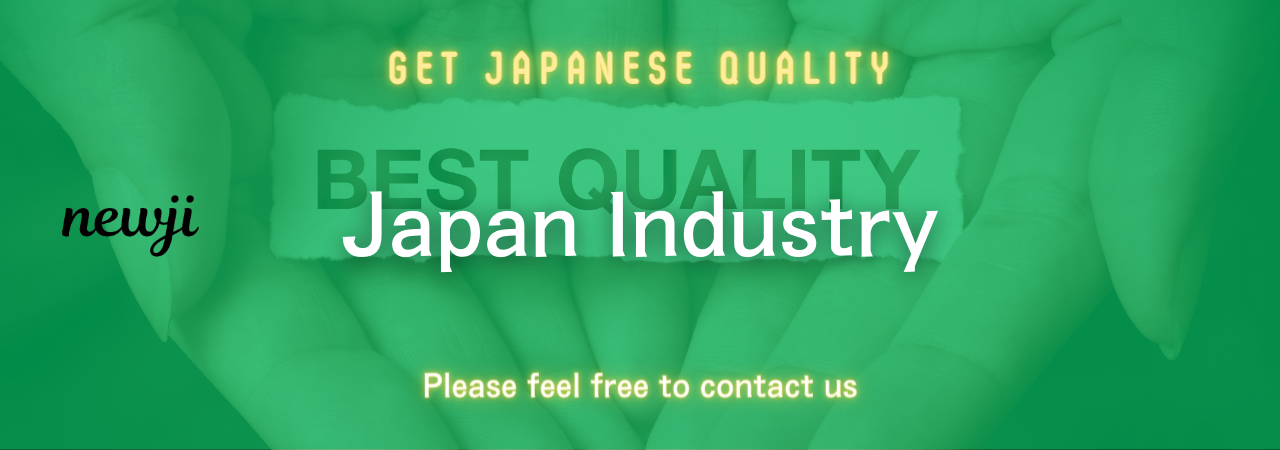
目次
Understanding Data Analysis
Data analysis involves examining datasets to draw conclusions about the information they contain.
It incorporates various statistical and logical techniques to evaluate data, transform it into useful outputs, and aid decision-making.
Data analysis is crucial for businesses and organizations as it helps them understand their performance, predict future trends, and make evidence-based decisions.
The process of data analysis typically involves several key steps.
Firstly, defining the questions or problems that need addressing is essential.
Next, data is collected from relevant sources, followed by cleaning and organizing it to ensure accuracy.
Subsequently, analytical techniques are applied to extract meaningful insights, which are then interpreted and communicated to stakeholders.
Types of Data Analysis
There are various types of data analysis, each serving different purposes:
1. **Descriptive Analysis**: This is about summarizing past data, often using statistics such as averages or percentages, to understand past performance.
2. **Diagnostic Analysis**: This involves examining data to understand the reasons behind past outcomes.
It’s like peeling the layers of an onion to explore underlying issues.
3. **Predictive Analysis**: By using historical data, predictive analysis forecasts future events.
Machine learning algorithms often play a significant role here to identify trends and patterns.
4. **Prescriptive Analysis**: This type explores various scenarios and predicts outcomes to recommend actions to achieve desired results.
Tools Used in Data Analysis
Various tools are available for data analysis, each catering to different needs and user expertise levels.
Some popular options include:
– **Excel**: A versatile tool for basic and intermediate data analysis tasks, widely used due to its ease of use and accessibility.
– **Python**: With libraries like Pandas, NumPy, and Matplotlib, Python provides powerful capabilities for complex data analysis.
– **R**: Known for its statistical computing capabilities, R is ideal for users needing advanced data analysis techniques.
– **Tableau**: This tool allows users to easily visualize data in an interactive and understandable format.
– **SQL**: Primarily used for querying databases, SQL is essential for extracting necessary data for analysis.
Examples of Data Analysis in Use
Data analysis is applied across various sectors with differing objectives and outcomes:
In Healthcare
Healthcare providers use data analysis to improve patient care, enhance operational efficiency, and manage costs.
For example, by analyzing patient data, healthcare institutions can identify trends in disease outbreaks, optimize staff allocation, and improve treatment plans based on historical patient outcomes.
In Retail
Retailers leverage data analysis to better understand customer behavior, manage inventory, and enhance the shopping experience.
Predictive analytics helps in forecasting product demand, while customer data analysis aids in personalized marketing strategies and optimizing product placement.
In Finance
Financial institutions apply data analysis to manage risks, detect fraud, and comply with regulations.
Advanced algorithms analyze transaction data to identify suspicious activities and assess potential credit risks, thus enabling effective risk management.
In Sports
Sports teams use data analysis to enhance player performance, devise game strategies, and improve fan engagement.
Analyzing player statistics and health data helps optimize training regimens and match tactics to achieve better outcomes on the field.
Challenges in Data Analysis
While data analysis offers numerous benefits, it also comes with challenges:
– **Data Quality**: Inaccurate or incomplete data leads to unreliable analyses and decisions.
Ensuring data cleanliness and accuracy is vital for effective analysis.
– **Privacy and Security**: Handling sensitive data responsibly while complying with privacy regulations remains a major concern for organizations.
– **Complexity**: With data growing in volume and variety, analyzing it effectively can be complex and requires skilled personnel.
– **Integration**: Combining data from various sources and formats presents integration challenges, demanding powerful tools or technologies.
Future of Data Analysis
As technology evolves, so does the field of data analysis, driven by:
– **AI and Machine Learning**: These technologies enable more sophisticated analysis, automation of routine tasks, and development of more accurate predictive models.
– **Real-time Analysis**: The demand for immediate insights is leading to the rise of real-time data analysis capabilities, critical for sectors like finance and security.
– **Big Data**: Organizations increasingly rely on big data analysis to understand consumer behavior, optimize operations, and innovate.
Embracing these advancements will allow businesses to stay competitive and glean more valuable insights from their data.
By understanding and implementing data analysis correctly, companies can unlock the potential hidden in their datasets, resulting in better strategies, improved operations, and gaining a competitive edge.
資料ダウンロード
QCD調達購買管理クラウド「newji」は、調達購買部門で必要なQCD管理全てを備えた、現場特化型兼クラウド型の今世紀最高の購買管理システムとなります。
ユーザー登録
調達購買業務の効率化だけでなく、システムを導入することで、コスト削減や製品・資材のステータス可視化のほか、属人化していた購買情報の共有化による内部不正防止や統制にも役立ちます。
NEWJI DX
製造業に特化したデジタルトランスフォーメーション(DX)の実現を目指す請負開発型のコンサルティングサービスです。AI、iPaaS、および先端の技術を駆使して、製造プロセスの効率化、業務効率化、チームワーク強化、コスト削減、品質向上を実現します。このサービスは、製造業の課題を深く理解し、それに対する最適なデジタルソリューションを提供することで、企業が持続的な成長とイノベーションを達成できるようサポートします。
オンライン講座
製造業、主に購買・調達部門にお勤めの方々に向けた情報を配信しております。
新任の方やベテランの方、管理職を対象とした幅広いコンテンツをご用意しております。
お問い合わせ
コストダウンが利益に直結する術だと理解していても、なかなか前に進めることができない状況。そんな時は、newjiのコストダウン自動化機能で大きく利益貢献しよう!
(Β版非公開)