- お役立ち記事
- Data Application Techniques in Manufacturing: From Collection to Analysis to Decision Making
Data Application Techniques in Manufacturing: From Collection to Analysis to Decision Making
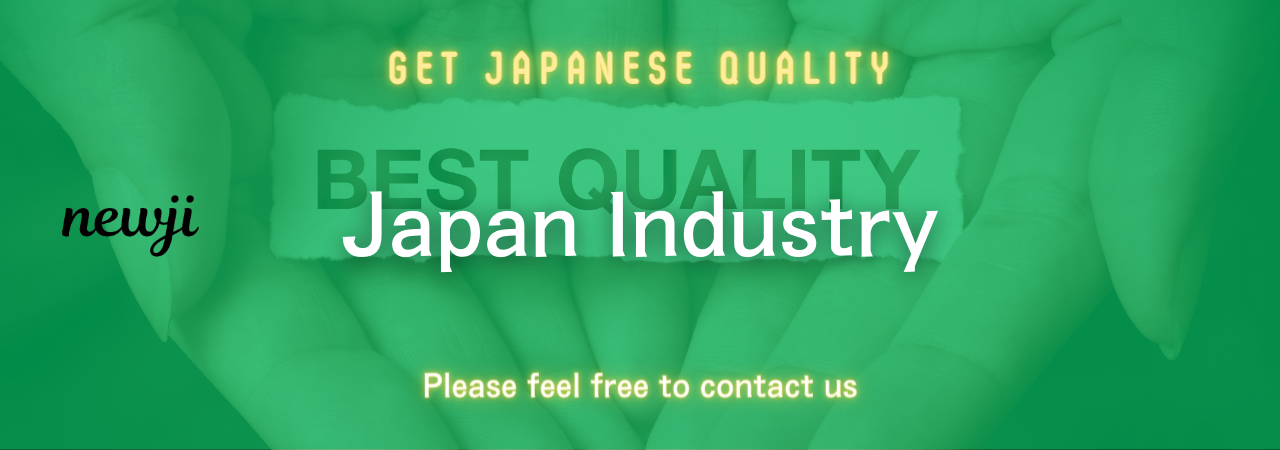
In the ever-evolving landscape of manufacturing, leveraging data effectively has become a keystone for businesses striving to enhance operational efficiency, improve productivity, and sustain competitive advantages.
Data application techniques in manufacturing encompass several stages, from collection to analysis and ultimately decision making.
Understanding these stages is crucial for any organization aiming to modernize its manufacturing processes.
目次
Data Collection: The Foundation Stone
The first step in the application of data techniques in manufacturing is data collection.
Manufacturing environments are filled with a myriad of data sources.
These include sensor readings from IoT devices, machine logs, production records, and quality control metrics.
The key here is to gather relevant and accurate data efficiently.
Manufacturers often use advanced sensors and IoT devices to collect real-time data from machines and other equipment.
These devices can yield a continuous stream of information regarding machine performance, temperature, runtime, and other critical parameters.
Another method is manual data entry, although this is less favored due to the potential for human error and time consumption.
Moreover, integrating different data sources into a unified system is essential.
Data silos can significantly hinder the ability to derive meaningful insights, so employing data integration tools and middleware is important to ensure seamless data collection.
Data Storage and Management: Organizing the Treasure Trove
Once collected, data must be appropriately stored and managed to be beneficial.
Deploying robust data storage solutions like cloud storage, data lakes, or on-premises servers can secure data and make it easily accessible.
Cloud storage, in particular, offers scalability and flexibility, which are invaluable for handling large volumes of manufacturing data.
Data management processes are equally important to maintain data quality.
This involves cleaning the data to remove any inaccuracies or redundancies and structuring it to facilitate smooth analysis.
Utilizing databases and management systems like SQL databases or NoSQL solutions can aid in effectively organizing and retrieving data when needed.
Data Analysis: Turning Data into Insights
Data analysis transforms raw data into insightful information, guiding manufacturers in making informed decisions.
Various techniques and tools are available to conduct this analysis effectively.
Descriptive Analytics
Descriptive analytics helps in understanding what has happened in the manufacturing process.
By examining historical data, manufacturers can determine patterns and trends.
Tools such as dashboards and reporting software provide visual representations of data, making it easier to interpret.
Predictive Analytics
Predictive analytics leverage historical data to predict future outcomes.
Machine learning models and algorithms can forecast machine downtime, quality defects, or other important events, enabling proactive measures to be taken.
This not only helps in maintenance scheduling but also improves overall efficiency and reduces unexpected downtimes.
Prescriptive Analytics
Prescriptive analytics goes a step further by recommending actions based on the data analysis.
It answers the question of what should be done by suggesting optimization strategies and decision-making pathways.
This can be especially useful for supply chain optimization, inventory management, and production scheduling.
Data Visualization: Making Sense of Data
Data visualization plays a pivotal role in data analysis by converting complex data sets into easily understandable visual formats.
Graphs, charts, and dashboards can provide a quick and clear overview of manufacturing operations, performance metrics, and problem areas.
Interactive visualization tools allow users to drill down into data, offering deeper insights and aiding in strategic planning.
Decision Making: From Insights to Actions
The ultimate goal of data application techniques in manufacturing is to aid in decision making.
Data-driven decision making ensures that choices are backed by solid evidence rather than gut feelings.
Here’s how it works.
Operational Decisions
On the shop floor, real-time data can inform decisions about equipment use, workforce allocation, and production scheduling.
For instance, if data indicates a machine is nearing its maintenance threshold, operators can schedule maintenance to avoid unexpected breakdowns, enhancing productivity.
Strategic Decisions
At a higher level, aggregated data from various sources helps in making long-term strategic decisions.
This includes forecasting demand, planning inventory, and evaluating the performance of different manufacturing processes.
Strategic decisions benefit from a holistic view of data, aligning business goals with operational capabilities.
Enhanced Quality Control
Data-driven decision making extends to quality control, where data from production processes can be analyzed to identify defects and root causes.
This enables manufacturers to implement quality improvement initiatives and reduce waste, ultimately leading to better product quality.
Challenges and Solutions
Implementing data application techniques in manufacturing comes with its set of challenges.
Data privacy and security remain prime concerns.
To mitigate these, manufacturers can employ robust cybersecurity measures and comply with data protection regulations.
Another challenge is the need for skilled personnel who can manage and analyze data effectively.
Investing in training and education programs for employees can bridge this skill gap.
Additionally, leveraging automation and AI tools can reduce the reliance on human intervention and streamline data processes.
The Future of Data Application in Manufacturing
The future of manufacturing will be increasingly data-centric.
Emerging technologies like Edge computing, AI, and machine learning will further revolutionize data application techniques.
These advancements will make it possible to analyze data at the source, provide real-time insights, and enable autonomous decision making.
However, achieving this future requires a proactive approach towards embracing these technologies and continuously evolving data practices.
Organizations that do so will be well-positioned to reap the benefits of enhanced efficiency, improved product quality, and sustained competitive advantage.
資料ダウンロード
QCD調達購買管理クラウド「newji」は、調達購買部門で必要なQCD管理全てを備えた、現場特化型兼クラウド型の今世紀最高の購買管理システムとなります。
ユーザー登録
調達購買業務の効率化だけでなく、システムを導入することで、コスト削減や製品・資材のステータス可視化のほか、属人化していた購買情報の共有化による内部不正防止や統制にも役立ちます。
NEWJI DX
製造業に特化したデジタルトランスフォーメーション(DX)の実現を目指す請負開発型のコンサルティングサービスです。AI、iPaaS、および先端の技術を駆使して、製造プロセスの効率化、業務効率化、チームワーク強化、コスト削減、品質向上を実現します。このサービスは、製造業の課題を深く理解し、それに対する最適なデジタルソリューションを提供することで、企業が持続的な成長とイノベーションを達成できるようサポートします。
オンライン講座
製造業、主に購買・調達部門にお勤めの方々に向けた情報を配信しております。
新任の方やベテランの方、管理職を対象とした幅広いコンテンツをご用意しております。
お問い合わせ
コストダウンが利益に直結する術だと理解していても、なかなか前に進めることができない状況。そんな時は、newjiのコストダウン自動化機能で大きく利益貢献しよう!
(Β版非公開)