- お役立ち記事
- Data assimilation and Bayesian statistics
月間76,176名の
製造業ご担当者様が閲覧しています*
*2025年3月31日現在のGoogle Analyticsのデータより
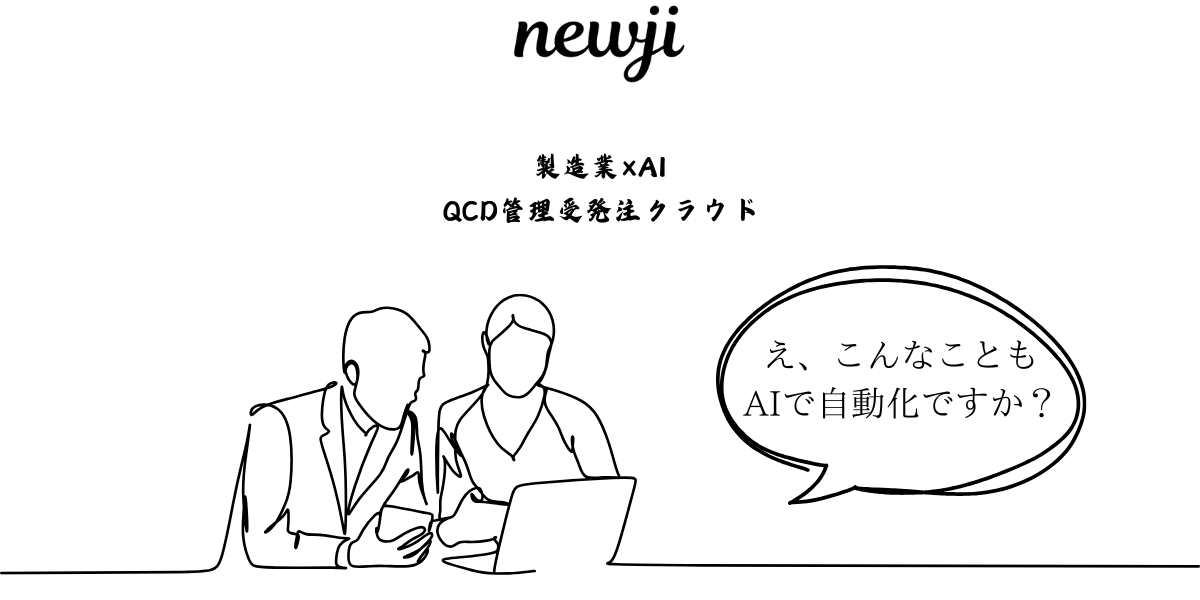
Data assimilation and Bayesian statistics
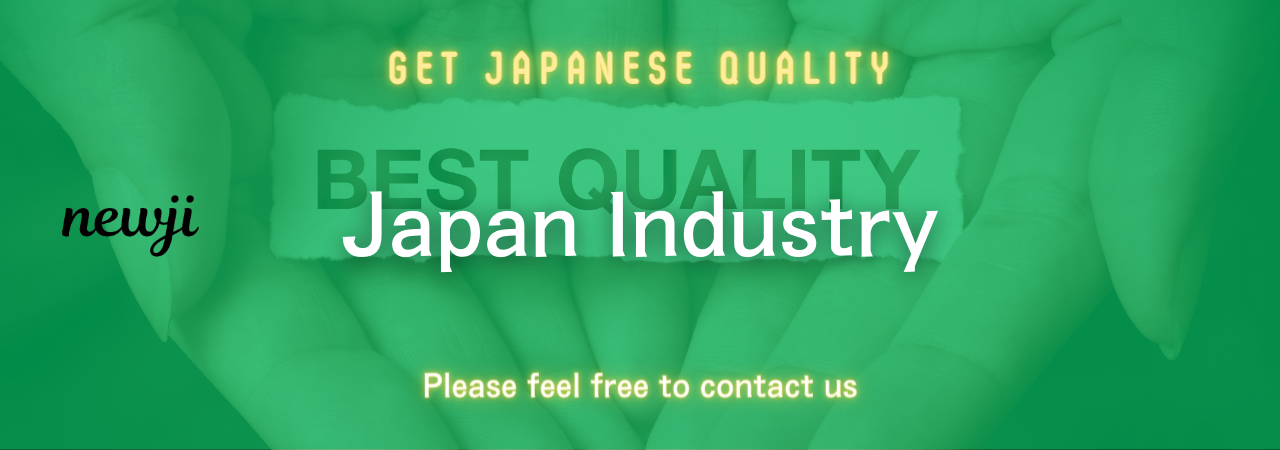
目次
Understanding Data Assimilation
Data assimilation is a crucial technique that makes numerical models more accurate by continuously updating them with real-world data.
Imagine scientists trying to predict the weather.
They start with a mathematical model based on physical laws and then improve it by assimilating observations like temperature and air pressure collected from the atmosphere.
The process of data assimilation helps in reducing errors, making forecasts more reliable.
At its core, data assimilation is a blend of predictions and observations.
It allows models to adjust and refine their outputs by incorporating new data as they come in.
This dynamic updating is crucial in various fields such as meteorology, oceanography, and even financial forecasting.
One common method used in data assimilation is the Kalman filter.
This iterative mathematical process updates predictions based on new incoming data.
The Kalman filter can efficiently estimate the state of a dynamic system, making it a popular choice in many industries.
Applications of Data Assimilation
Data assimilation is employed in numerous fields.
In meteorology, it plays a pivotal role in weather forecasting.
Modern-day weather predictions heavily rely on assimilated data to capture the rapidly changing atmospheric conditions accurately.
In oceanography, data assimilation helps model sea surface temperatures and currents.
It assists in understanding and predicting phenomena like El Niño, which have widespread environmental impacts.
Even in financial markets, data assimilation techniques aid in making informed decisions.
Financial analysts use these methods to integrate diverse data sources, improving risk assessments and investment strategies.
Exploring Bayesian Statistics
Bayesian statistics is a powerful branch of statistics that involves updating the probability of a hypothesis as more evidence becomes available.
It offers a flexible framework for reasoning under uncertainty.
The core principle behind Bayesian statistics is Bayes’ theorem.
It mathematically combines prior information with new evidence to form an updated probability, known as the posterior probability.
Bayesian statistics are particularly useful for cases where new information continually emerges.
This makes it an excellent tool for machine learning, medical diagnosis, and risk analysis.
Bayes’ Theorem in Action
Bayes’ theorem is expressed as:
\[ P(H|E) = \frac{P(E|H) \cdot P(H)}{P(E)} \]
Where:
– \( P(H|E) \) is the posterior probability of hypothesis \( H \) given the evidence \( E \).
– \( P(E|H) \) is the likelihood of evidence \( E \) given that hypothesis \( H \) is true.
– \( P(H) \) is the prior probability of the hypothesis before seeing the evidence.
– \( P(E) \) is the probability of evidence.
This formula allows us to update our beliefs systematically.
For instance, in medical diagnosis, it helps update the diagnosis probability as new symptoms or test results arrive.
Applications of Bayesian Statistics
Bayesian statistics find applications in various fields.
In machine learning, Bayesian methods help in model selection and parameter estimation.
They allow models to be adaptive, learning from the data as it becomes available.
In clinical trials, Bayesian statistics provide a framework for making decisions with incomplete data.
It helps in assessing the effectiveness of new treatments, enabling more informed medical decisions.
Risk management also benefits from Bayesian approaches.
In finance, Bayesian methods evaluate uncertain market conditions, aiding in decision-making and strategy formulation.
Combining Data Assimilation and Bayesian Statistics
Combining data assimilation and Bayesian statistics leads to a robust approach in dealing with dynamic systems.
Both methodologies complement each other by continuously updating models with new data and understanding uncertainty.
In weather forecasting, Bayesian data assimilation methods are emerging as a powerful tool.
By incorporating Bayesian statistics, meteorologists can better capture the uncertainties in weather models, leading to more accurate predictions.
In healthcare, integrating Bayesian statistics with data assimilation helps refine patient monitoring systems.
By constantly updating medical models with real-time patient data, healthcare providers can improve diagnostics and treatment plans.
In conclusion, data assimilation and Bayesian statistics are essential tools in today’s data-driven world.
Their applications span across numerous fields, from enhancing weather forecasts to refining financial analyses.
By understanding these concepts, industries can harness the full potential of their data, improving decision-making processes and outcomes.
資料ダウンロード
QCD管理受発注クラウド「newji」は、受発注部門で必要なQCD管理全てを備えた、現場特化型兼クラウド型の今世紀最高の受発注管理システムとなります。
ユーザー登録
受発注業務の効率化だけでなく、システムを導入することで、コスト削減や製品・資材のステータス可視化のほか、属人化していた受発注情報の共有化による内部不正防止や統制にも役立ちます。
NEWJI DX
製造業に特化したデジタルトランスフォーメーション(DX)の実現を目指す請負開発型のコンサルティングサービスです。AI、iPaaS、および先端の技術を駆使して、製造プロセスの効率化、業務効率化、チームワーク強化、コスト削減、品質向上を実現します。このサービスは、製造業の課題を深く理解し、それに対する最適なデジタルソリューションを提供することで、企業が持続的な成長とイノベーションを達成できるようサポートします。
製造業ニュース解説
製造業、主に購買・調達部門にお勤めの方々に向けた情報を配信しております。
新任の方やベテランの方、管理職を対象とした幅広いコンテンツをご用意しております。
お問い合わせ
コストダウンが利益に直結する術だと理解していても、なかなか前に進めることができない状況。そんな時は、newjiのコストダウン自動化機能で大きく利益貢献しよう!
(β版非公開)