- お役立ち記事
- Data Assimilation Basics for Digital Twin Development and Applications
Data Assimilation Basics for Digital Twin Development and Applications
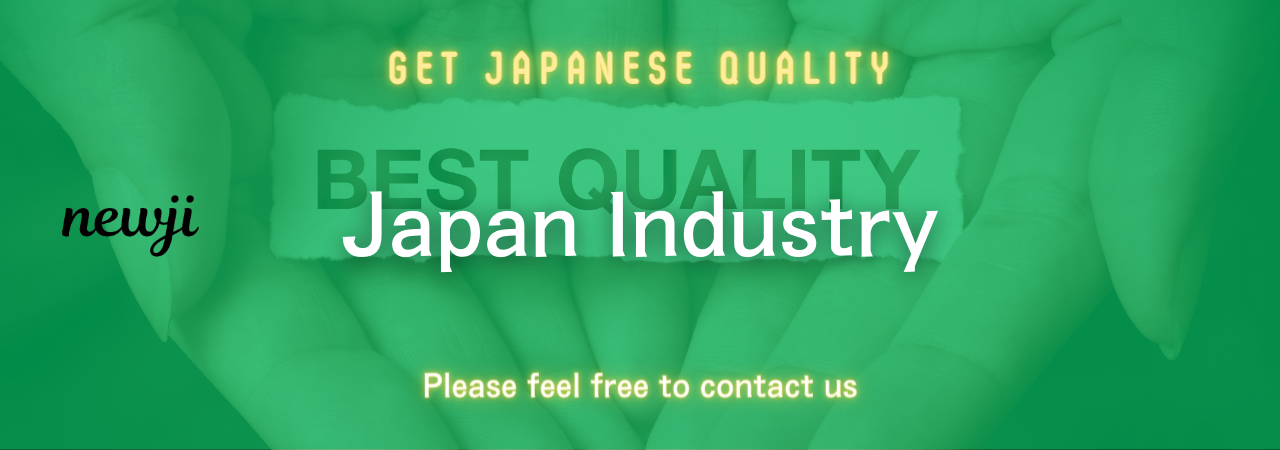
目次
Understanding Data Assimilation
Data assimilation is a concept that involves integrating real-world data into a computer model to improve its accuracy and reliability.
Imagine you have a weather model that predicts tomorrow’s weather.
By feeding this model the latest weather observations, you can improve its predictions.
This is the essence of data assimilation.
It is not just about adding more data, but about updating the model so it reflects the true state of the system.
This approach is crucial in many fields like meteorology, oceanography, and, more recently, in developing digital twins.
What Is a Digital Twin?
Digital twins are virtual replicas of physical systems.
They can be anything from an aircraft engine to a manufacturing plant.
By creating and analyzing these digital models, businesses can predict and optimize the performance of their physical counterparts.
For digital twins to be effective, they need to mirror the real-world system accurately, which is where data assimilation plays a vital role.
The Role of Data Assimilation in Digital Twins
In digital twin development, data assimilation helps in ensuring that the digital twin remains synchronized with its physical counterpart.
As new data becomes available, it is used to adjust and maintain the digital model.
The continuous cycle of updating ensures that the twin is continuously learning and improving, making it a powerful tool for predicting future states and decision-making.
Benefits of Data Assimilation for Digital Twins
Data assimilation offers several significant benefits when applied to digital twins:
Improved Accuracy
By regularly updating digital models with accurate, real-world data, digital twins become more precise.
This enhanced accuracy leads to better predictions and insights.
Real-Time Monitoring
Data assimilation enables real-time updates, ensuring the digital twin mirrors the current state of the physical asset.
This facilitates real-time monitoring and decision-making.
Predictive Maintenance
With accurate, up-to-date models, businesses can predict when a machine might fail or need maintenance.
This proactive approach can save time and money while reducing downtime.
Enhancing Decision-Making
Having access to a precise model allows companies to test different scenarios and their outcomes virtually.
This capability aids in making informed decisions quickly and efficiently.
Applications of Data Assimilation in Digital Twins
Data assimilation is utilized across various industries where digital twins are employed:
Manufacturing
In manufacturing, digital twins are used to optimize production processes.
Data assimilation helps in understanding the production line’s current state, identifying bottlenecks, and improving efficiency.
Healthcare
Digital twins are being developed to simulate human organs and predict outcomes of medical conditions or treatments.
Data assimilation ensures these simulations accurately reflect individual patient conditions, leading to personalized treatment plans.
Aerospace
In aerospace, digital twins help monitor aircraft systems and predict maintenance needs.
Data assimilation allows for the integration of real-time flight data, improving safety and performance.
Smart Cities
Digital twins of urban environments are being developed to manage resources and infrastructure effectively.
Data assimilation helps synchronize these models with real-world data, aiding in traffic management, energy distribution, and emergency response.
Challenges in Data Assimilation for Digital Twins
Despite its benefits, there are challenges associated with integrating data assimilation in digital twin development:
Data Quality
The quality of data fed into a digital twin is crucial.
Inaccurate or incomplete data can lead to faulty models and incorrect conclusions.
Data Integration
Merging data from various sources into a single model can be challenging due to differences in format, scale, and reliability.
Computational Resources
Data assimilation, particularly in real-time, can be resource-intensive.
It requires significant computational power to process and integrate vast amounts of data continuously.
Future of Data Assimilation in Digital Twin Development
The future of data assimilation in digital twin development looks promising as advancements in AI and machine learning continue to expand.
These technologies can enhance data assimilation processes by improving data processing speed and accuracy.
Moreover, the ongoing development of IoT technologies provides more data points, enhancing the completeness and reliability of digital twins.
As the integration of data assimilation in digital twin development progresses, we can expect more refined models capable of providing even more precise predictions and insights.
This will open up new possibilities across industries, from more efficient manufacturing processes to personalized healthcare solutions.
In conclusion, data assimilation plays a crucial role in the development and application of digital twins.
By continuously integrating real-world data, digital twins can accurately mirror their physical counterparts, leading to enhanced monitoring, predictive maintenance, and optimized decision-making.
Despite challenges such as data quality and computational demands, technological advancements hold the promise of overcoming these hurdles, paving the way for more efficient and effective digital twin applications.
資料ダウンロード
QCD調達購買管理クラウド「newji」は、調達購買部門で必要なQCD管理全てを備えた、現場特化型兼クラウド型の今世紀最高の購買管理システムとなります。
ユーザー登録
調達購買業務の効率化だけでなく、システムを導入することで、コスト削減や製品・資材のステータス可視化のほか、属人化していた購買情報の共有化による内部不正防止や統制にも役立ちます。
NEWJI DX
製造業に特化したデジタルトランスフォーメーション(DX)の実現を目指す請負開発型のコンサルティングサービスです。AI、iPaaS、および先端の技術を駆使して、製造プロセスの効率化、業務効率化、チームワーク強化、コスト削減、品質向上を実現します。このサービスは、製造業の課題を深く理解し、それに対する最適なデジタルソリューションを提供することで、企業が持続的な成長とイノベーションを達成できるようサポートします。
オンライン講座
製造業、主に購買・調達部門にお勤めの方々に向けた情報を配信しております。
新任の方やベテランの方、管理職を対象とした幅広いコンテンツをご用意しております。
お問い合わせ
コストダウンが利益に直結する術だと理解していても、なかなか前に進めることができない状況。そんな時は、newjiのコストダウン自動化機能で大きく利益貢献しよう!
(Β版非公開)