- お役立ち記事
- Data-Driven Control Basics and Controller Tuning Techniques
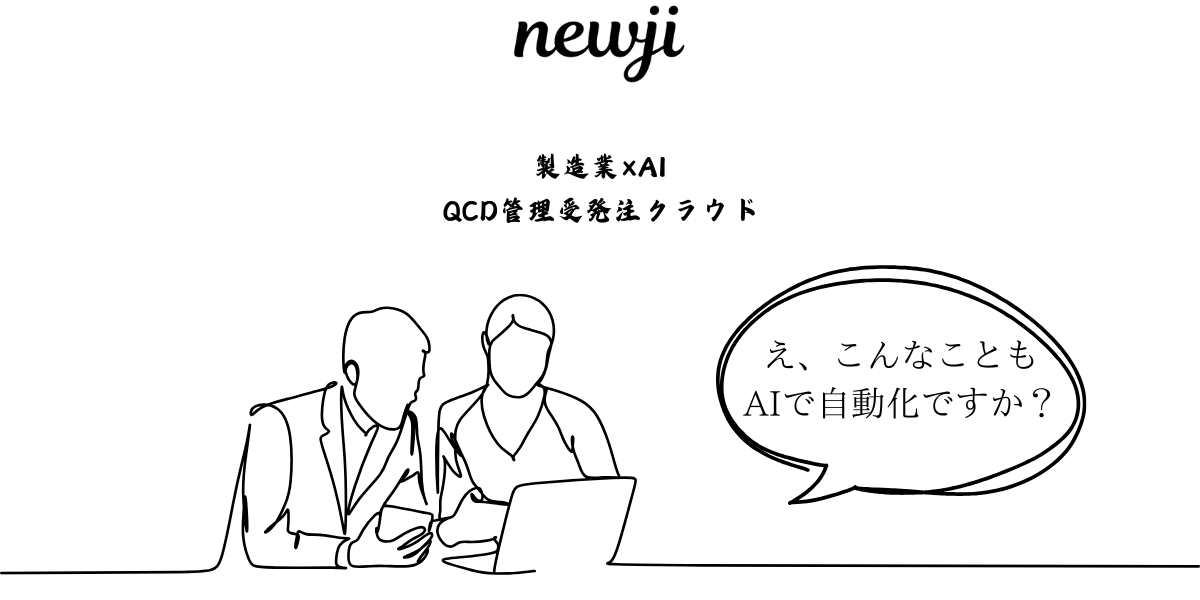
Data-Driven Control Basics and Controller Tuning Techniques
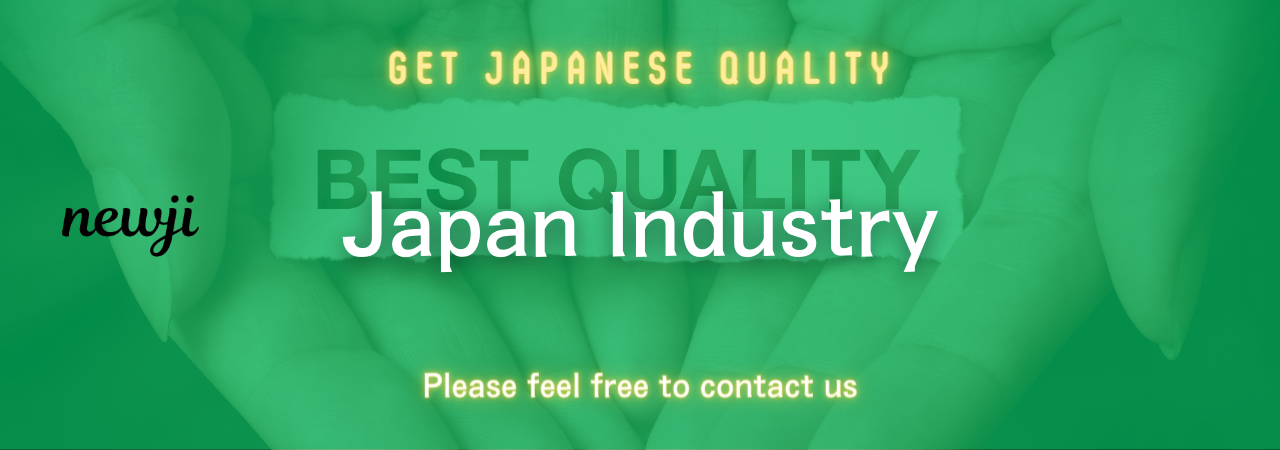
目次
Understanding Data-Driven Control
Data-driven control is a modern approach in control systems where decisions are guided by data rather than theoretical models alone.
This method has become increasingly important as industries prioritize precision and efficiency.
By leveraging data, systems can be fine-tuned to operate optimally under various conditions.
In traditional control systems, controllers are designed based on mathematical models.
These models often rely on assumptions and simplifications of the real-world process.
However, as processes become more complex, these approximations can lead to inefficiencies.
This is where data-driven control steps in, offering a way to enhance performance by directly using data from the system.
The Importance of Data in Control Systems
Data plays a crucial role in modern control systems.
With advancements in sensor technology and data analytics, it’s possible to collect and process large volumes of data in real-time.
This data serves as a valuable resource for understanding system behavior and making informed control decisions.
Data-driven control systems can automatically adjust to changes and uncertainties in the environment.
This adaptability is beneficial in industries such as manufacturing, aerospace, and robotics, where conditions can vary significantly.
Controller Tuning Techniques
Effective controller tuning is essential for achieving desired performance in control systems.
Tuning refers to the process of adjusting controller parameters to optimize system response.
Several techniques are commonly used to achieve this aim.
PID Controller Tuning
PID (Proportional-Integral-Derivative) controllers are widely used due to their simplicity and effectiveness.
Tuning a PID controller involves setting the proportional, integral, and derivative gains to achieve a specific performance.
Several methods exist for PID tuning, including trial-and-error, Ziegler-Nichols, and software-based optimization.
Each method varies in complexity and effectiveness, depending on the system and the desired outcome.
Model-Based Tuning
Model-based tuning involves creating a mathematical representation of the system and using it to derive optimal controller parameters.
This approach can be more accurate than others, as it specifically considers the dynamics of the process.
Simulation tools enable engineers to test various tuning scenarios before applying them to the actual system.
This not only saves time but also minimizes risks associated with incorrect parameter settings.
Adaptive Control
Adaptive control is a technique where the controller parameters are automatically adjusted in real-time based on performance feedback.
This approach is particularly useful in environments where conditions change frequently or are unpredictable.
By continually analyzing system output, adaptive control systems can maintain optimal performance without manual intervention.
This dynamic adjustment sets adaptive control apart from traditional methods.
Challenges and Considerations
Despite its advantages, implementing data-driven control and tuning techniques comes with challenges.
The quality of the data is paramount—poor or inaccurate data can lead to incorrect decisions and suboptimal performance.
Moreover, the complexity of some data-driven methods might be a barrier for industries lacking the necessary technical expertise.
There’s also the issue of data management, as storing and processing large datasets require substantial resources.
Another consideration is the trade-off between model accuracy and computational efficiency.
While complex models might provide better control, they also demand more processing power and can slow down decision-making.
The Future of Data-Driven Control
As technology advances, we can expect data-driven control to become more prevalent.
The integration of artificial intelligence and machine learning with control systems is likely to enhance their capabilities.
These advancements will enable systems to learn and adapt even more efficiently, leading to greater autonomy and improved performance.
Industries stand to gain a significant edge by implementing these modern techniques, as they allow for a more nuanced and responsive approach to controlling processes.
In conclusion, data-driven control, alongside effective controller tuning techniques, represents the future of control systems.
Embracing these methods will not only enhance efficiency and performance but also provide a competitive advantage in an increasingly dynamic world.
資料ダウンロード
QCD調達購買管理クラウド「newji」は、調達購買部門で必要なQCD管理全てを備えた、現場特化型兼クラウド型の今世紀最高の購買管理システムとなります。
ユーザー登録
調達購買業務の効率化だけでなく、システムを導入することで、コスト削減や製品・資材のステータス可視化のほか、属人化していた購買情報の共有化による内部不正防止や統制にも役立ちます。
NEWJI DX
製造業に特化したデジタルトランスフォーメーション(DX)の実現を目指す請負開発型のコンサルティングサービスです。AI、iPaaS、および先端の技術を駆使して、製造プロセスの効率化、業務効率化、チームワーク強化、コスト削減、品質向上を実現します。このサービスは、製造業の課題を深く理解し、それに対する最適なデジタルソリューションを提供することで、企業が持続的な成長とイノベーションを達成できるようサポートします。
オンライン講座
製造業、主に購買・調達部門にお勤めの方々に向けた情報を配信しております。
新任の方やベテランの方、管理職を対象とした幅広いコンテンツをご用意しております。
お問い合わせ
コストダウンが利益に直結する術だと理解していても、なかなか前に進めることができない状況。そんな時は、newjiのコストダウン自動化機能で大きく利益貢献しよう!
(Β版非公開)