- お役立ち記事
- Data Utilization in Quality Control in Manufacturing Industry: Case Studies and Effectiveness of AI and IoT
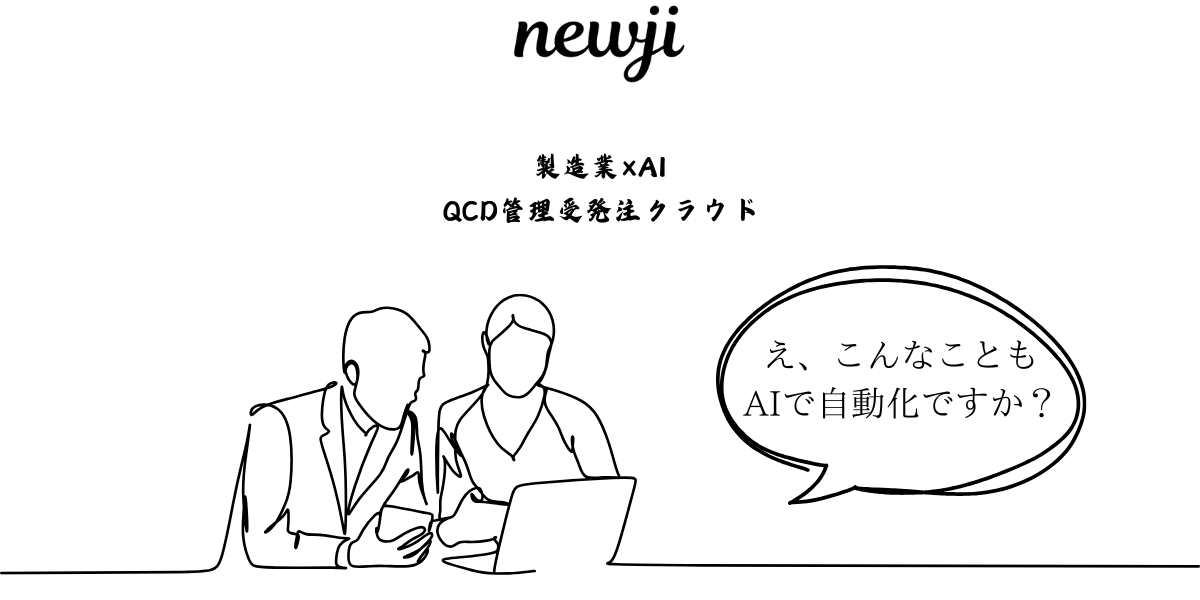
Data Utilization in Quality Control in Manufacturing Industry: Case Studies and Effectiveness of AI and IoT
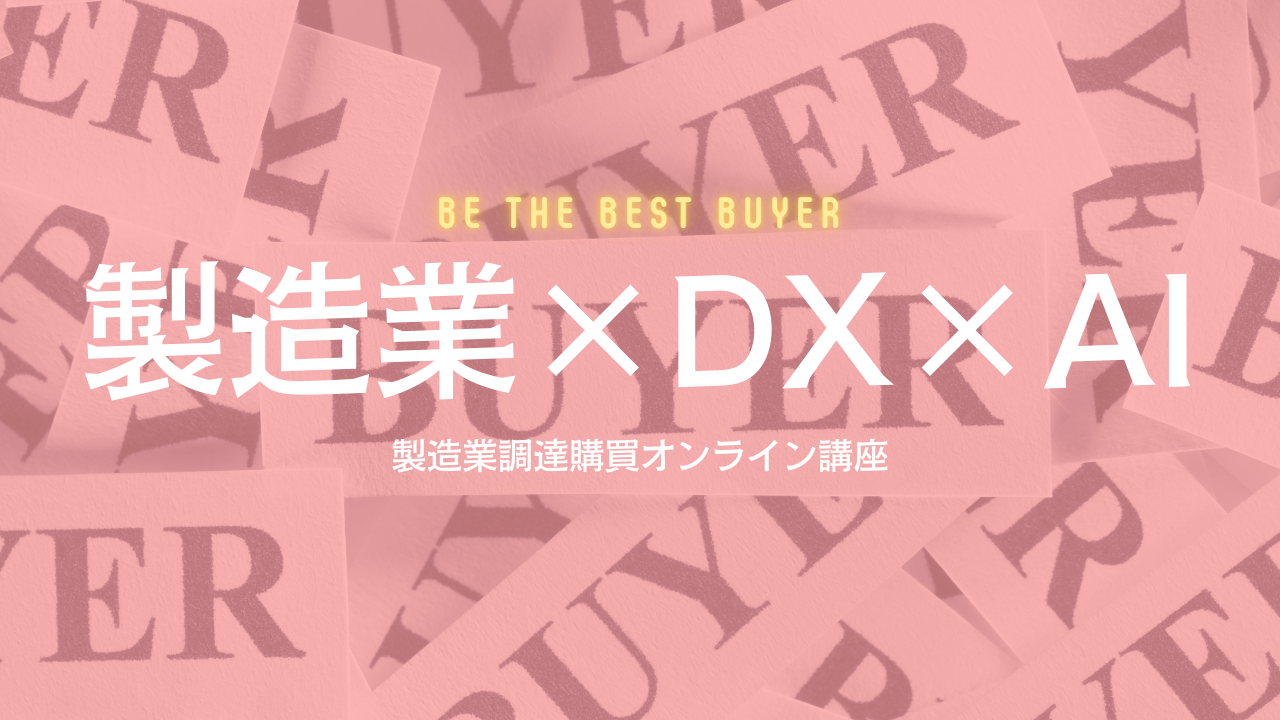
Quality control is crucial for any manufacturing industry in order to ensure customer satisfaction and meet regulations. With advanced technologies available today, quality control processes are becoming more data-driven and automated. Let’s take a look at how companies in various manufacturing sectors are leveraging data from different sources to improve quality inspection and control.
One manufacturer that has successfully implemented data-driven quality control is Anthropic, a company that makes robotic arms. Their robotic arms need to be carefully calibrated and tested during the production process to meet precision and safety standards. Previously, quality inspectors had to manually examine each robotic arm and record test results. This process was time-consuming and prone to human errors.
Anthropic equipped their production line with sensors to collect data on assembly and test parameters. Motors, joints and end-effectors of each robotic arm are put through a set of calibration movements while sensors capture various data points like position, velocity and torque. This large dataset is analyzed using AI models trained on historical performance data. Any deviations from expected parameters are flagged right away to be addressed before the product is shipped.
The AI system was also able to detect subtle defects and anomalies that human inspectors might miss. This has helped Anthropic achieve a 15% reduction in rework. Automating data collection and analysis has sped up quality inspection by 30% without compromising accuracy. Having real-time insights into production has further helped Anthropic optimize their processes.
Sensors and AI are also playing a key role in quality control for food manufacturers. Conagra Foods, a leading packaged food company, uses IoT sensors and predictive defect analysis to enhance food safety across their factories. Each production line at Conagra generates terabytes of sensor and machine data daily.
This data, along with computer vision insights into packaging and labeling, is fed into ML models. These models have been trained on historical defect patterns to identify signs of potential contamination or imperfections early in the process. Any issues detected can be immediately addressed before it impacts food quality or results in a product recall down the line.
Since implementing this IoT and AI quality control solution a few years ago, Conagra has reduced waste and recalls by over 25%. Their predictive system was even able to identify defects that humans inspectors missed, avoiding potential risks to consumers and damage to brand reputation. The automated, data-driven quality control approach has helped Conagra strengthen operational efficiency as well as uphold stringent food safety standards.
Another innovative IoT quality control implementation comes from Ford Motors. Ford assembles hundreds of thousands of vehicles each year across multiple assembly plants globally. Traditionally, the automaker relied on spot-checks and after-production audits to ensure consistent quality.
Ford equipped their assembly lines with thousands of networked sensors to monitor over 4,000 data points per vehicle in real-time – from paint application parameters to tightness of bolts. This wealth of production data flows into Ford’s quality prediction system powered by machine learning. The AI algorithms analyze data patterns from individual vehicles as well as historical trends to predict defects with up 90% accuracy.
Issues can be detected and addressed while the vehicle is still on the assembly line, avoiding the need for repairs down the road. This predictive quality approach has helped Ford reduce defects by 30% and lower rework costs significantly. Continuous monitoring through IoT also allows Ford to proactively spot potential issues and make adjustments before quality is impacted on a large scale.
In summary, leveraging data through technologies like IoT, AI/ML and analytics is enabling major manufacturing industries to take quality control and inspection to the next level. Higher levels of automation, real-time insights and predictive defect analysis are helping companies reduce errors, streamline operations and uphold consistent standards of quality and safety. The data-driven quality approach also makes processes more transparent and optimized. As technologies continue to advance, they will transform quality management across various sectors of manufacturing.
資料ダウンロード
QCD調達購買管理クラウド「newji」は、調達購買部門で必要なQCD管理全てを備えた、現場特化型兼クラウド型の今世紀最高の購買管理システムとなります。
ユーザー登録
調達購買業務の効率化だけでなく、システムを導入することで、コスト削減や製品・資材のステータス可視化のほか、属人化していた購買情報の共有化による内部不正防止や統制にも役立ちます。
NEWJI DX
製造業に特化したデジタルトランスフォーメーション(DX)の実現を目指す請負開発型のコンサルティングサービスです。AI、iPaaS、および先端の技術を駆使して、製造プロセスの効率化、業務効率化、チームワーク強化、コスト削減、品質向上を実現します。このサービスは、製造業の課題を深く理解し、それに対する最適なデジタルソリューションを提供することで、企業が持続的な成長とイノベーションを達成できるようサポートします。
オンライン講座
製造業、主に購買・調達部門にお勤めの方々に向けた情報を配信しております。
新任の方やベテランの方、管理職を対象とした幅広いコンテンツをご用意しております。
お問い合わせ
コストダウンが利益に直結する術だと理解していても、なかなか前に進めることができない状況。そんな時は、newjiのコストダウン自動化機能で大きく利益貢献しよう!
(Β版非公開)