- お役立ち記事
- deep learning
月間76,176名の
製造業ご担当者様が閲覧しています*
*2025年3月31日現在のGoogle Analyticsのデータより
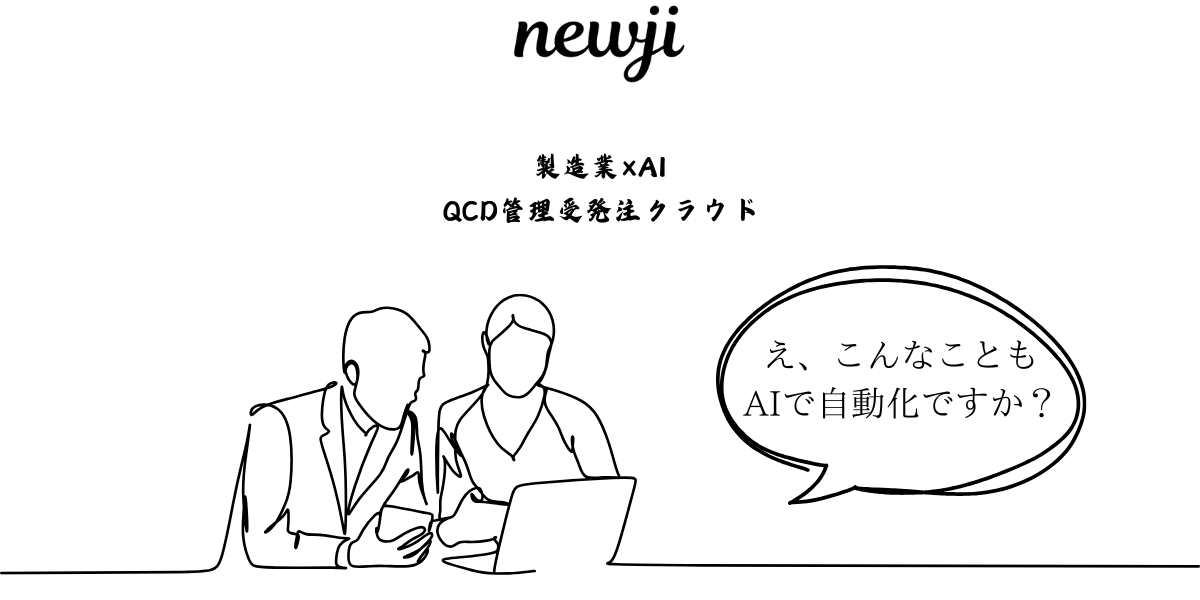
deep learning
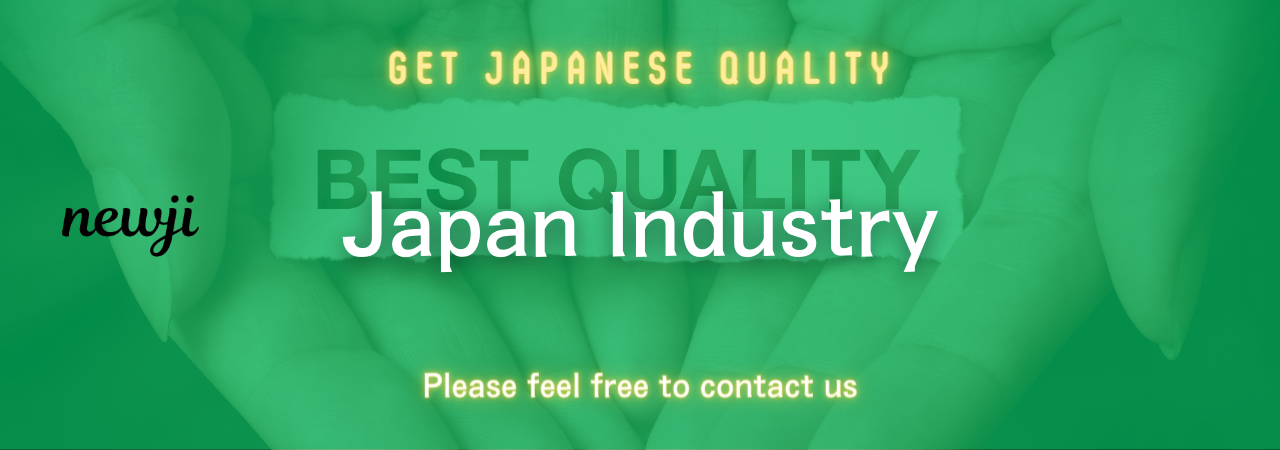
Deep learning is a powerful subset of machine learning that has gained significant attention in recent years due to its impressive ability to process and analyze vast amounts of data.
It is a technology that mimics the way the human brain works, using neural networks to model complex patterns in data.
In this article, we will explore what deep learning is, how it works, its applications, and the future possibilities it holds.
目次
What is Deep Learning?
Deep learning is a type of artificial intelligence (AI) that focuses on employing neural networks with multiple layers to perform complex computations.
Unlike traditional machine learning algorithms, which rely on humans to guide them in feature engineering, deep learning algorithms automatically learn to identify important features directly from the data.
The term “deep” references the depth of layers in the neural networks, which can range from a few to hundreds.
Each layer in a neural network is composed of units called neurons, which are interconnected and work together to process information.
These networks are capable of handling diverse forms of data, such as images, audio, and text, making them incredibly versatile.
How Deep Learning Works
Deep learning requires a large amount of data and computing power to train models effectively.
The process begins with feeding the neural network a dataset, where each layer processes the data, extracting increasingly complex features.
The end goal is for the network to accurately predict or categorize data it has never seen before.
Neural Networks
Neural networks are the backbone of deep learning.
Each neural network consists of layers: an input layer, one or more hidden layers, and an output layer.
The input layer accepts the data inputs.
These inputs are then passed through the hidden layers, where computations are performed using mathematical functions.
The hidden layers learn to extract higher-level features from the raw input data.
Finally, the output layer provides the end predictions.
Training Deep Learning Models
Training a deep learning model involves adjusting the weights of the connections between the neurons.
This is done using a process called backpropagation, which involves calculating the error at the output layer and propagating it back through the network.
The algorithm adjusts the weights in a way that minimizes the error each time the data passes through the network.
An common optimization algorithm used is gradient descent, which aims to find the minimum of the error function.
Applications of Deep Learning
Deep learning has various applications across numerous industries, significantly impacting and transforming how businesses operate and innovate.
Image and Speech Recognition
One of the most well-known applications of deep learning is in image and speech recognition.
Deep learning models power facial recognition systems, enabling technology to accurately identify and authenticate individuals based on facial features.
Similarly, voice assistants like Amazon’s Alexa and Apple’s Siri utilize deep learning for speech recognition, interpreting and responding to voice commands effectively.
Natural Language Processing (NLP)
Natural language processing leverages deep learning to facilitate interactions between humans and machines using natural language.
Applications include language translation services, chatbots, and sentiment analysis tools that analyze customer feedback or social media interactions.
The ability of deep learning models to understand and process language at a high level is continually improving customer experience and operational efficiencies.
Healthcare and Diagnostics
In healthcare, deep learning assists in diagnostics and predictive analytics.
For instance, deep learning algorithms can analyze medical images to detect diseases like cancer with high accuracy.
By interpreting complex patterns in imaging data, these models aid healthcare professionals in early diagnosis and treatment planning.
Furthermore, predictive tools developed through deep learning offer insights into patient health outcomes, helping clinicians make better-informed decisions.
The Future of Deep Learning
As technology advances, deep learning continues to evolve, offering new and exciting possibilities.
Enhancements in Neural Networks
Future developments in neural networks, including more efficient architectures and algorithms, promise to enhance the capability of deep learning systems.
Innovations such as transformer networks and reinforcement learning are paving the way for more sophisticated applications.
Generative Adversarial Networks (GANs), for example, can create highly realistic images and are expanding into fields like content creation and entertainment.
Integration with IoT
Deep learning’s integration with the Internet of Things (IoT) is set to transform industries like manufacturing, agriculture, and smart cities.
IoT devices equipped with deep learning capabilities can process data locally, enabling faster decision-making and reducing the need for cloud-based computational resources.
This integration fosters the development of autonomous systems, enhancing productivity and efficiency.
Ethical Considerations and Challenges
As deep learning continues to advance, ethical considerations surrounding data privacy, bias, and security remain crucial.
Developers must ensure that the algorithms are transparent, fair, and accountable, addressing any biases that might arise from the datasets used.
Striking a balance between innovation and ethical guidelines will be essential for fostering trust in deep learning technologies.
In conclusion, deep learning is a transformative technology that is reshaping the way we approach data processing and analysis.
Its applications span across various industries, enhancing capabilities in image recognition, language processing, and healthcare.
As the field progresses, the potential for deep learning is boundless, holding promise for more intelligent and autonomous systems.
However, it is essential to address the ethical challenges it presents to ensure a responsible and sustainable future for this powerful technology.
資料ダウンロード
QCD管理受発注クラウド「newji」は、受発注部門で必要なQCD管理全てを備えた、現場特化型兼クラウド型の今世紀最高の受発注管理システムとなります。
ユーザー登録
受発注業務の効率化だけでなく、システムを導入することで、コスト削減や製品・資材のステータス可視化のほか、属人化していた受発注情報の共有化による内部不正防止や統制にも役立ちます。
NEWJI DX
製造業に特化したデジタルトランスフォーメーション(DX)の実現を目指す請負開発型のコンサルティングサービスです。AI、iPaaS、および先端の技術を駆使して、製造プロセスの効率化、業務効率化、チームワーク強化、コスト削減、品質向上を実現します。このサービスは、製造業の課題を深く理解し、それに対する最適なデジタルソリューションを提供することで、企業が持続的な成長とイノベーションを達成できるようサポートします。
製造業ニュース解説
製造業、主に購買・調達部門にお勤めの方々に向けた情報を配信しております。
新任の方やベテランの方、管理職を対象とした幅広いコンテンツをご用意しております。
お問い合わせ
コストダウンが利益に直結する術だと理解していても、なかなか前に進めることができない状況。そんな時は、newjiのコストダウン自動化機能で大きく利益貢献しよう!
(β版非公開)