- お役立ち記事
- Deep learning utilization and optimization of optical measurement data analysis
Deep learning utilization and optimization of optical measurement data analysis
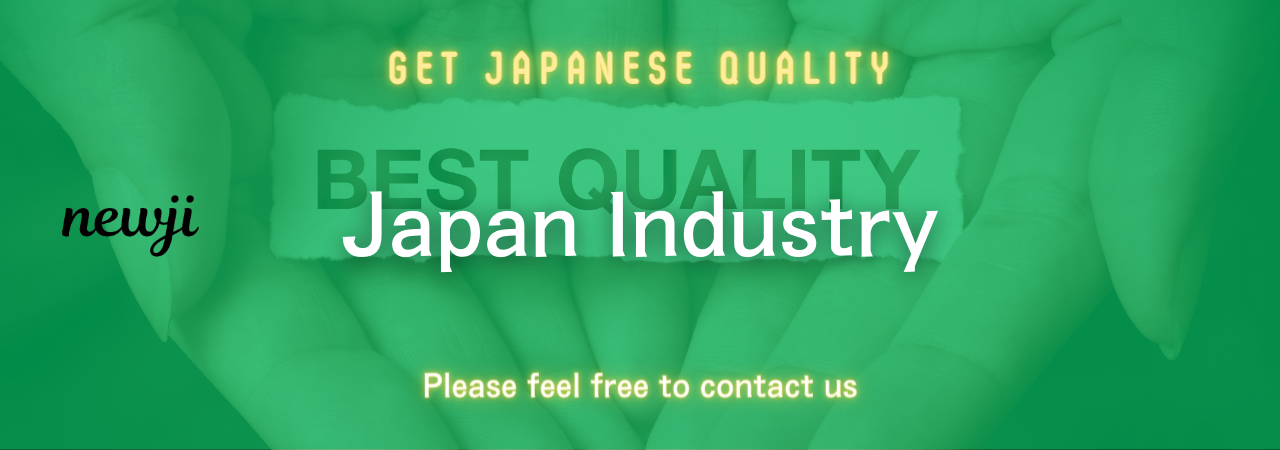
目次
Understanding Optical Measurement Data
Optical measurement is a fundamental technique used in various fields such as manufacturing, engineering, and healthcare for accurate data collection and analysis.
By using light-based tools and instruments, optical measurement ensures high precision and reliability when capturing data about materials, devices, and biological samples.
As technology advances, the accumulation of massive optical measurement data requires efficient analysis techniques to extract valuable insights.
The Role of Deep Learning in Data Analysis
Deep learning, a subset of machine learning, has emerged as a cutting-edge tool in handling large datasets due to its ability to analyze complex patterns and relationships within the data.
Characterized by neural networks with multiple layers, deep learning efficiently processes massive amounts of data, making it ideal for analyzing optical measurement data.
One key advantage of deep learning is its impressive capability to automate complex decision-making processes.
By learning from vast datasets, deep learning models can effectively recognize patterns and detect anomalies that may not be evident to human analysts.
This results in more accurate and faster discoveries, improving the efficiency of optical measurement data analysis.
Applying Deep Learning to Optical Measurement
The incorporation of deep learning in optical measurement data analysis has sparked transformative changes across various industries.
Enhancing Precision in Manufacturing
In the manufacturing industry, precise optical measurement is crucial for quality control and process optimization.
By integrating deep learning, manufacturers can enhance the accuracy of defect detection and optimize production processes.
Deep learning algorithms can be programmed to monitor real-time production line data, quickly flagging any discrepancies that may impact product quality.
The adaptability of neural networks means models can continually learn from new data, refining their predictions over time for superior outcomes.
Advancing Medical Imaging
In the medical field, optical measurements are crucial for diagnostic imaging techniques like MRI, CT scans, and X-rays.
Deep learning has revolutionized medical imaging, enabling more refined image analysis for accurate diagnosis.
For example, convolutional neural networks (CNNs) are particularly adept at identifying disease patterns and segmenting anatomical structures within medical images.
The ability of deep learning to interpret complex visual data greatly aids radiologists in making informed clinical decisions, delivering targeted treatments based on precise diagnoses.
Improving Environmental Monitoring
Environmental scientists rely heavily on optical measurement for monitoring various ecological parameters, such as air quality, water quality, and climate changes.
Deep learning models can provide detailed analyses of environmental data, allowing researchers to detect subtle changes imperceptible through traditional methods.
Through image recognition and data patterning, deep learning can forecast trends and potential environmental impacts, supporting proactive measures for sustainable resource management.
Strategies for Optimization
While deep learning offers substantial opportunities for optical measurement data analysis, optimization strategies are necessary for maximizing performance and efficiency.
Data Preprocessing and Quality Control
Quality data is critical for training effective deep learning models.
Preprocessing techniques, including noise filtering, normalization, and data augmentation, ensure the dataset is clean and relevant.
By enhancing data quality, models are equipped with reliable inputs, facilitating accurate predictions and analyses.
Hyperparameter Tuning
Hyperparameters are crucial settings within machine learning models that significantly influence their learning process and final outcome.
Tuning hyperparameters carefully can notably optimize deep learning performance.
This involves adjusting learning rates, batch sizes, and activation functions to achieve optimal results for specific optical measurement tasks.
Automated tools, like grid search or Bayesian optimization, can assist in identifying the most suitable configuration for effective model training.
Model Regularization
Regularization techniques help prevent overfitting, a common challenge in deep learning where models become too complex and fail to generalize to new data.
Regularization adds constraints to the model during training to enhance its ability to generalize from the training set.
Techniques, such as dropout and weight decay, are implemented in optical measurement data analysis to achieve robust and scalable neural networks.
Transfer Learning
Transfer learning harnesses pre-trained models on large datasets, adapting them for related tasks with smaller, domain-specific datasets.
This method saves time and computational resources, as the model benefits from pre-existing knowledge and requires less data for fine-tuning.
For optical measurement analysis, transfer learning valuable reduces training time and ensures effective performance even with limited data.
Conclusion
The power of deep learning in analyzing optical measurement data is undeniable.
It has paved the way for unprecedented precision, efficiency, and decision-making across multiple sectors, such as manufacturing, healthcare, and environmental science.
By applying strategic optimization techniques like data preprocessing, hyperparameter tuning, and transfer learning, the utilization of deep learning in optical measurement can be further enhanced.
As technology continues to evolve, it is pivotal that industries capitalize on deep learning to unlock the full potential of their optical measurement data, leading to improved outcomes and innovative solutions.
資料ダウンロード
QCD調達購買管理クラウド「newji」は、調達購買部門で必要なQCD管理全てを備えた、現場特化型兼クラウド型の今世紀最高の購買管理システムとなります。
ユーザー登録
調達購買業務の効率化だけでなく、システムを導入することで、コスト削減や製品・資材のステータス可視化のほか、属人化していた購買情報の共有化による内部不正防止や統制にも役立ちます。
NEWJI DX
製造業に特化したデジタルトランスフォーメーション(DX)の実現を目指す請負開発型のコンサルティングサービスです。AI、iPaaS、および先端の技術を駆使して、製造プロセスの効率化、業務効率化、チームワーク強化、コスト削減、品質向上を実現します。このサービスは、製造業の課題を深く理解し、それに対する最適なデジタルソリューションを提供することで、企業が持続的な成長とイノベーションを達成できるようサポートします。
オンライン講座
製造業、主に購買・調達部門にお勤めの方々に向けた情報を配信しております。
新任の方やベテランの方、管理職を対象とした幅広いコンテンツをご用意しております。
お問い合わせ
コストダウンが利益に直結する術だと理解していても、なかなか前に進めることができない状況。そんな時は、newjiのコストダウン自動化機能で大きく利益貢献しよう!
(Β版非公開)