- お役立ち記事
- Demonstration of fracture surface analysis method using machine learning
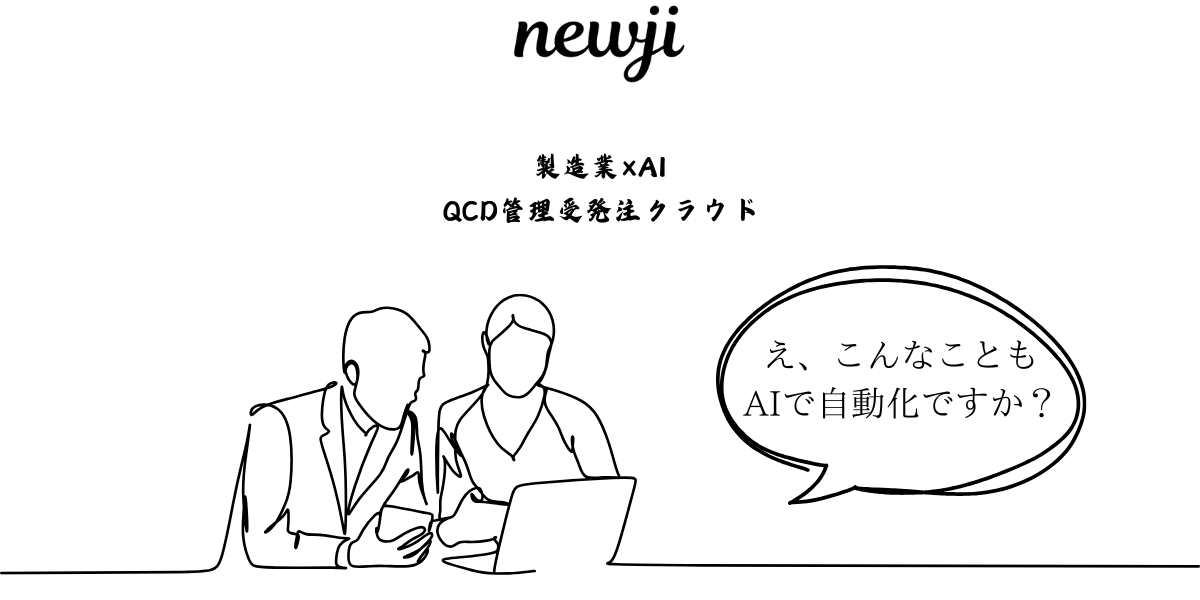
Demonstration of fracture surface analysis method using machine learning
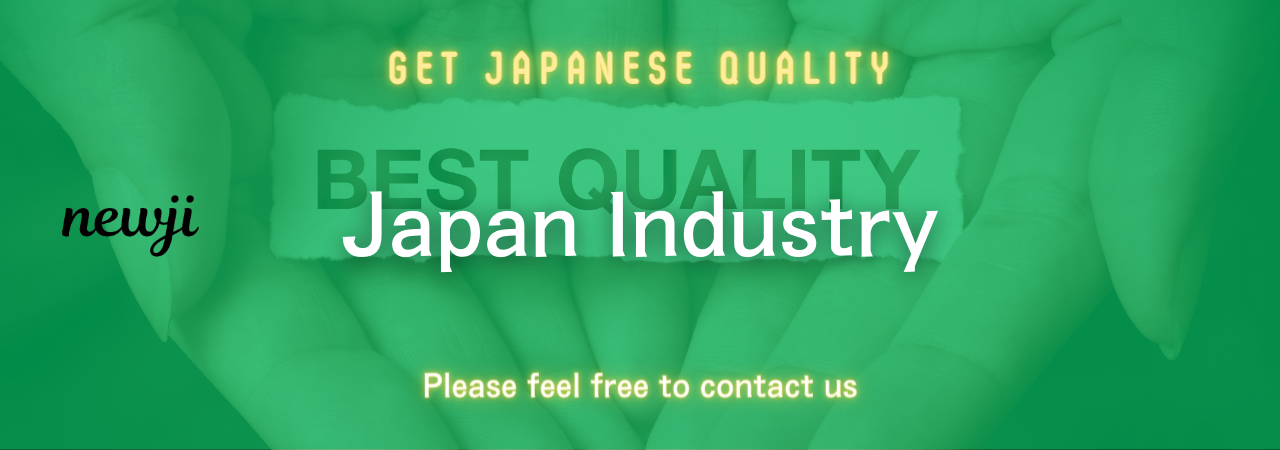
目次
Understanding Fracture Surface Analysis
Fracture surface analysis is a fascinating field that plays a crucial role in understanding how and why materials break.
By examining the patterns and features on broken surfaces, scientists and engineers can infer valuable information about the fracture process and the mechanical properties of materials.
This knowledge is pivotal in designing safer and more resilient structures, from bridges and buildings to aircraft and automotive components.
Traditional fracture surface analysis involves meticulous examination using techniques like scanning electron microscopy (SEM) or atomic force microscopy (AFM).
These methods provide detailed images of fracture surfaces, allowing experts to identify features such as striations, ridges, and dimple patterns.
However, the interpretation of these images is often subjective, relying heavily on the expertise and experience of the analyst.
In recent years, machine learning has emerged as a powerful tool to complement and enhance traditional fracture surface analysis.
By harnessing the power of algorithms, machine learning can automate feature extraction and classification, offering a more objective and efficient approach to fracture surface analysis.
Machine Learning and Fracture Surface Analysis
Machine learning involves training algorithms on large sets of data to recognize patterns and make predictions.
In the context of fracture surface analysis, machine learning models can be trained on a wide variety of fracture surface images.
These models learn to identify key features associated with different types of fractures, such as ductile, brittle, or fatigue fractures.
The integration of machine learning into fracture surface analysis involves several steps.
First, a comprehensive dataset of fracture surface images needs to be compiled.
This dataset should include images of fractures from different materials and under various loading conditions, ensuring the model can generalize well.
Once the dataset is ready, the next step is to preprocess the images.
This involves enhancing the image quality and performing tasks like noise reduction and edge detection.
These steps help ensure the machine learning model receives clear and relevant information from the input data.
Feature Extraction and Model Training
Feature extraction is a critical step in the machine learning process.
In fracture surface analysis, this step involves identifying and quantifying specific patterns and features from the images.
These could include textural properties, lines, curves, and other geometric attributes that characterize the fracture surface.
Various techniques can be used for feature extraction, ranging from basic methods like histogram analysis to more sophisticated approaches like convolutional neural networks (CNNs).
CNNs, in particular, have proven highly effective in image analysis tasks due to their ability to automatically learn complex patterns from raw images.
After feature extraction, the next stage is model training.
During this phase, the extracted features are used to train a machine learning model.
The model learns to associate certain patterns with specific types of fractures.
Training involves adjusting the model parameters iteratively until it can accurately classify fracture surfaces based on the learned features.
Evaluation and Validation
Once the model is trained, it needs to be evaluated to ensure its reliability and accuracy.
This evaluation phase typically involves testing the model on a separate set of fracture surface images that were not used during training.
The model’s predictions are compared against the known classifications to assess its performance.
Several metrics can be used to evaluate a machine learning model, including accuracy, precision, recall, and F1-score.
These metrics provide insights into how well the model is performing and where improvements might be needed.
If the model demonstrates satisfactory performance, it can be validated further through blind testing or real-world applications.
This step ensures the model’s robustness and adaptability when applied to new and unseen data.
Benefits and Challenges
The application of machine learning in fracture surface analysis offers numerous benefits.
It allows for rapid and consistent analysis of fracture surfaces, reducing the need for subjective interpretation.
This automation leads to increased efficiency, enabling experts to focus on more complex tasks and decision-making.
Additionally, machine learning models have the potential to uncover patterns or correlations that might escape traditional analysis.
By processing vast amounts of data, these models can identify subtle features that could provide new insights into fracture mechanisms.
However, integrating machine learning into fracture surface analysis also presents challenges.
One significant challenge is the need for extensive and high-quality datasets for training.
Collecting and curating such datasets can be time-consuming and resource-intensive.
Another challenge is ensuring the model remains interpretable and explainable.
While machine learning models can be highly accurate, their decision-making process is often a “black box.”
Researchers must find ways to interpret the model’s predictions and understand which features it considers most significant.
The Future of Fracture Surface Analysis
As machine learning technology continues to advance, its role in fracture surface analysis is likely to grow.
Researchers are exploring the integration of machine learning with other emerging technologies, such as augmented reality and virtual reality, to create more interactive and informative analysis tools.
Moreover, ongoing advancements in machine learning algorithms and hardware will enable more complex and sophisticated analyses, unlocking new possibilities in materials science and engineering.
In conclusion, the demonstration of fracture surface analysis using machine learning represents a significant step forward in the field.
By combining traditional techniques with modern technology, scientists and engineers can achieve a deeper understanding of material behavior, leading to safer and more reliable products and structures.
This synergy between technology and traditional knowledge exemplifies the exciting future of fracture surface analysis and materials science.
資料ダウンロード
QCD調達購買管理クラウド「newji」は、調達購買部門で必要なQCD管理全てを備えた、現場特化型兼クラウド型の今世紀最高の購買管理システムとなります。
ユーザー登録
調達購買業務の効率化だけでなく、システムを導入することで、コスト削減や製品・資材のステータス可視化のほか、属人化していた購買情報の共有化による内部不正防止や統制にも役立ちます。
NEWJI DX
製造業に特化したデジタルトランスフォーメーション(DX)の実現を目指す請負開発型のコンサルティングサービスです。AI、iPaaS、および先端の技術を駆使して、製造プロセスの効率化、業務効率化、チームワーク強化、コスト削減、品質向上を実現します。このサービスは、製造業の課題を深く理解し、それに対する最適なデジタルソリューションを提供することで、企業が持続的な成長とイノベーションを達成できるようサポートします。
オンライン講座
製造業、主に購買・調達部門にお勤めの方々に向けた情報を配信しております。
新任の方やベテランの方、管理職を対象とした幅広いコンテンツをご用意しております。
お問い合わせ
コストダウンが利益に直結する術だと理解していても、なかなか前に進めることができない状況。そんな時は、newjiのコストダウン自動化機能で大きく利益貢献しよう!
(Β版非公開)