- お役立ち記事
- Development and Utilization of Data Analytics Talent in Quality Management for Manufacturing
月間76,176名の
製造業ご担当者様が閲覧しています*
*2025年3月31日現在のGoogle Analyticsのデータより
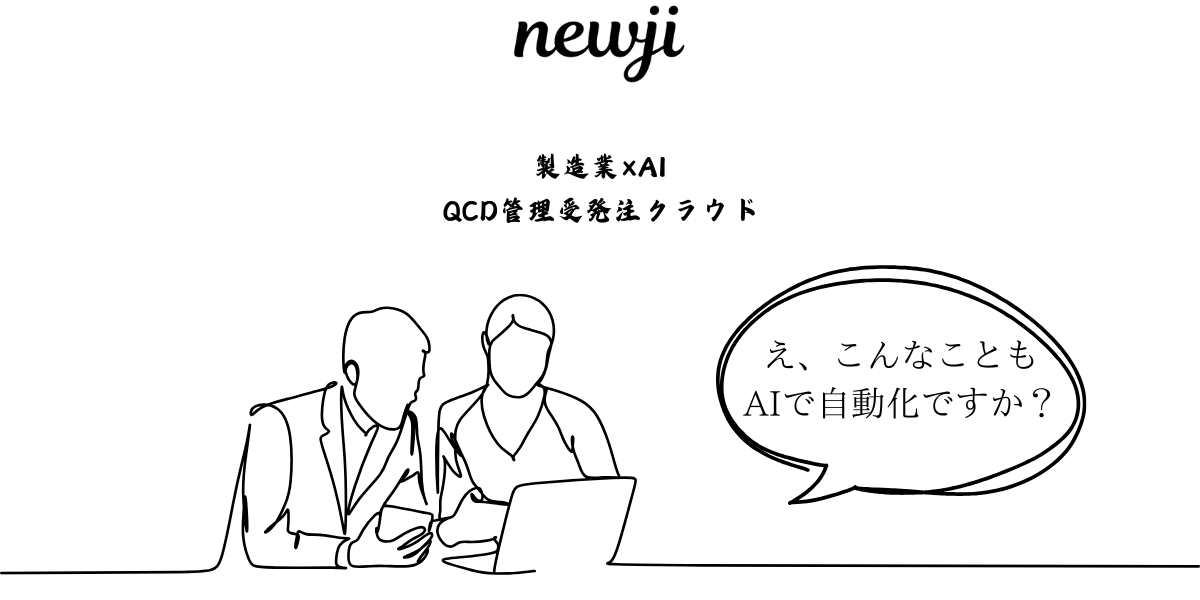
Development and Utilization of Data Analytics Talent in Quality Management for Manufacturing
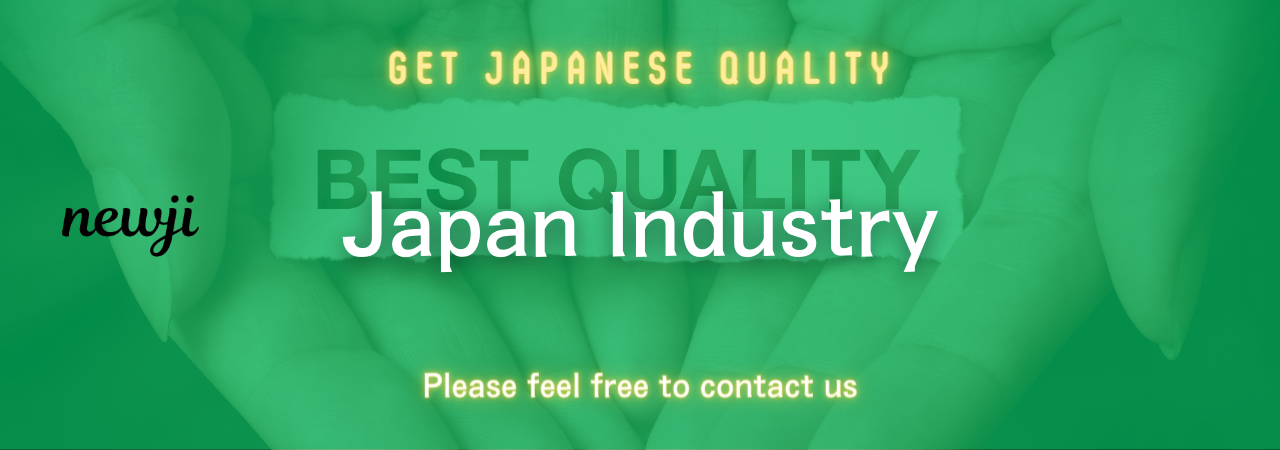
In the rapidly evolving manufacturing sector, quality management remains a cornerstone for industry success.
Traditionally, manufacturers relied on manual inspection and basic statistical methods to ensure product quality.
However, with the advent of data analytics, there’s a new paradigm in quality management, demanding the development and utilization of data analytics talent.
This comprehensive blog aims to explore the importance of data analytics in quality management and how organizations can foster and capitalize on such talent effectively.
目次
The Role of Data Analytics in Quality Management
Data analytics has transformed many industries, and manufacturing is no exception.
When applied to quality management, data analytics can provide insights that were previously impossible to obtain.
It enables manufacturers to predict defects, optimize processes, and make data-driven decisions that enhance product quality.
Predictive Analysis
Predictive analytics uses historical data to predict future outcomes.
In manufacturing, it can anticipate potential defects before they occur.
For instance, sensors on assembly lines can collect data that, when analyzed, indicate signs of potential failures.
This enables corrective actions before any defective products are produced and shipped.
Process Optimization
Process optimization involves fine-tuning production processes to ensure maximum efficiency without compromising quality.
Data analytics can identify bottlenecks, inefficiencies, and variations in the production process.
These insights allow manufacturers to streamline operations, reducing waste and enhancing overall product quality.
Real-Time Monitoring
Real-time monitoring powered by data analytics provides continuous insights into production lines.
It allows managers to swiftly identify and address issues before they escalate.
This continuous monitoring ensures that the manufacturing process adheres to quality standards at all times.
Building a Data-Driven Quality Management Team
Despite the evident benefits, integrating data analytics into quality management is challenging without the right talent.
Organizations need to invest in developing a team skilled in both manufacturing and data analytics.
Identifying Key Skills
Manufacturers should identify the key skills necessary for data analytics in quality management.
These include statistical analysis, machine learning, data mining, and industry-specific knowledge.
A blend of these skills is crucial for effective data-driven quality management.
Training and Development Programs
Organizations must invest in continuous training and development programs.
This helps existing employees upgrade their skills and keeps pace with technological advancements.
Collaborating with educational institutions to create specialized programs can also be beneficial.
Hiring Specialized Talent
Sometimes, training existing employees might not be enough.
Hiring specialized talent with a background in data analytics and manufacturing can fill the gap.
Offering competitive salaries and growth opportunities can attract the right talent.
Utilizing Data Analytics in Quality Management
Once the talent is in place, the next step is to effectively utilize data analytics for quality management.
This involves several strategic approaches.
Integrating Data Sources
Quality management data can come from various sources, such as production lines, supply chain systems, and customer feedback.
Integrating these data sources into a centralized system allows for comprehensive analysis.
This unified approach provides a holistic view of quality across the entire manufacturing process.
Implementing Advanced Tools
Leveraging advanced tools and technologies is crucial for effective data analytics.
Tools such as machine learning algorithms, artificial intelligence, and data visualization software can provide deeper insights.
Implementing these tools can revolutionize quality management practices.
Encouraging a Data-Driven Culture
Creating a culture that values data-driven decision-making is crucial.
From top management to shop floor workers, everyone should understand the importance of data analytics.
Conducting workshops, seminars, and regular training can foster this culture.
The Challenges and How to Overcome Them
Despite the advantages, integrating data analytics into quality management poses challenges.
Recognition of these challenges and planning to overcome them is essential for success.
Data Quality and Management
The quality of insights derived from analytics depends on data quality.
Incomplete, inaccurate, or outdated data can lead to erroneous conclusions.
Establishing robust data governance frameworks ensures high data quality and reliability.
Resistance to Change
Introducing new technologies often meets resistance from employees accustomed to traditional methods.
Communicating the benefits of data analytics and providing adequate training can mitigate resistance.
Involving employees in the transition process fosters ownership and eases the adaptation.
Cost Implications
Implementing data analytics can be financially demanding.
The cost includes not only tools and technologies but also training and hiring skilled professionals.
However, the long-term benefits, such as reduced waste, increased efficiency, and enhanced product quality, often outweigh the initial investment.
Case Studies and Success Stories
To better understand the impact of data analytics in quality management, it’s useful to look at successful implementations.
Case Study: Automotive Industry
An automotive manufacturer implemented machine learning algorithms in its quality management system.
By analyzing production data, the system could predict potential defects up to 24 hours before they occurred.
This proactive approach reduced defects by 20%, leading to significant cost savings and improved customer satisfaction.
Case Study: Electronics Manufacturing
An electronics manufacturer used data analytics for real-time monitoring and process optimization.
By analyzing data from IoT sensors on the production line, the company significantly improved yield rates.
The data-driven insights allowed for immediate corrective actions, ensuring high-quality output.
In conclusion, the development and utilization of data analytics talent are crucial for the future of quality management in manufacturing.
Organizations must focus on building skilled teams, leveraging advanced tools, and fostering a data-driven culture.
Overcoming the challenges and effectively integrating data analytics can lead to unparalleled improvements in product quality and operational efficiency.
As the manufacturing industry continues to evolve, embracing data analytics is no longer an option but a necessity for sustaining competitiveness and delivering superior products.
資料ダウンロード
QCD管理受発注クラウド「newji」は、受発注部門で必要なQCD管理全てを備えた、現場特化型兼クラウド型の今世紀最高の受発注管理システムとなります。
ユーザー登録
受発注業務の効率化だけでなく、システムを導入することで、コスト削減や製品・資材のステータス可視化のほか、属人化していた受発注情報の共有化による内部不正防止や統制にも役立ちます。
NEWJI DX
製造業に特化したデジタルトランスフォーメーション(DX)の実現を目指す請負開発型のコンサルティングサービスです。AI、iPaaS、および先端の技術を駆使して、製造プロセスの効率化、業務効率化、チームワーク強化、コスト削減、品質向上を実現します。このサービスは、製造業の課題を深く理解し、それに対する最適なデジタルソリューションを提供することで、企業が持続的な成長とイノベーションを達成できるようサポートします。
製造業ニュース解説
製造業、主に購買・調達部門にお勤めの方々に向けた情報を配信しております。
新任の方やベテランの方、管理職を対象とした幅広いコンテンツをご用意しております。
お問い合わせ
コストダウンが利益に直結する術だと理解していても、なかなか前に進めることができない状況。そんな時は、newjiのコストダウン自動化機能で大きく利益貢献しよう!
(β版非公開)