- お役立ち記事
- Efficient controller design method realized by data-driven control
Efficient controller design method realized by data-driven control
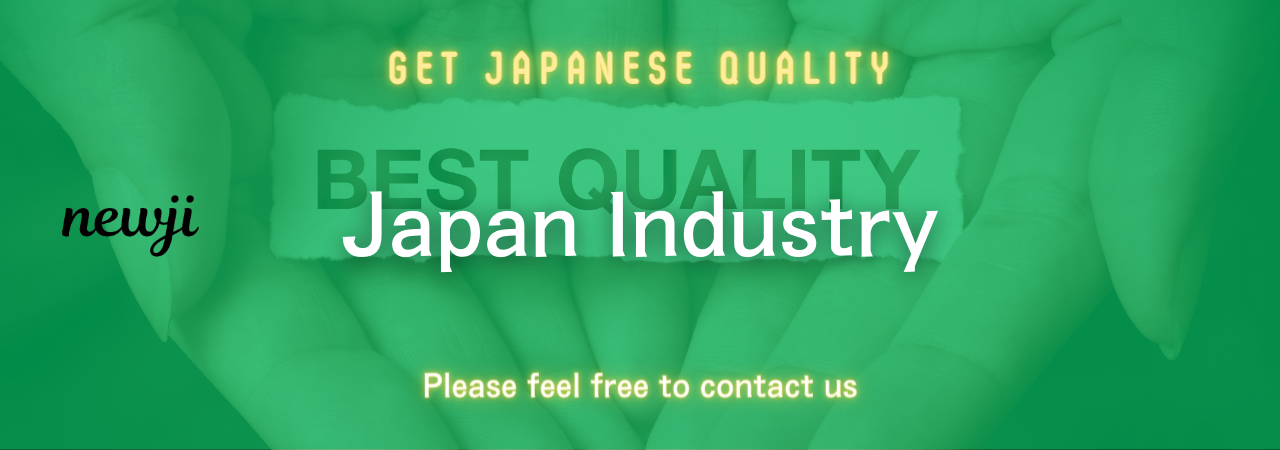
目次
Introduction to Data-Driven Control
In the world of control systems, traditional methods often rely heavily on mathematical models.
These models are derived from first principles or complex simulations.
However, in many cases, obtaining an accurate model of a system can be challenging and time-consuming.
This is where data-driven control comes into play.
Data-driven control uses real-world data to design control systems, making it an efficient alternative to the traditional model-based approaches.
By leveraging data, engineers can create accurate, responsive control systems without the extensive modeling effort.
What is Data-Driven Control?
Data-driven control is a methodology that focuses on using empirical data to design and optimize control systems.
Instead of relying on theoretical models and assumptions, data-driven control techniques work directly with the data collected from the actual system.
This allows for designing controllers that are more robust and adaptive to changes in the system behavior.
The core idea is to minimize the system control error using historical operational data rather than an estimated model.
Controllers can be fine-tuned and adjusted as new data becomes available, making them flexible and less sensitive to inaccuracies in theoretical modeling.
Benefits of Data-Driven Control
One of the main advantages of using data-driven control methods is efficiency.
Since these methods do not rely on extensive modeling, much of the time and effort spent on developing accurate system models is saved.
This allows engineers to focus on improving system performance and achieving desired outcomes more quickly.
Another benefit is adaptability.
Data-driven controllers can be modified easily as more data is collected, allowing for continuous optimization.
This feature is particularly useful in systems where conditions frequently change, which can often lead to outdated models in traditional control scenarios.
Furthermore, data-driven control can often provide a better fit for complex or nonlinear systems, where traditional models struggle.
The empirical approach allows for direct tuning based on actual system behavior, potentially improving control accuracy and overall system performance.
Applications of Data-Driven Control
Data-driven control techniques have a wide range of applications across various industries.
In manufacturing, they can optimize processes for efficiency and quality by adjusting system parameters in real-time.
Automation in supply chains can also greatly benefit from these methods, leading to more responsive and agile operations.
In the automotive sector, data-driven control is helping to advance autonomous vehicle technologies.
By analyzing data from numerous sensors and control units, these systems can make intelligent decisions that improve safety and performance.
The energy sector also benefits, particularly in managing complex power grids and renewable energy sources.
By using data-driven control, these systems can better adapt to fluctuating power supply and demand, improving stability and efficiency.
How to Implement Data-Driven Control
Implementing a data-driven control system involves several key steps.
First and foremost, collecting high-quality data is crucial.
The accuracy and effectiveness of the control strategy rely heavily on the quality of the data collected from the system.
Next, engineers must select appropriate data-driven techniques to analyze the data.
Techniques such as machine learning, statistical analysis, and optimization algorithms are employed to extract meaningful insights and patterns from the data.
Once analysis is complete, the insights can inform the design of the control system.
This typically involves tuning the parameters of the control laws to achieve desired system behaviors.
Finally, continuous monitoring and adaptation are crucial.
A data-driven control system should be continuously updated and refined as new data streams in, ensuring optimal performance over time.
Challenges in Data-Driven Control
Despite the benefits, data-driven control does come with challenges.
One of the biggest challenges is ensuring the quality of the data.
Noisy or incomplete data can lead to inaccurate insights, undermining the efficacy of the controller.
Additionally, there can be computational challenges, especially with large datasets.
The need for powerful computing resources to process and analyze vast amounts of data is essential.
Moreover, there can be concerns about interpretability and transparency.
Data-driven models, especially those utilizing complex algorithms like neural networks, may not be easily understandable, which can be a barrier in critical applications where understanding the control decision-making process is necessary.
Conclusion
Data-driven control represents a significant shift in the way control systems are designed and implemented.
With its emphasis on real-world data and its ability to adapt and improve over time, it offers a promising alternative to traditional model-based approaches.
By embracing this methodology, engineers can enhance system performance, reduce development time, and create more robust, responsive control systems.
As industries continue to evolve, and data becomes increasingly abundant, the role of data-driven control will likely expand, offering new possibilities and efficiencies in the realm of automated systems.
資料ダウンロード
QCD調達購買管理クラウド「newji」は、調達購買部門で必要なQCD管理全てを備えた、現場特化型兼クラウド型の今世紀最高の購買管理システムとなります。
ユーザー登録
調達購買業務の効率化だけでなく、システムを導入することで、コスト削減や製品・資材のステータス可視化のほか、属人化していた購買情報の共有化による内部不正防止や統制にも役立ちます。
NEWJI DX
製造業に特化したデジタルトランスフォーメーション(DX)の実現を目指す請負開発型のコンサルティングサービスです。AI、iPaaS、および先端の技術を駆使して、製造プロセスの効率化、業務効率化、チームワーク強化、コスト削減、品質向上を実現します。このサービスは、製造業の課題を深く理解し、それに対する最適なデジタルソリューションを提供することで、企業が持続的な成長とイノベーションを達成できるようサポートします。
オンライン講座
製造業、主に購買・調達部門にお勤めの方々に向けた情報を配信しております。
新任の方やベテランの方、管理職を対象とした幅広いコンテンツをご用意しております。
お問い合わせ
コストダウンが利益に直結する術だと理解していても、なかなか前に進めることができない状況。そんな時は、newjiのコストダウン自動化機能で大きく利益貢献しよう!
(Β版非公開)