- お役立ち記事
- Elucidation of main factors through factor analysis
Elucidation of main factors through factor analysis
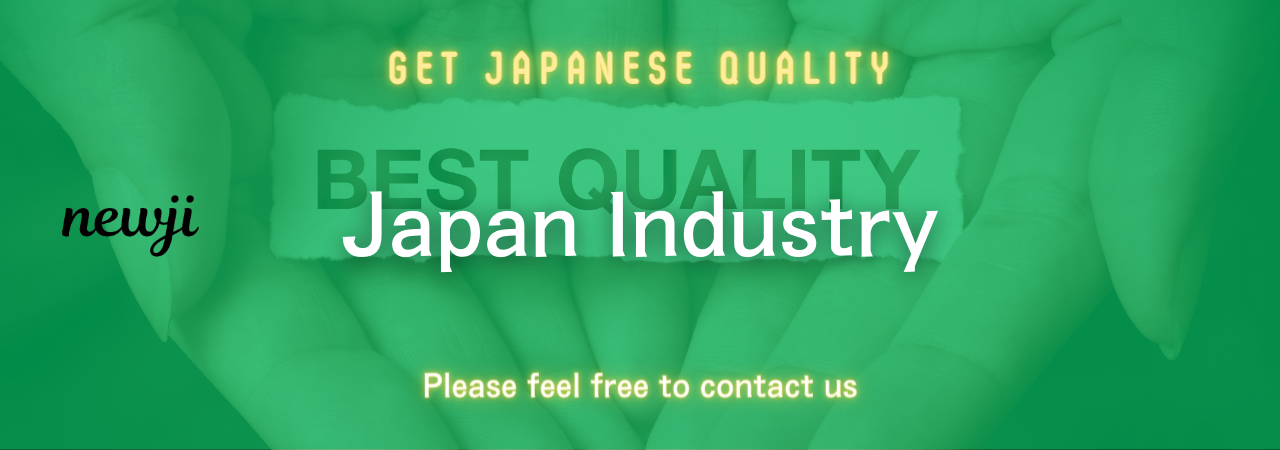
目次
Understanding Factor Analysis
Factor analysis is a powerful statistical technique used to identify the underlying relationships between various variables in a dataset.
It essentially aims to uncover the main factors that explain the patterns observed among the variables.
By reducing the number of variables and identifying the core components, factor analysis provides insight into the data structure.
The technique is widely used across different fields such as psychology, marketing, finance, and social sciences.
It helps researchers and analysts to simplify complex data, making it easier to interpret and understand.
Factor analysis is particularly useful when dealing with large datasets with numerous variables, allowing for a more efficient analysis of the underlying patterns.
Types of Factor Analysis
Before diving deeper, it’s important to differentiate between the two main types of factor analysis: Exploratory Factor Analysis (EFA) and Confirmatory Factor Analysis (CFA).
Exploratory Factor Analysis (EFA)
Exploratory Factor Analysis is used when the researcher does not have a preconceived idea about the structure or number of factors within the dataset.
EFA is an initial step to explore the data and generate hypotheses.
This type of factor analysis helps in understanding how different variables cluster together and suggest potential factors.
Confirmatory Factor Analysis (CFA)
On the other hand, Confirmatory Factor Analysis is used when the researcher has a clear hypothesis about the number of factors and the loadings of observed variables on these factors.
CFA is used to test whether the data fits a hypothesized measurement model.
It requires a more structured approach, often used to validate theories or constructs that have already been identified through EFA or previous research.
The Process of Factor Analysis
Factor analysis follows a systematic process that includes several steps to ensure accurate and meaningful results.
Data Collection and Preparation
The first step involves collecting relevant data and preparing it for analysis.
The data should be quantitative and measured on an interval or ratio scale.
It’s essential to ensure that the dataset has sufficient sample size to produce reliable results.
Choosing the Right Variables
The next step is to carefully choose the variables that will be included in the analysis.
Variables should be relevant to the research question and represent different aspects of the phenomenon being studied.
Correlation Matrix Calculation
Creating a correlation matrix is a crucial step in factor analysis.
This matrix shows the relationships between the variables and helps in identifying those that are strongly correlated, which could potentially form factors.
Extracting Factors
Factor extraction involves determining the number of factors that best represent the dataset.
Two common methods for factor extraction are the Principal Component Analysis (PCA) and the Maximum Likelihood method.
These methods analyze the correlation matrix and identify factors that account for as much variance as possible.
Factor Rotation
Once the factors are extracted, factor rotation techniques are applied to make the output more interpretable.
Varimax rotation, for example, is often used to simplify the factor structure by making the loadings of variables on factors more distinct.
Interpreting Results
The final step involves interpreting the results and understanding the meaning of each factor.
Factor loadings indicate how strongly each variable is associated with a factor.
Researchers examine these loadings to label the factors and gain insight into what each factor represents.
Applications of Factor Analysis
Factor analysis has a wide range of applications across different fields:
Psychology
In psychology, factor analysis is used to identify underlying traits or dimensions of personality, intelligence, or behavior.
It helps in developing psychological tests and exploring the structure of mental abilities.
Marketing
In marketing, businesses use factor analysis to segment customers based on purchasing behaviors or preferences.
It aids in identifying target markets and developing marketing strategies that resonate with specific consumer groups.
Finance
In finance, factor analysis is used to manage investment portfolios and identify factors that affect stock returns.
Investors can make informed decisions by understanding the common forces driving asset prices.
Social Sciences
Social scientists use factor analysis to study complex social phenomena like political attitudes or cultural values.
It helps in identifying key themes and patterns in survey data or social indicators.
Potential Pitfalls and Challenges
While factor analysis offers significant advantages, it also presents potential pitfalls and challenges that analysts need to be aware of:
Sample Size
A common challenge in factor analysis is the need for a sufficiently large sample size.
Small samples can lead to unstable and unreliable factor solutions.
Subjectivity
Interpreting factors requires a certain level of subjectivity.
Different researchers may arrive at different conclusions based on the same factor loadings.
Complex Datasets
In complex datasets with high multicollinearity, factor analysis may produce factors that are difficult to interpret.
Ensuring a clear understanding of the data structure is crucial.
Conclusion
Factor analysis is a vital tool for uncovering the main factors that explain the relationships among variables.
By simplifying complex datasets, it allows for a more comprehensive understanding of the data.
However, it requires careful consideration of the process, challenges, and potential biases.
Employing factor analysis appropriately can provide valuable insights that drive informed decision-making and research exploration across various fields.
資料ダウンロード
QCD調達購買管理クラウド「newji」は、調達購買部門で必要なQCD管理全てを備えた、現場特化型兼クラウド型の今世紀最高の購買管理システムとなります。
ユーザー登録
調達購買業務の効率化だけでなく、システムを導入することで、コスト削減や製品・資材のステータス可視化のほか、属人化していた購買情報の共有化による内部不正防止や統制にも役立ちます。
NEWJI DX
製造業に特化したデジタルトランスフォーメーション(DX)の実現を目指す請負開発型のコンサルティングサービスです。AI、iPaaS、および先端の技術を駆使して、製造プロセスの効率化、業務効率化、チームワーク強化、コスト削減、品質向上を実現します。このサービスは、製造業の課題を深く理解し、それに対する最適なデジタルソリューションを提供することで、企業が持続的な成長とイノベーションを達成できるようサポートします。
オンライン講座
製造業、主に購買・調達部門にお勤めの方々に向けた情報を配信しております。
新任の方やベテランの方、管理職を対象とした幅広いコンテンツをご用意しております。
お問い合わせ
コストダウンが利益に直結する術だと理解していても、なかなか前に進めることができない状況。そんな時は、newjiのコストダウン自動化機能で大きく利益貢献しよう!
(Β版非公開)