- お役立ち記事
- Enhancing Object Detection with Deep Learning for Real-World Applications
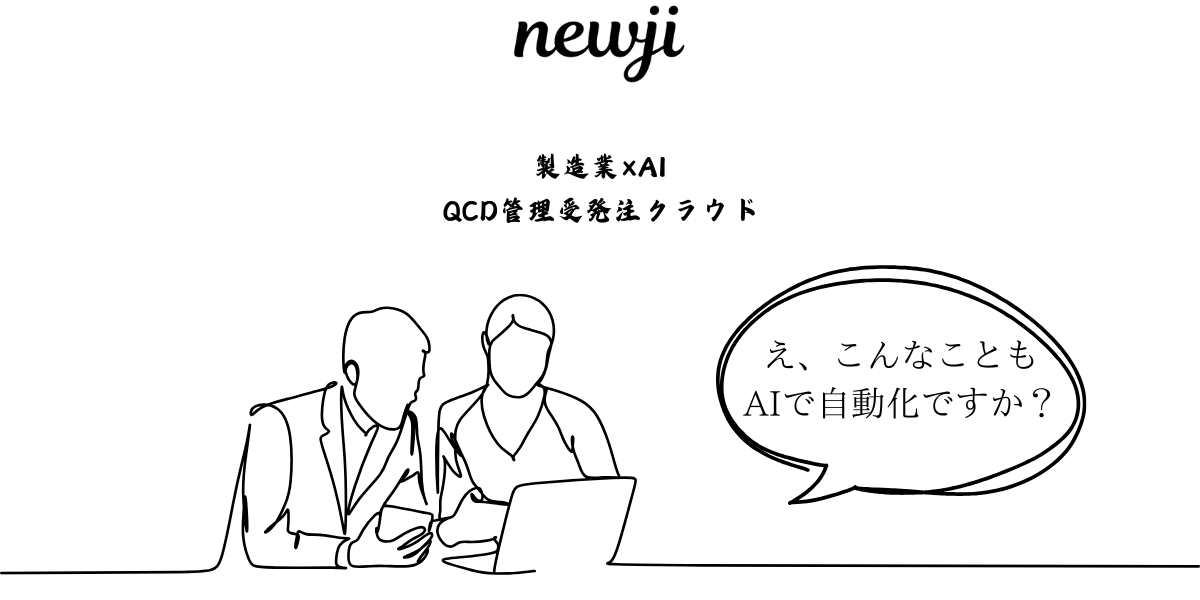
Enhancing Object Detection with Deep Learning for Real-World Applications
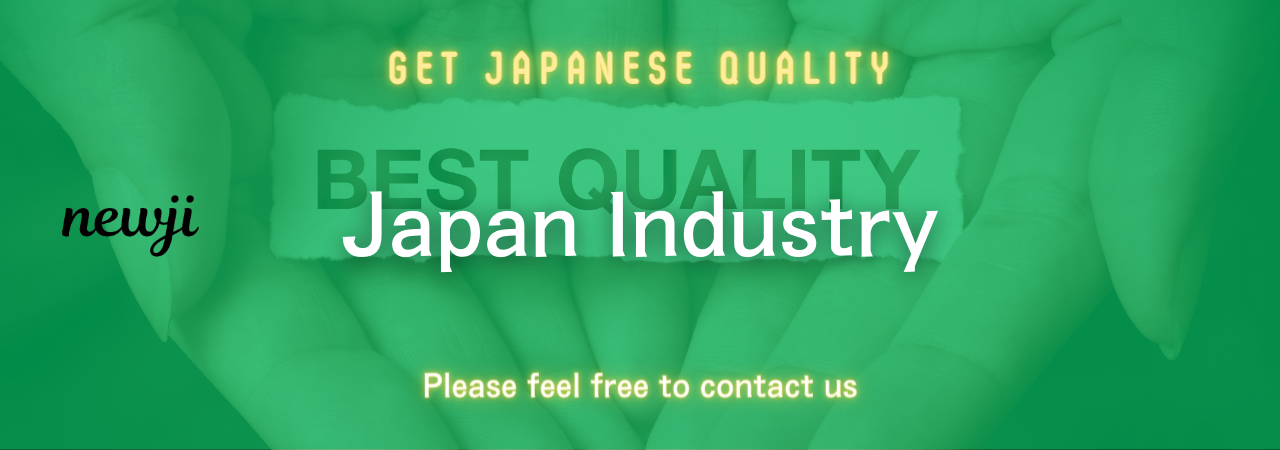
目次
Understanding Object Detection
Object detection is a crucial technology in today’s digital world, focusing on identifying and locating objects within an image or video.
It combines image classification and localization, helping systems understand what objects are present and where they are.
The application of object detection spans various fields, including autonomous driving, healthcare, retail, and security.
This technology enhances user experiences by providing improved interaction and automation capabilities.
To achieve this, deep learning techniques are employed, offering significant improvements over traditional machine learning methods.
What is Deep Learning?
Deep learning is a subset of machine learning that uses neural networks with many layers to model and understand complex patterns in data.
These layers allow systems to learn representations of the data with multiple levels of abstraction, which is essential for tasks like object detection.
Deep learning techniques have proven incredibly effective in enabling systems to excel in identifying and classifying objects in real-world scenarios.
By training models on vast datasets, they can generalize better to unseen data, making them ideal for dynamic and complex environments.
How Deep Learning Enhances Object Detection
Incorporating deep learning into object detection has revolutionized the field.
Here are a few ways deep learning has enhanced object detection for real-world applications:
1. Improved Accuracy
Deep learning models, especially convolutional neural networks (CNNs), have significantly boosted the accuracy of object detection systems.
They achieve this by automatically learning intricate features of objects, which helps in improving precision and recall rates.
This accuracy is critical in applications like autonomous vehicles, where missing or misidentifying an object can have dire consequences.
2. Real-Time Processing
Another advantage of deep learning methods is their ability to process data in real-time.
Techniques such as You Only Look Once (YOLO) and Single Shot MultiBox Detector (SSD) enable real-time object detection by optimizing computational efficiency.
This capability is particularly beneficial in industries requiring immediate responses, such as surveillance and robotics.
3. Handling Diverse Environments
Deep learning models excel in detecting objects across different environments and conditions due to their ability to learn diverse features.
They can handle variations in lighting, scale, and occlusion, making them robust for real-world applications.
This adaptability is essential for technologies that operate in uncontrolled settings, like drones or wildlife monitoring systems.
4. Transfer Learning
Transfer learning is a significant advancement, allowing models trained on one task to be adapted to another.
It enables quicker development by leveraging pre-trained models and fine-tuning them for specific applications.
This process reduces the need for extensive datasets and computational resources, facilitating the deployment of object detection solutions in new contexts rapidly.
Applications of Enhanced Object Detection
The integration of deep learning in object detection has expanded its applicability in numerous industries.
Autonomous Vehicles
In autonomous driving, object detection helps vehicles understand their surroundings by identifying other cars, pedestrians, traffic signals, and obstacles.
Enhanced detection improves safety and navigation efficiency, crucial for developing reliable self-driving technologies.
Healthcare Industry
In healthcare, object detection is used for medical imaging analysis, assisting in identifying anomalies like tumors or fractures.
By improving accuracy and reducing diagnostic times, it helps healthcare professionals provide better care to patients.
Retail and E-commerce
Retail businesses implement object detection to enhance customer experiences through automated checkout systems and inventory management.
For example, real-time product detection can streamline shopping by enabling cashier-less stores and promoting efficient stock monitoring.
Security and Surveillance
In security, enhanced object detection is employed for monitoring and identifying suspicious activities.
By providing robust surveillance solutions, it helps authorities maintain public safety and respond promptly to threats.
Challenges and Future Directions
While deep learning has significantly improved object detection, challenges remain.
Computational Costs
The computational demand of deep learning models can be high, requiring powerful hardware and resources.
Striking a balance between model complexity and efficiency remains a challenge for developers.
Data Privacy
The need for vast amounts of data to train these models presents privacy concerns.
Ensuring that data collection and usage comply with regulations is critical for maintaining trust and security.
Interpretability
Deep learning models often operate as “black boxes,” making it difficult to understand their decision-making processes.
Improving model transparency is necessary for building trust in AI-driven systems, especially in critical applications like healthcare.
Future Outlook
The future of object detection lies in addressing these challenges while expanding its capabilities.
Hybrid models, combining deep learning with other AI techniques, may offer solutions to current limitations.
Additionally, advancements in hardware, such as specialized AI chips, could alleviate computational burdens and enhance real-time processing.
Conclusion
Enhancing object detection with deep learning has already had a profound impact on numerous industries, leading to safer, more efficient, and smarter solutions.
As technology continues to evolve, so will the applications and accuracy of object detection systems, making them even more integral to our daily lives.
By overcoming current challenges and embracing innovation, the potential of deep learning in object detection will only continue to grow.
資料ダウンロード
QCD調達購買管理クラウド「newji」は、調達購買部門で必要なQCD管理全てを備えた、現場特化型兼クラウド型の今世紀最高の購買管理システムとなります。
ユーザー登録
調達購買業務の効率化だけでなく、システムを導入することで、コスト削減や製品・資材のステータス可視化のほか、属人化していた購買情報の共有化による内部不正防止や統制にも役立ちます。
NEWJI DX
製造業に特化したデジタルトランスフォーメーション(DX)の実現を目指す請負開発型のコンサルティングサービスです。AI、iPaaS、および先端の技術を駆使して、製造プロセスの効率化、業務効率化、チームワーク強化、コスト削減、品質向上を実現します。このサービスは、製造業の課題を深く理解し、それに対する最適なデジタルソリューションを提供することで、企業が持続的な成長とイノベーションを達成できるようサポートします。
オンライン講座
製造業、主に購買・調達部門にお勤めの方々に向けた情報を配信しております。
新任の方やベテランの方、管理職を対象とした幅広いコンテンツをご用意しております。
お問い合わせ
コストダウンが利益に直結する術だと理解していても、なかなか前に進めることができない状況。そんな時は、newjiのコストダウン自動化機能で大きく利益貢献しよう!
(Β版非公開)