- お役立ち記事
- Equipment maintenance evolving with AI: Practical points for abnormality detection and failure prediction
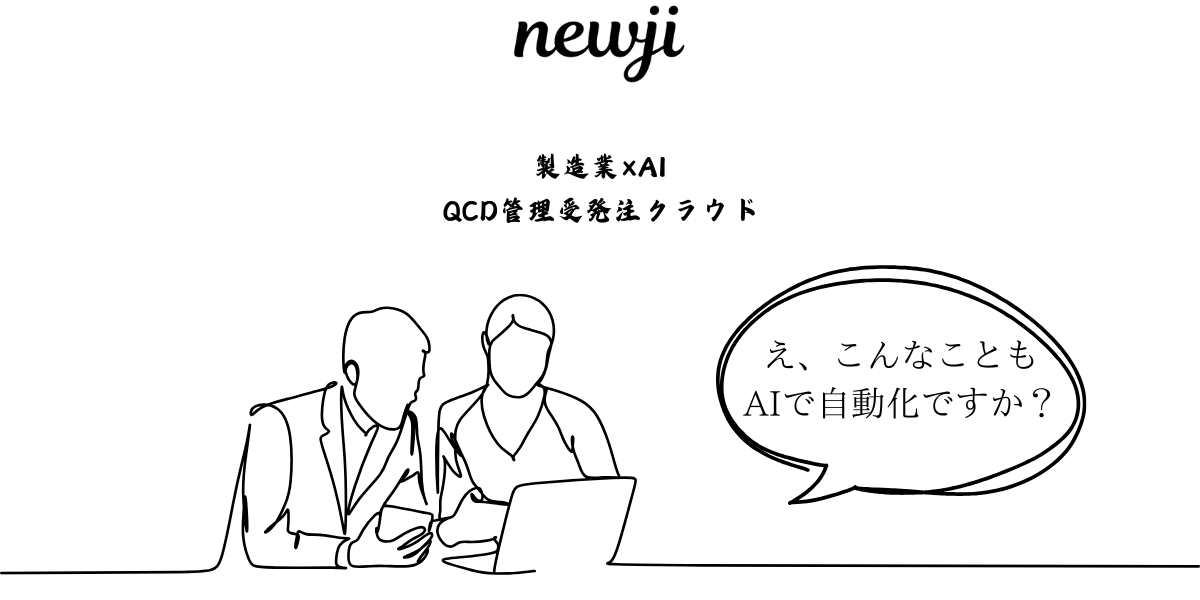
Equipment maintenance evolving with AI: Practical points for abnormality detection and failure prediction
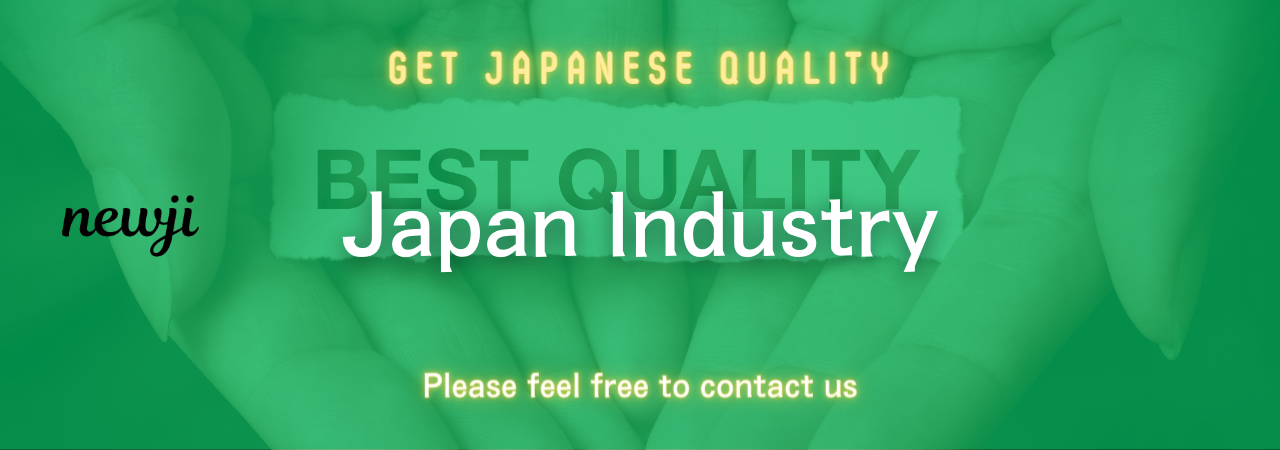
目次
Introduction to AI in Equipment Maintenance
In recent years, the integration of AI into equipment maintenance has transformed how industries manage machinery and avoid unexpected downtimes.
AI technology has opened up new possibilities for detecting abnormalities and predicting failures.
This not only improves efficiency but also significantly cuts costs related to machine downtime and repairs.
Predictive maintenance, powered by AI, shifts the paradigm from performing maintenance on a fixed schedule to a more dynamic and proactive approach.
With AI, industries can now leverage vast amounts of data collected from sensors and IoT devices to evaluate the condition of equipment in real-time.
Let’s explore how AI is evolving in equipment maintenance and delve into the key practical points for implementing this technology effectively.
Understanding Abnormality Detection
Abnormality detection is one of the fundamental applications of AI in equipment maintenance.
Traditionally, detecting discrepancies in machinery meant relying on manual inspections or basic monitoring systems that could easily miss early signs of failure.
AI changes that by continuously analyzing data from equipment and flagging any anomalies that may indicate a potential issue.
How AI Detects Abnormalities
AI models, particularly those based on machine learning algorithms, learn from historical data to identify patterns of normal operating conditions.
When current data deviates from these learned patterns, the system recognizes an anomaly.
These anomalies could be anything from unusual temperature spikes to irregular vibration patterns in a machine.
By detecting these discrepancies early, maintenance teams can take action before a serious problem arises.
This reduces downtime and prevents costly repairs.
Moreover, AI systems can prioritize anomalies, ensuring that the most critical issues receive immediate attention, enhancing overall operational efficiency.
The Role of IoT in Data Collection
The Internet of Things (IoT) plays a pivotal role in collecting the data needed for AI-powered abnormality detection.
IoT devices, such as sensors embedded in machinery, continuously gather information related to temperature, pressure, vibration, and other critical performance indicators.
This data is then processed and analyzed by AI algorithms to determine the health of the equipment.
Predictive Maintenance with AI
Another exciting application of AI in equipment maintenance is predictive maintenance.
Instead of servicing machinery at regular intervals, predictive maintenance uses AI to predict when a machine is likely to fail, allowing for maintenance to be performed just-in-time.
This approach not only extends the lifespan of equipment but also optimizes the efficiency of maintenance operations.
The Advantages of Predictive Maintenance
Predictive maintenance offers numerous benefits over traditional maintenance strategies.
Firstly, it minimizes unexpected failures by accurately predicting when a malfunction is likely to occur.
This ensures that maintenance can be scheduled at the most convenient times, reducing disruptions to production.
Furthermore, predictive maintenance reduces the frequency of maintenance inspections, saving time and labor costs.
Since maintenance is performed only when necessary, the resources are better utilized, avoiding unnecessary replacements of parts and materials.
Steps to Implement Predictive Maintenance
To implement predictive maintenance effectively, several steps must be followed:
1. **Data Collection:** Begin by gathering data from all relevant equipment using IoT sensors. This data provides the foundation for building reliable predictive models.
2. **Data Analysis:** Apply machine learning algorithms to analyze historical data and identify trends associated with equipment performance and failures.
3. **Model Training:** Train predictive models using historical and real-time data. Ensure these models are continuously updated to improve their accuracy over time.
4. **Integration:** Seamlessly integrate the predictive maintenance system with existing maintenance workflows and systems. This will facilitate easy adoption by maintenance teams.
5. **Continuous Monitoring:** Establish mechanisms for ongoing monitoring and adjustments to the predictive maintenance strategy, ensuring the system remains effective as equipment and conditions change.
Challenges and Considerations
While AI-driven equipment maintenance presents numerous advantages, there are also challenges and considerations to address for successful implementation.
Data Quality and Management
The success of AI applications heavily depends on the quality and volume of data.
Ensuring that data collected is accurate, comprehensive, and timely is crucial.
Furthermore, maintaining a robust data management system to handle and process the vast amounts of generated data is essential for effective analysis.
Integration with Legacy Systems
Many industries operate legacy systems that may not be immediately compatible with AI technologies.
Integrating AI solutions with these existing systems can be complex and may require modifications or upgrades to ensure successful interoperability.
Cost and Resource Allocation
Implementing AI in equipment maintenance can require significant initial investment in terms of technology and human resources.
Industries must carefully assess the cost-benefit ratio to ensure the long-term advantages justify the initial expenses.
Conclusion
AI is revolutionizing equipment maintenance by providing advanced tools for abnormality detection and failure prediction.
By utilizing AI, industries can achieve unprecedented levels of efficiency, reliability, and cost-effectiveness in maintaining their machinery.
Although challenges exist, they can be managed with careful planning and execution.
As AI continues to evolve, staying ahead of technological advancements and incorporating them into maintenance strategies will be crucial for industries aiming to enhance their operational excellence.
資料ダウンロード
QCD調達購買管理クラウド「newji」は、調達購買部門で必要なQCD管理全てを備えた、現場特化型兼クラウド型の今世紀最高の購買管理システムとなります。
ユーザー登録
調達購買業務の効率化だけでなく、システムを導入することで、コスト削減や製品・資材のステータス可視化のほか、属人化していた購買情報の共有化による内部不正防止や統制にも役立ちます。
NEWJI DX
製造業に特化したデジタルトランスフォーメーション(DX)の実現を目指す請負開発型のコンサルティングサービスです。AI、iPaaS、および先端の技術を駆使して、製造プロセスの効率化、業務効率化、チームワーク強化、コスト削減、品質向上を実現します。このサービスは、製造業の課題を深く理解し、それに対する最適なデジタルソリューションを提供することで、企業が持続的な成長とイノベーションを達成できるようサポートします。
オンライン講座
製造業、主に購買・調達部門にお勤めの方々に向けた情報を配信しております。
新任の方やベテランの方、管理職を対象とした幅広いコンテンツをご用意しております。
お問い合わせ
コストダウンが利益に直結する術だと理解していても、なかなか前に進めることができない状況。そんな時は、newjiのコストダウン自動化機能で大きく利益貢献しよう!
(Β版非公開)