- お役立ち記事
- Example of data quality assurance utilization using rule base and AI
月間76,176名の
製造業ご担当者様が閲覧しています*
*2025年3月31日現在のGoogle Analyticsのデータより
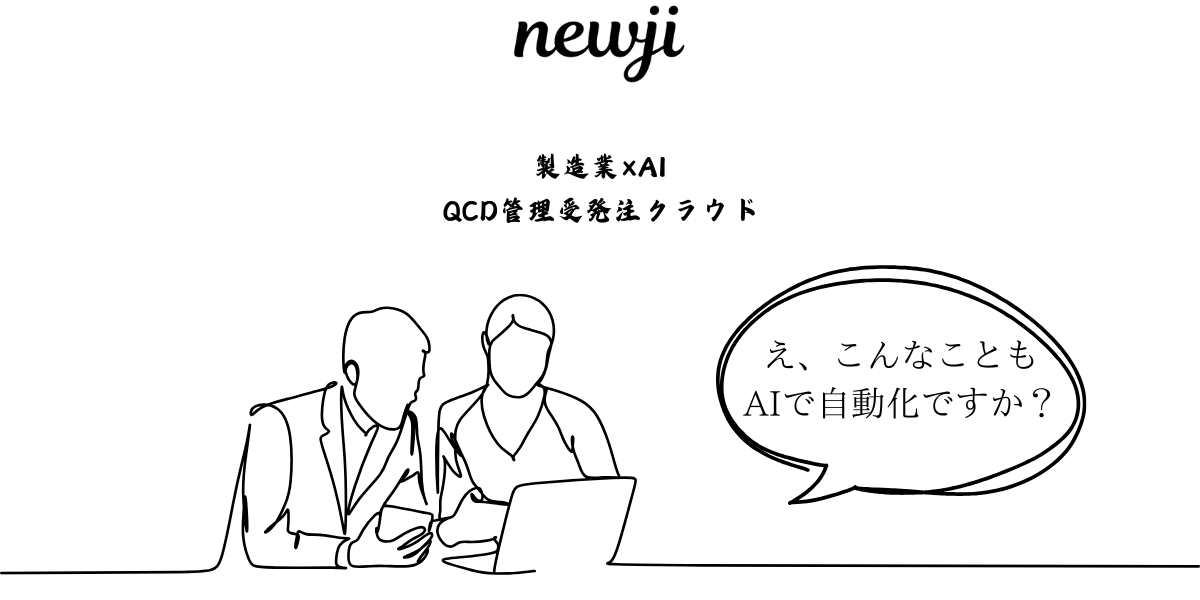
Example of data quality assurance utilization using rule base and AI
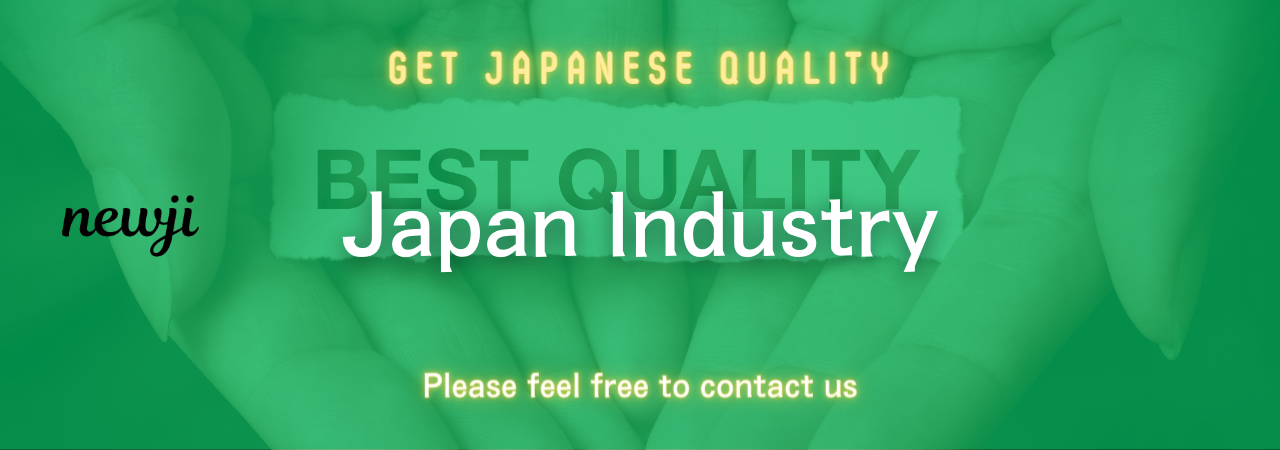
目次
Understanding Data Quality Assurance
Data quality assurance is a critical aspect of managing and utilizing data effectively.
In today’s world, where data drives decision-making processes across industries, ensuring the accuracy, reliability, and consistency of data is more important than ever.
Data quality assurance involves a set of practices aimed at identifying and correcting data errors to improve its reliability.
There are various approaches to achieve data quality assurance.
One popular method involves the use of rule-based systems and artificial intelligence (AI).
These technologies can significantly enhance the process of data validation and cleaning, leading to improved data quality.
The Role of Rule-Based Systems
Rule-based systems play an essential role in data quality assurance.
These systems use predefined rules to identify discrepancies and anomalies in data.
The rules are designed based on the specific requirements and objectives of the organization.
For instance, a rule may specify that a customer’s date of birth should not be a future date.
Another rule might ensure that customer email addresses follow the standard format of username@domain.com.
By applying these rules, organizations can easily spot errors and inconsistencies within their datasets.
The primary advantage of rule-based systems is their simplicity and effectiveness.
They allow organizations to implement straightforward and simple checks on data without the need for complex algorithms.
However, they may struggle with more complex data quality problems, which is where AI comes into play.
Leveraging AI for Data Quality Assurance
Artificial intelligence offers more advanced solutions for data quality assurance.
AI systems can learn from data patterns and make intelligent decisions to identify errors and improve data quality.
Machine learning models, a subset of AI, are particularly useful in this domain.
They can be trained using large datasets to detect patterns and anomalies that might be difficult to catch with traditional rule-based systems.
For example, AI can detect subtle changes in data patterns that indicate potentially erroneous data, such as an unusually high number of transactions in a short period.
These systems improve over time as they process more data, making them an effective tool for ongoing data quality assurance.
Moreover, AI can automate repetitive tasks involved in data cleaning.
By doing so, it reduces the time and resources required to maintain high data quality standards.
Combining Rule-Based Systems and AI
While rule-based systems and AI individually contribute to data quality assurance, their true potential is realized when they are used together.
Combining these approaches provides a comprehensive solution that leverages the strengths of both methodologies.
A typical use case would involve using rule-based systems to address straightforward validation checks, while AI handles more complex and nuanced data quality issues.
By integrating AI with rule-based systems, organizations can continuously monitor and enhance data quality more efficiently.
Practical Examples of Data Quality Assurance with Rule Base and AI
Let’s delve into some practical examples of how organizations utilize rule-based systems and AI for data quality assurance.
Financial Services
In the financial sector, data quality is paramount for ensuring regulatory compliance and accurate financial reporting.
Rule-based systems can verify transaction records, ensuring that each entry has valid account numbers and complies with the relevant financial regulations.
AI systems can further enhance these checks by analyzing transaction patterns to detect fraudulent activities or errors that may go unnoticed by rule-based systems alone.
This combination helps financial institutions maintain high data accuracy and integrity.
Healthcare
Healthcare organizations rely on accurate patient data to provide quality care and treatment.
Rule-based systems can check for completeness in medical records, ensuring that all required fields are filled.
AI can assist by predicting missing information based on existing patient data, such as suggesting possible diagnoses or treatments based on previous cases that share similar attributes.
This synergy ensures that healthcare providers have access to reliable data for making informed medical decisions.
Retail
In the retail industry, customer data is critical for personalized marketing and improving customer experience.
Rule-based systems are used to validate customer information, like ensuring all addresses are within serviceable regions.
AI enhances this process by analyzing purchasing patterns to suggest new products to customers based on their past buying behavior.
This improves customer satisfaction by making personalized recommendations while ensuring data accuracy.
Challenges and Future Prospects
Despite the promising benefits, implementing data quality assurance using rule-based systems and AI is not without challenges.
Organizations may face difficulties in defining appropriate rules for various datasets.
Similarly, developing and training AI models requires significant expertise and resources.
Machine learning models also need continuous updates to keep up with changing data patterns and user needs.
Organizations must invest in regular monitoring and retraining of AI systems to maintain data quality over time.
Looking ahead, advancements in AI technology are expected to further enhance data quality assurance methodologies.
New techniques, such as deep learning, could provide more accurate predictions and classifications.
Additionally, the integration of natural language processing (NLP) with AI systems can improve the analysis of unstructured data, expanding the scope of data quality assurance.
Conclusion
Data quality assurance is essential for organizations to make informed decisions and maintain data integrity.
By utilizing rule-based systems and AI, businesses can significantly improve the quality of their data.
This combination offers a powerful toolset capable of addressing both simple and complex data quality issues.
As technology continues to evolve, so too will the methods for ensuring data quality.
Organizations that invest in these innovative solutions will be better positioned to harness the full potential of their data assets.
資料ダウンロード
QCD管理受発注クラウド「newji」は、受発注部門で必要なQCD管理全てを備えた、現場特化型兼クラウド型の今世紀最高の受発注管理システムとなります。
ユーザー登録
受発注業務の効率化だけでなく、システムを導入することで、コスト削減や製品・資材のステータス可視化のほか、属人化していた受発注情報の共有化による内部不正防止や統制にも役立ちます。
NEWJI DX
製造業に特化したデジタルトランスフォーメーション(DX)の実現を目指す請負開発型のコンサルティングサービスです。AI、iPaaS、および先端の技術を駆使して、製造プロセスの効率化、業務効率化、チームワーク強化、コスト削減、品質向上を実現します。このサービスは、製造業の課題を深く理解し、それに対する最適なデジタルソリューションを提供することで、企業が持続的な成長とイノベーションを達成できるようサポートします。
製造業ニュース解説
製造業、主に購買・調達部門にお勤めの方々に向けた情報を配信しております。
新任の方やベテランの方、管理職を対象とした幅広いコンテンツをご用意しております。
お問い合わせ
コストダウンが利益に直結する術だと理解していても、なかなか前に進めることができない状況。そんな時は、newjiのコストダウン自動化機能で大きく利益貢献しよう!
(β版非公開)