- お役立ち記事
- Expansion of Bayesian modeling (applications)
Expansion of Bayesian modeling (applications)
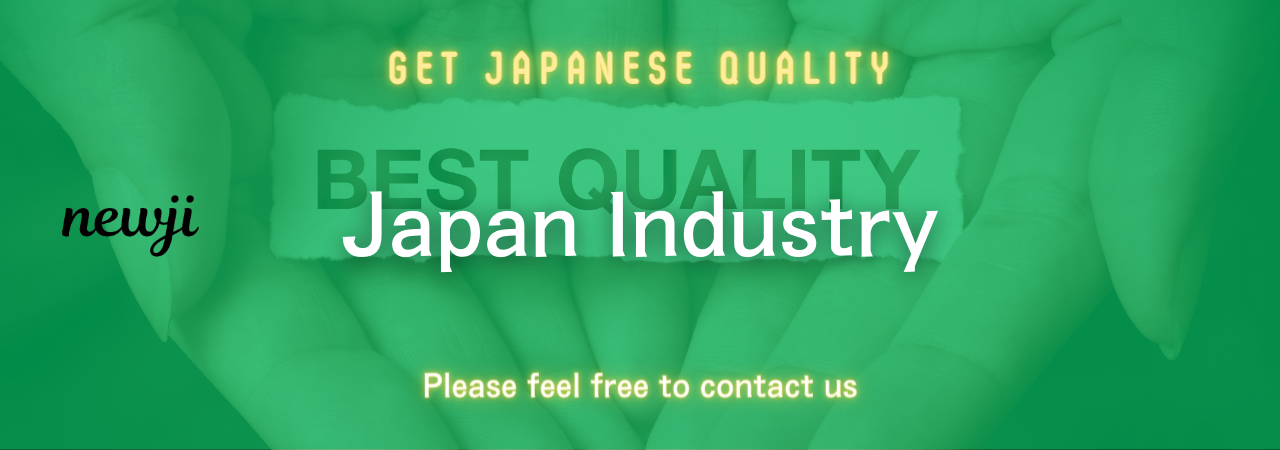
目次
Introduction to Bayesian Modeling
Bayesian modeling is a statistical method that uses probability to represent uncertainty in models and data analysis.
It builds on Bayes’ Theorem, which describes the probability of an event based on prior knowledge of related events.
Bayesian modeling is widely used across various fields for its ability to incorporate prior information and update beliefs with new evidence.
Over the years, the applications of Bayesian modeling have expanded considerably, finding utility in diverse areas such as healthcare, finance, and machine learning.
This article delves into some of the key applications that highlight how Bayesian models contribute to advancements in different industries.
Applications in Healthcare
Bayesian modeling has proved to be invaluable in the healthcare sector.
It aids in decision-making processes by integrating clinical data and expert knowledge, which is crucial for patient diagnosis and treatment planning.
For instance, Bayesian models can help predict the probability of disease occurrence based on genetic markers and lifestyle factors.
In clinical trials, Bayesian methods allow for the incorporation of prior studies and expert opinions, enabling more efficient trial designs.
This leads to faster conclusions about the efficacy of new therapies, which is crucial in drug development.
Furthermore, Bayesian networks are used in diagnostic systems to evaluate multiple hypotheses simultaneously, providing probabilities for different diagnoses.
This can improve the accuracy of diagnosis and personalize treatment plans, offering better patient outcomes.
Finance and Risk Management
In finance, Bayesian modeling has gained popularity for its capacity to model uncertainties and update predictions with new market data.
Bayesian models are leveraged in portfolio management to evaluate the risks and returns associated with different assets.
They help in optimizing investment strategies by accounting for uncertainty and market volatility.
Risk management is another area where Bayesian models shine.
Financial institutions use Bayesian inference to assess credit risk, allowing them to make informed lending decisions and set appropriate interest rates.
Bayesian methods are also applied in econometrics to predict economic variables, considering the uncertainty in economic models.
This can be instrumental in formulating monetary policies and conducting macroeconomic analysis.
Machine Learning and Artificial Intelligence
Bayesian modeling plays a critical role in the advancement of machine learning and artificial intelligence (AI).
It provides a probabilistic framework for machine learning algorithms, enabling them to model uncertainty intrinsically.
Bayesian methods are particularly useful in situations with limited data, where traditional frequentist approaches might struggle.
In supervised learning, Bayesian models can be used to update predictions as more data becomes available, improving model accuracy over time.
This is crucial for applications such as image and speech recognition, where new data continuously flows in.
In reinforcement learning, Bayesian approaches help in exploring and exploiting the environment by strategically updating the agent’s belief about the best actions.
This leads to more efficient learning in complex environments.
Furthermore, Bayesian optimization is used to fine-tune hyperparameters of machine learning models, enhancing their performance without exhaustive searches.
This is particularly beneficial in the field of neural networks, where optimal hyperparameter selection is vital for model success.
Environmental Science and Ecology
Bayesian modeling is extensively used in environmental science to deal with uncertainties inherent in ecological data.
It helps in understanding the dynamics of ecosystems and predicting changes due to various factors such as climate change and human activities.
For example, Bayesian methods are applied to model species distribution, incorporating uncertainties from various sources such as environmental variations and observational errors.
This can inform conservation strategies and biodiversity management.
In climate modeling, Bayesian approaches allow for the integration of different climate models and observational data, providing a probabilistic assessment of future climate scenarios.
This is crucial for policy-making and planning climate adaptation strategies.
Social Sciences and Humanities
In the social sciences, Bayesian models assist in understanding complex social phenomena by incorporating prior information and handling diverse data types.
For instance, Bayesian networks are used in sociology and political science to model relationships between social variables and predict social changes.
Bayesian methods are also applied in psychology, where they help in modeling cognitive processes and understanding human behavior.
They allow researchers to update hypotheses about mental states with empirical evidence, improving the interpretation of psychological data.
In the humanities, Bayesian approaches can be used in textual analysis and historical research, where they facilitate the evaluation of evidence and the estimation of historical event probabilities.
Conclusion
The expansion of Bayesian modeling applications across various fields highlights its versatility and strength in dealing with uncertainty and integrating prior knowledge.
From healthcare and finance to machine learning and environmental science, Bayesian models continue to contribute to advancements and innovation.
As computational capabilities grow and data availability increases, we can expect Bayesian modeling to become even more prevalent, further influencing research, decision-making, and policy across multiple disciplines.
The potential for Bayesian models to continue influencing various fields seems boundless, promising a future rich with data-driven insights and improved decision-making processes.
資料ダウンロード
QCD調達購買管理クラウド「newji」は、調達購買部門で必要なQCD管理全てを備えた、現場特化型兼クラウド型の今世紀最高の購買管理システムとなります。
ユーザー登録
調達購買業務の効率化だけでなく、システムを導入することで、コスト削減や製品・資材のステータス可視化のほか、属人化していた購買情報の共有化による内部不正防止や統制にも役立ちます。
NEWJI DX
製造業に特化したデジタルトランスフォーメーション(DX)の実現を目指す請負開発型のコンサルティングサービスです。AI、iPaaS、および先端の技術を駆使して、製造プロセスの効率化、業務効率化、チームワーク強化、コスト削減、品質向上を実現します。このサービスは、製造業の課題を深く理解し、それに対する最適なデジタルソリューションを提供することで、企業が持続的な成長とイノベーションを達成できるようサポートします。
オンライン講座
製造業、主に購買・調達部門にお勤めの方々に向けた情報を配信しております。
新任の方やベテランの方、管理職を対象とした幅広いコンテンツをご用意しております。
お問い合わせ
コストダウンが利益に直結する術だと理解していても、なかなか前に進めることができない状況。そんな時は、newjiのコストダウン自動化機能で大きく利益貢献しよう!
(Β版非公開)