- お役立ち記事
- Fatigue Strength Basics and Machine Learning for Fracture Cause Prediction
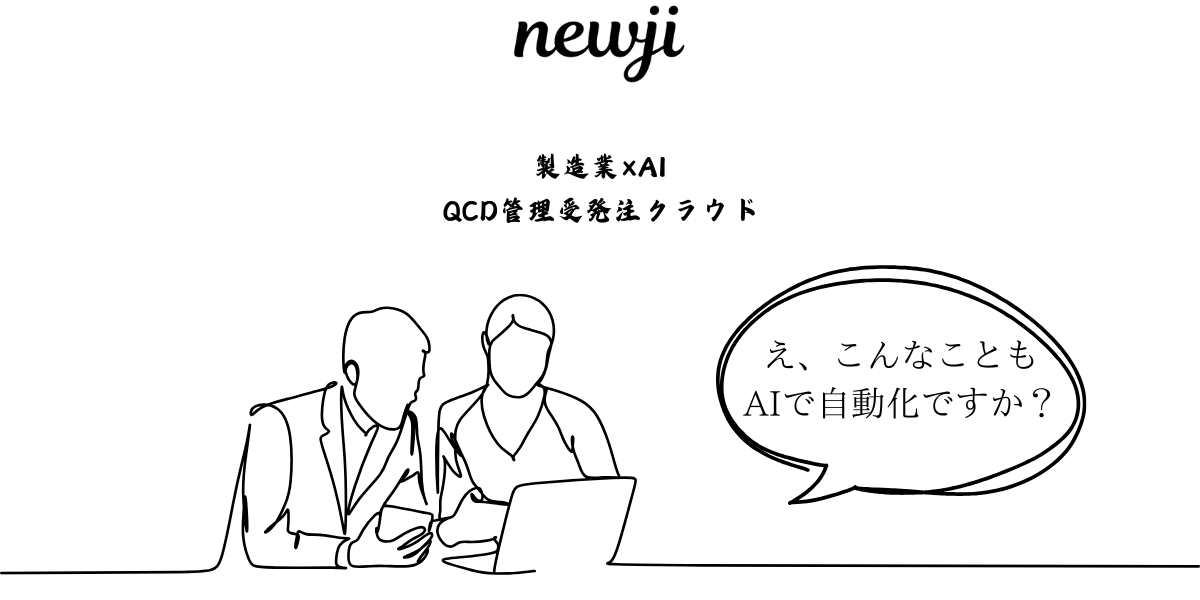
Fatigue Strength Basics and Machine Learning for Fracture Cause Prediction
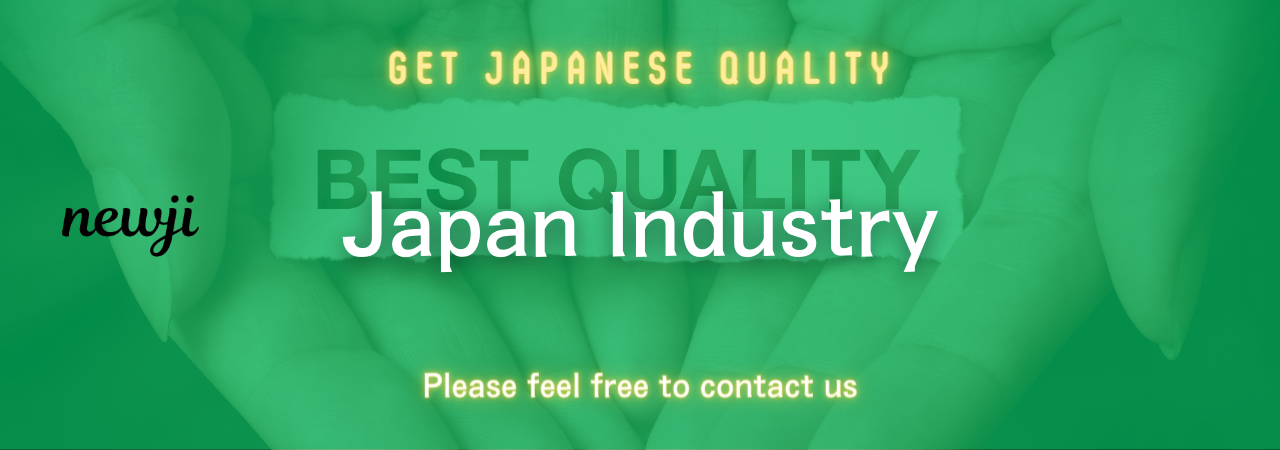
Fatigue strength is a critical concept in engineering and materials science, especially when it comes to understanding how materials behave under repetitive stress.
It’s the ability of a material to withstand cyclic loading and resist failure over time.
When a material is subjected to repeated loading and unloading, it may fail at stress levels well below its tensile strength.
This phenomenon is what we refer to as fatigue.
Understanding fatigue strength is essential for engineers and designers to ensure the longevity and safety of structures and machines.
目次
What is Fatigue Strength?
Fatigue strength refers to the highest stress level a material can withstand for a specified number of cycles without failing.
This attribute is vital for designing components that experience fluctuating loads, such as bridges, aircraft, and automotive parts.
The fatigue limit, or endurance limit, is the stress level below which a material can endure an infinite number of cycles without breaking.
However, not all materials exhibit a clear fatigue limit, especially non-ferrous metals and some composite materials.
The Importance of Fatigue Testing
Fatigue testing is crucial to determine the fatigue strength of materials.
In a fatigue test, a specimen is subjected to repeated cyclic loading until it fails.
The number of cycles to failure is recorded, and this data helps build an S-N curve (stress vs. number of cycles curve).
This curve shows the relationship between the stress amplitude and the number of cycles a material can sustain before failure.
Engineers use these curves to design components that can withstand a specific number of cycles at a given stress level.
Factors Affecting Fatigue Strength
Several factors impact the fatigue strength of a material:
Material Composition
Different materials have varying fatigue strengths.
For instance, steel generally has a higher fatigue strength compared to aluminum.
The presence of impurities, voids, or inclusions in a material can significantly reduce its fatigue strength.
Surface Finish
A smooth surface finish can improve the fatigue strength of a material.
Surface irregularities, such as scratches or notches, can act as stress concentrators and reduce fatigue strength.
Environmental Conditions
Environmental factors like temperature, humidity, and exposure to corrosive elements can affect fatigue strength.
For example, corrosive environments may lead to accelerated fatigue crack growth.
Geometric Factors
The design and shape of a component can influence its fatigue strength.
Sharp corners or abrupt changes in cross-section can lead to stress concentrations, which reduce fatigue strength.
Machine Learning for Fracture Cause Prediction
With advancements in technology, machine learning is becoming a valuable tool in predicting the causes of material fractures.
By analyzing vast amounts of data, machine learning algorithms can identify patterns and factors contributing to fatigue failure.
This predictive capability helps engineers diagnose potential issues before they become critical.
The Role of Data in Machine Learning
Machine learning relies heavily on data.
For predicting fatigue failures, data from fatigue tests, material properties, environmental conditions, and operational loads are essential.
Machine learning models can analyze patterns in this data, identifying correlations that might not be immediately apparent to human analysts.
Types of Machine Learning Algorithms Used
Several machine learning algorithms are used for fatigue analysis, including:
– **Supervised Learning:** This involves training the model on a labeled dataset, where the input and output data are known.
The model learns the relationship between inputs and outputs, making it a useful tool for predicting fatigue life based on known factors.
– **Unsupervised Learning:** This type of learning is used when there is no labeled data.
The algorithm identifies patterns and groupings autonomously, which can be useful for identifying unknown factors contributing to fatigue.
– **Reinforcement Learning:** This approach involves training a model through trial and error.
It can be used to optimize designs by simulating different loading conditions and iteratively improving the model’s predictions.
Applications of Machine Learning in Fatigue Analysis
Machine learning offers several applications in fatigue analysis:
Predictive Maintenance
By predicting when a component is likely to fail, machine learning enables predictive maintenance, reducing downtime and preventing catastrophic failures.
Design Optimization
Machine learning can help engineers optimize designs for maximum fatigue life by identifying critical factors affecting fatigue and suggesting design improvements.
Real-time Monitoring
Machine learning models can be integrated into sensors for real-time monitoring of components under cyclic loads.
This allows for immediate detection and response to potential fatigue issues.
Failure Analysis
In cases where failure has already occurred, machine learning can help identify the root causes, aiding in future design improvements and prevention strategies.
Challenges and Future Directions
While machine learning offers significant potential for fatigue analysis, there are challenges to overcome, such as data quality, model accuracy, and the interpretability of machine learning results.
However, ongoing research and technological advancements continue to improve these areas.
In the future, we can expect more sophisticated models with better predictive capabilities and integration with other technologies like the Internet of Things (IoT) for enhanced monitoring and analysis of fatigue in real-world applications.
資料ダウンロード
QCD調達購買管理クラウド「newji」は、調達購買部門で必要なQCD管理全てを備えた、現場特化型兼クラウド型の今世紀最高の購買管理システムとなります。
ユーザー登録
調達購買業務の効率化だけでなく、システムを導入することで、コスト削減や製品・資材のステータス可視化のほか、属人化していた購買情報の共有化による内部不正防止や統制にも役立ちます。
NEWJI DX
製造業に特化したデジタルトランスフォーメーション(DX)の実現を目指す請負開発型のコンサルティングサービスです。AI、iPaaS、および先端の技術を駆使して、製造プロセスの効率化、業務効率化、チームワーク強化、コスト削減、品質向上を実現します。このサービスは、製造業の課題を深く理解し、それに対する最適なデジタルソリューションを提供することで、企業が持続的な成長とイノベーションを達成できるようサポートします。
オンライン講座
製造業、主に購買・調達部門にお勤めの方々に向けた情報を配信しております。
新任の方やベテランの方、管理職を対象とした幅広いコンテンツをご用意しております。
お問い合わせ
コストダウンが利益に直結する術だと理解していても、なかなか前に進めることができない状況。そんな時は、newjiのコストダウン自動化機能で大きく利益貢献しよう!
(Β版非公開)