- お役立ち記事
- Feature extraction method using image processing and deep learning and its application to anomaly detection
月間77,185名の
製造業ご担当者様が閲覧しています*
*2025年2月28日現在のGoogle Analyticsのデータより
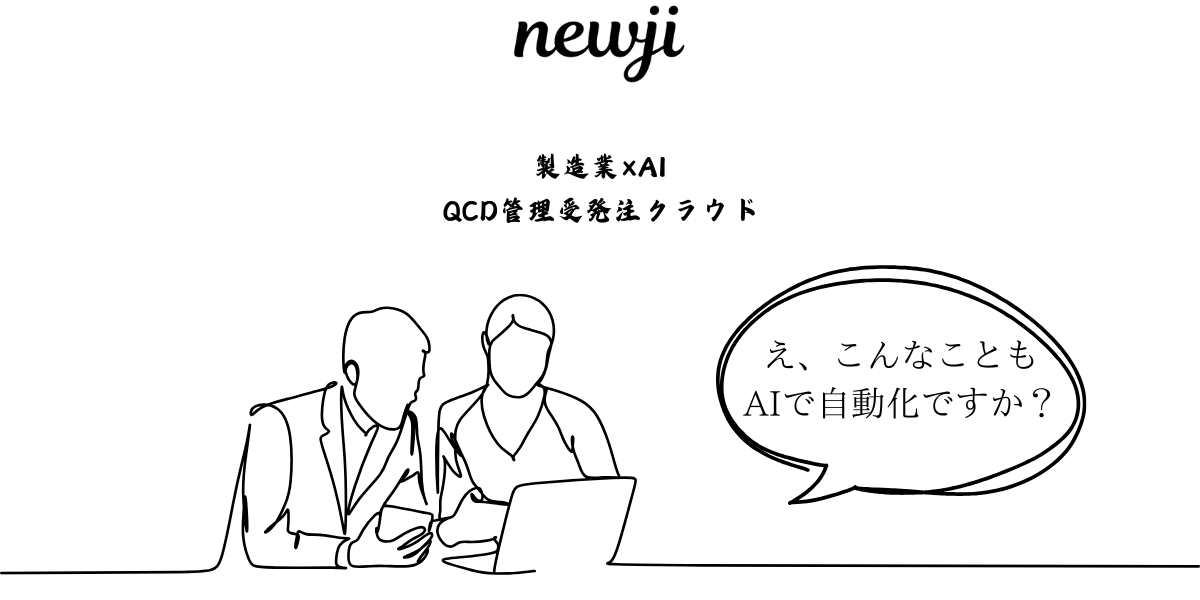
Feature extraction method using image processing and deep learning and its application to anomaly detection
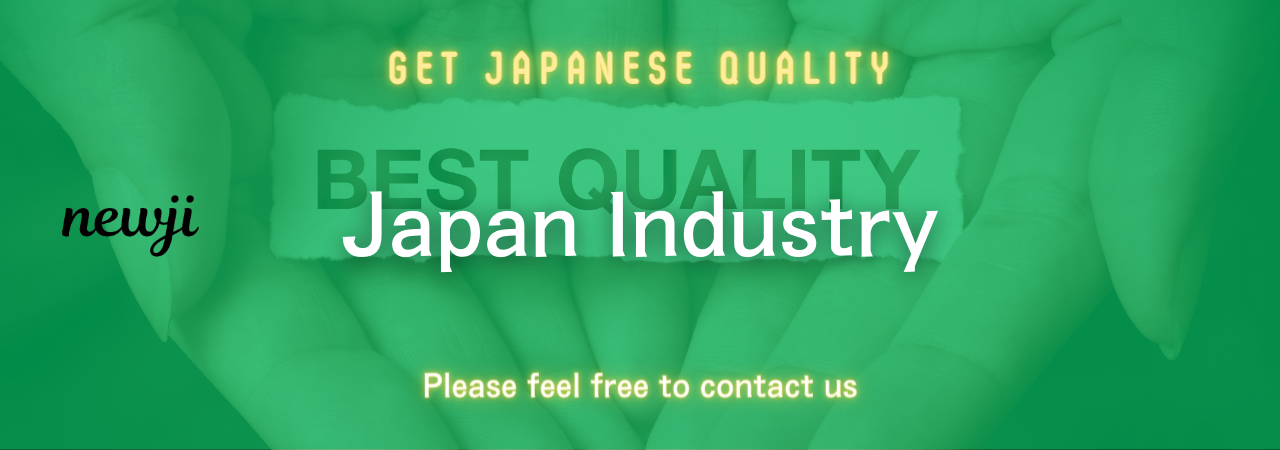
目次
Introduction to Feature Extraction
Feature extraction is a fundamental step in image processing and machine learning, especially when dealing with complex data such as images.
By extracting important features from an image, it becomes easier for a machine to understand and interpret that image.
These features could include edges, textures, shapes, or even colors that help in identifying various objects within the image.
The combination of image processing and deep learning has proved to be a powerful strategy for feature extraction.
Image processing techniques are used to enhance the image, making it easier for deep learning models to extract features.
Deep learning models, particularly Convolutional Neural Networks (CNNs), are capable of automatically identifying relevant features, making them ideal for image classification tasks.
Image Processing Techniques
Image processing plays a critical role in preparing images for deep learning models.
Some common image processing techniques include filtering, edge detection, and thresholding.
Filtering helps in removing noise from images, ensuring that the features extracted are clear and distinct.
Edge detection is employed to identify the boundaries of objects within the image, which is crucial for object recognition tasks.
Thresholding helps in segmenting the image into various parts or regions that may be of interest for further analysis.
These preprocessing steps are essential for ensuring that the images fed into deep learning models are of high quality, improving the accuracy of feature extraction.
Without proper image processing, deep learning models may struggle to perform well, resulting in poor outcomes in tasks such as classification or anomaly detection.
Deep Learning for Feature Extraction
Deep learning has revolutionized the way features are extracted from images.
Convolutional Neural Networks (CNNs), a type of deep learning model, are highly effective for this purpose due to their architecture, which mimics the human visual system.
CNNs consist of layers that convolve over the input image, enabling them to detect patterns and features at various levels of abstraction.
The process starts with the initial layers focusing on simple features like edges and colors.
As the data moves through deeper layers, more intricate and abstract features are identified.
This hierarchical feature extraction method allows deep learning models to comprehend complex structures within images, making them suitable for a wide range of applications, from facial recognition to medical imaging.
Application to Anomaly Detection
Feature extraction using image processing and deep learning can be particularly beneficial for anomaly detection.
Anomaly detection involves identifying patterns in data that deviate from expected behavior or norm, which is vital in fields like manufacturing, healthcare, and security.
When applying feature extraction for anomaly detection, the focus is on identifying features that signify irregularities or unusual patterns.
For example, in industrial settings, anomalies could indicate a defect in a product, while in medical imaging, they might suggest abnormalities in a patient’s scan.
By leveraging the power of deep learning, it’s possible to detect these anomalies accurately and efficiently.
Anomaly Detection Techniques
Several techniques can be used for anomaly detection once features have been extracted.
One common method is supervised learning, where models are trained on labeled data with known outcomes.
These models learn what constitutes a normal and an anomalous example, making predictions on unseen data based on this learning.
Another method is unsupervised learning, where the model identifies anomalies without any prior labels.
In this approach, models like autoencoders are often employed.
Autoencoders learn to reconstruct input data, with anomalies detected as instances where reconstruction significantly deviates from the original input.
Advantages of Using Deep Learning for Anomaly Detection
Utilizing deep learning for anomaly detection presents several advantages.
Firstly, deep learning models, especially CNNs, can process large volumes of data and identify subtle patterns that may not be apparent to human observers or traditional algorithms.
This scalability is crucial in environments where data generation is continuous and massive.
Secondly, deep learning models can adapt to new types of data and anomalies over time, making them adaptive and flexible tools in dynamic environments.
Furthermore, they offer the potential for real-time anomaly detection, providing timely alerts and enabling prompt intervention, which is particularly valuable in industries such as finance, health care, and cybersecurity.
Implementation Challenges
Despite the advantages, implementing deep learning for anomaly detection comes with its challenges.
The requirement for extensive labeled datasets for supervised learning can be a significant barrier, as anomaly data is often scarce or imbalanced.
Moreover, training deep learning models is computationally intensive and can be prohibitively expensive without access to high-performance computing resources.
Interpretability is another challenge.
Deep learning models are often seen as “black boxes,” making it difficult to understand how they make decisions.
Improving model transparency and interpretability is crucial, particularly in sensitive fields such as healthcare.
Conclusion
Feature extraction using image processing and deep learning is a powerful approach to tackling complex image-related tasks, with applications extending to anomaly detection.
By combining effective image processing techniques with the advanced pattern recognition capabilities of deep learning models, it is possible to achieve high levels of accuracy and efficiency in anomaly detection.
Although challenges such as data requirements and interpretability remain, ongoing research and advancements continue to mitigate these issues.
As technology evolves, the integration of image processing and deep learning for feature extraction and anomaly detection will become increasingly intuitive and accessible, driving innovation across multiple fields.
資料ダウンロード
QCD管理受発注クラウド「newji」は、受発注部門で必要なQCD管理全てを備えた、現場特化型兼クラウド型の今世紀最高の受発注管理システムとなります。
ユーザー登録
受発注業務の効率化だけでなく、システムを導入することで、コスト削減や製品・資材のステータス可視化のほか、属人化していた受発注情報の共有化による内部不正防止や統制にも役立ちます。
NEWJI DX
製造業に特化したデジタルトランスフォーメーション(DX)の実現を目指す請負開発型のコンサルティングサービスです。AI、iPaaS、および先端の技術を駆使して、製造プロセスの効率化、業務効率化、チームワーク強化、コスト削減、品質向上を実現します。このサービスは、製造業の課題を深く理解し、それに対する最適なデジタルソリューションを提供することで、企業が持続的な成長とイノベーションを達成できるようサポートします。
製造業ニュース解説
製造業、主に購買・調達部門にお勤めの方々に向けた情報を配信しております。
新任の方やベテランの方、管理職を対象とした幅広いコンテンツをご用意しております。
お問い合わせ
コストダウンが利益に直結する術だと理解していても、なかなか前に進めることができない状況。そんな時は、newjiのコストダウン自動化機能で大きく利益貢献しよう!
(β版非公開)