- お役立ち記事
- Features of deep learning and differences from machine learning
月間76,176名の
製造業ご担当者様が閲覧しています*
*2025年3月31日現在のGoogle Analyticsのデータより
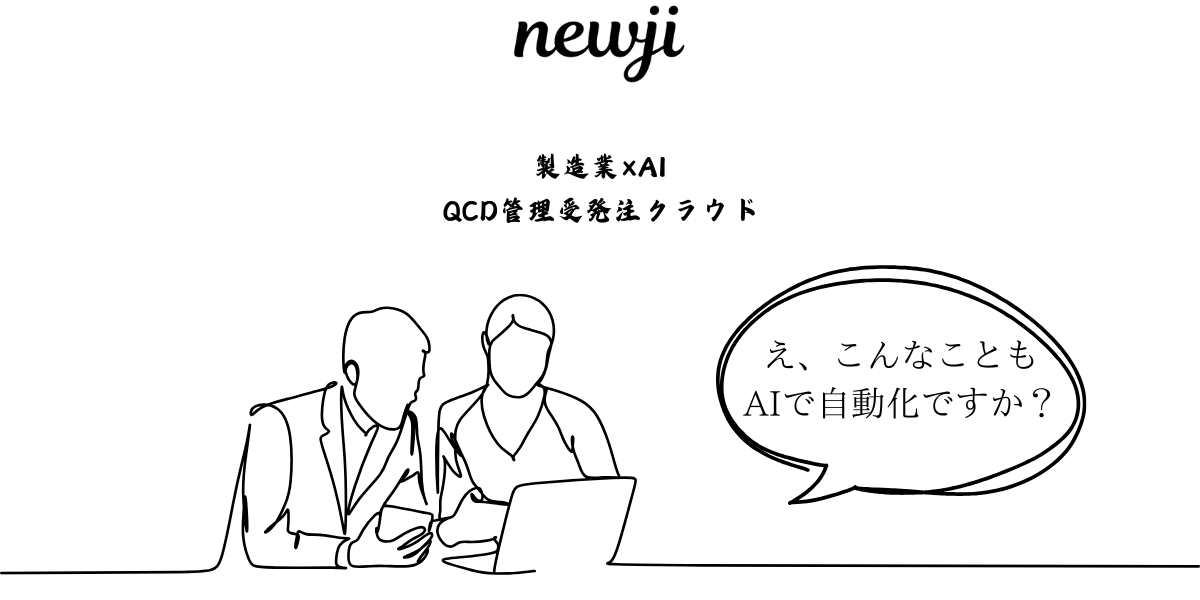
Features of deep learning and differences from machine learning
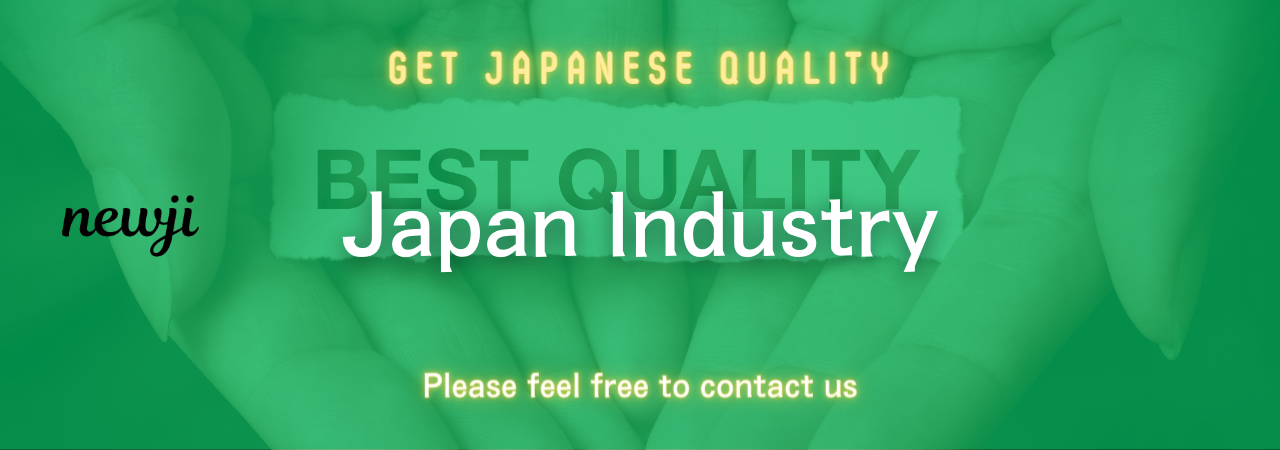
目次
Understanding Deep Learning
Deep learning is a subfield of artificial intelligence (AI) and machine learning that imitates the human brain in processing data and creating patterns for decision-making.
It involves neural networks with three or more layers.
These neural networks attempt to simulate the behavior of the human brain using data inputs, weights, and biases to identify, understand, and predict outcomes.
Deep learning is capable of learning from unstructured data, such as images and audio, making it extremely powerful and versatile in scenarios involving complex data.
For instance, deep learning can be applied to recognize images, process natural language, translate languages, and even drive vehicles autonomously.
How Deep Learning Works
Neural networks are the backbone of deep learning.
They consist of a series of interconnected layers—input, hidden, and output layers.
Data is fed through the input layers, processed through one or more hidden layers, and results are produced in the output layers.
In each layer, the network performs computations, extracting features or patterns from the data.
Neurons, which are individual processing units within these layers, each have weights and biases that together determine the neuron’s output.
Neurons are activated only when certain conditions are met, a process guided by activation functions.
As data travels through the network, the model adjusts these weights and biases to improve accuracy, a key feature of deep learning.
The training process involves using massive datasets, enabled by advancements in computing power and data storage.
The network performs a series of operations on the input data, compares its prediction to the actual outcome, and iteratively adjusts to minimize errors.
Machine Learning vs. Deep Learning
While deep learning is a subset of machine learning, there are significant differences that set them apart.
1. Data Dependency
Machine learning algorithms, especially traditional ones, can perform well with limited data.
Deep learning, however, thrives on large volumes of data as it requires diverse datasets to learn effectively and make accurate predictions.
This is because deep learning models involve numerous parameters that need to be tuned using vast amounts of input data.
2. Feature Engineering
In machine learning, feature engineering is crucial and requires humans to specify the attributes from input data that the computer can understand.
It involves selecting, modifying, or creating features to improve the model’s predictive performance.
In contrast, deep learning models inherently manage feature extraction and selection.
This automation significantly reduces the manual work involved and is a major advantage, especially in complex tasks like image and speech recognition.
3. Interpretability
One of the major challenges with deep learning is its lack of interpretability.
Deep learning models are often seen as “black boxes” because they operate at such a high level of complexity that understanding how they make decisions is difficult.
Traditional machine learning models tend to be more interpretable and offer some insights into the model’s decision process.
This transparency is crucial in sectors where traceability and accountability are important, like healthcare or finance.
4. Computational Power
Deep learning requires significantly more computational resources compared to traditional machine learning.
The training phase in deep learning involves numerous mathematical computations, which are CPU and GPU-intensive, requiring advanced hardware such as high-end graphics cards.
Traditional machine learning models usually do not require this level of computational power and can be executed on regular computer systems.
Applications of Deep Learning
Deep learning has been revolutionary across various industries due to its ability to process and learn from large, complex datasets.
Some of its most common applications include:
1. Image and Video Recognition
Deep learning excels at identifying and classifying images or videos due to its capability to recognize intricate patterns.
Applications include facial recognition systems, photo tagging in social media platforms, and automated security cameras.
2. Natural Language Processing (NLP)
In NLP, deep learning is used to analyze and understand human languages.
This ability is the backbone of technologies such as virtual assistants, translation apps, and voice-processing applications.
3. Autonomous Vehicles
Deep learning supports the development of self-driving cars by using sensors and cameras to identify objects, process signals, and make real-time driving decisions.
The model’s ability to learn from vast datasets enables autonomous vehicles to improve safety and efficiency on the road.
4. Healthcare
In the healthcare sector, deep learning assists in diagnosing diseases, developing drugs, and personalizing patient treatment plans based on predictive analytics.
Its pattern recognition capabilities are ideal for detecting anomalies in medical imaging.
The Future of Deep Learning
As the field continues to evolve, deep learning is expected to become more efficient and accessible.
Efforts are being made to make these models more interpretable and less reliant on enormous datasets, which could broaden their use and acceptance in critical fields.
Additionally, advancements in AI and deep learning could lead to innovations in robotics, genome sequencing, and personalized medicine, enhancing how we interact with technology and improving human life quality.
Understanding these foundational concepts provides insight into why deep learning is so important and how it differs from traditional machine learning techniques.
The trajectory of deep learning may well shape the future of technology as we know it.
資料ダウンロード
QCD管理受発注クラウド「newji」は、受発注部門で必要なQCD管理全てを備えた、現場特化型兼クラウド型の今世紀最高の受発注管理システムとなります。
ユーザー登録
受発注業務の効率化だけでなく、システムを導入することで、コスト削減や製品・資材のステータス可視化のほか、属人化していた受発注情報の共有化による内部不正防止や統制にも役立ちます。
NEWJI DX
製造業に特化したデジタルトランスフォーメーション(DX)の実現を目指す請負開発型のコンサルティングサービスです。AI、iPaaS、および先端の技術を駆使して、製造プロセスの効率化、業務効率化、チームワーク強化、コスト削減、品質向上を実現します。このサービスは、製造業の課題を深く理解し、それに対する最適なデジタルソリューションを提供することで、企業が持続的な成長とイノベーションを達成できるようサポートします。
製造業ニュース解説
製造業、主に購買・調達部門にお勤めの方々に向けた情報を配信しております。
新任の方やベテランの方、管理職を対象とした幅広いコンテンツをご用意しております。
お問い合わせ
コストダウンが利益に直結する術だと理解していても、なかなか前に進めることができない状況。そんな時は、newjiのコストダウン自動化機能で大きく利益貢献しよう!
(β版非公開)