- お役立ち記事
- Fluid Dynamics and Reinforcement Learning for Prediction and Optimal Control
月間77,185名の
製造業ご担当者様が閲覧しています*
*2025年2月28日現在のGoogle Analyticsのデータより
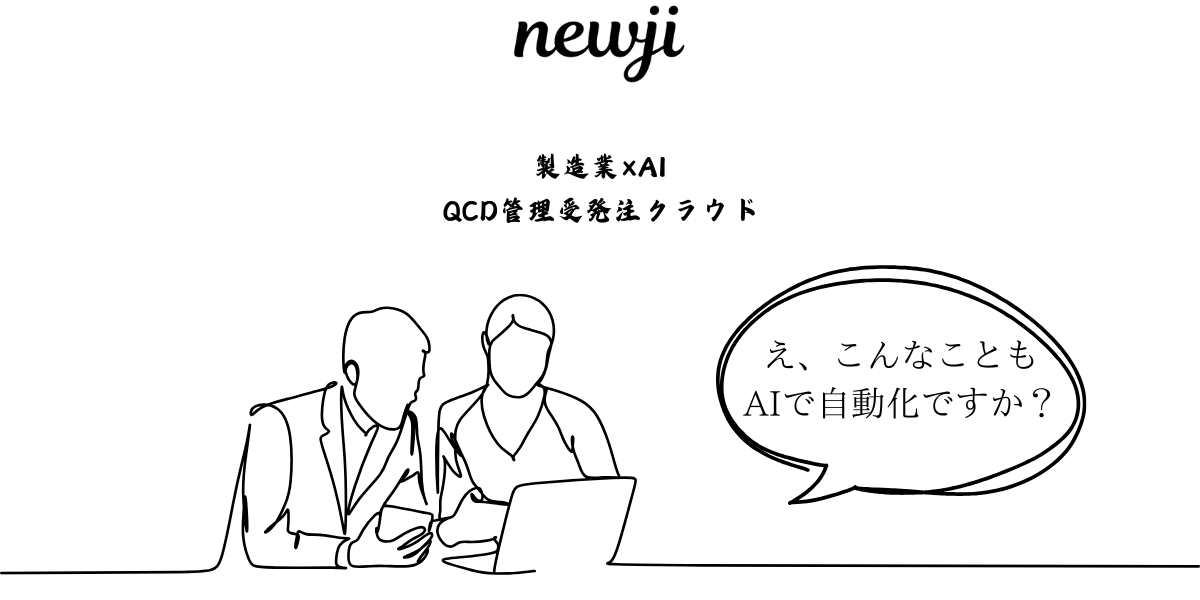
Fluid Dynamics and Reinforcement Learning for Prediction and Optimal Control
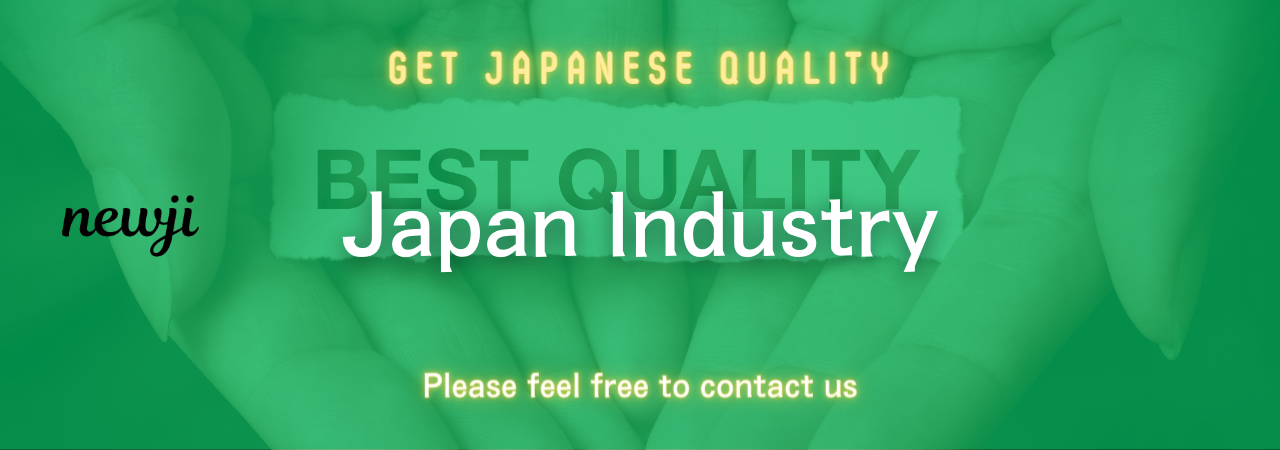
目次
Understanding Fluid Dynamics
Fluid dynamics is a fascinating branch of physics that focuses on the behavior of liquids and gases in motion.
It plays a crucial role in a myriad of industries and disciplines, from aerodynamics in transportation to the study of blood flow in biological systems.
When we talk about fluid dynamics, we refer to the study of fluid flow patterns, the pressure exerted by fluids, and the energy and interaction in fluid systems.
One of the fundamental concepts within fluid dynamics is understanding the forces that act upon the fluid particles.
These include gravitational forces, pressure gradients, and viscous forces.
The study of these forces helps predict how fluids will move under different conditions, which is essential for efficiency and safety in engineering applications.
Key Equations in Fluid Dynamics
In fluid dynamics, several key equations are used to model and predict fluid behavior.
The first and perhaps most significant is the Navier-Stokes equation, which describes how the velocity field of a fluid substance behaves.
It incorporates factors such as viscosity, pressure, density, and external forces to predict the motion of fluid particles.
Bernoulli’s principle is another fundamental concept, explaining how the speed of a fluid relates to its pressure.
According to this principle, an increase in the speed of a fluid occurs simultaneously with a decrease in pressure or a reduction in the fluid’s potential energy.
By applying these equations, engineers and scientists can design systems that manage fluid flow more effectively, whether it’s the combustion engine of a car or the air conditioning system in a building.
Role of Reinforcement Learning in Fluid Dynamics
Reinforcement learning (RL), a subfield of machine learning, is proving to be a powerful tool in optimizing and predicting fluid dynamics.
RL involves training algorithms to make decisions through reward systems.
Over time, the algorithm learns optimal strategies by receiving feedback from the environment—a process that significantly enhances predictive accuracy.
Enhancing Prediction with Reinforcement Learning
Traditionally, simulating fluid dynamics scenarios relied on computational fluid dynamics (CFD) models.
While effective, CFD can be computationally expensive and time-consuming.
Reinforcement learning provides a promising alternative.
By employing RL algorithms, scientists can simulate numerous fluid scenarios rapidly, optimizing the processes by finding patterns and solutions that might not be apparent initially.
This approach also helps in learning complex boundary conditions and in fluid interactions that might be challenging or impossible to predict from a purely analytical standpoint.
Moreover, reinforcement learning models are being tailored to not only predict but also control fluid dynamics systems autonomously.
This development can lead to more efficient systems that adapt to real-time changes in fluid behavior, providing potential improvements in fields like robotics and autonomous vehicles.
Optimal Control through Reinforcement Learning
Optimal control refers to the process of optimizing system parameters to achieve the desired state in fluid systems, minimizing energy usage or maximizing output in technical applications.
Reinforcement learning excels in this arena by enabling systems to self-improve based on previous experiences and feedback, making the systems more resilient and adaptable.
Imagine the practical applications—using RL for optimal control in cooling systems for data centers can significantly reduce energy consumption while maintaining performance.
In aircraft design, RL can help in optimizing aerodynamic shapes to enhance fuel efficiency without compromising safety.
Furthermore, RL’s ability to accommodate unforeseen changes in system dynamics ensures that control strategies remain robust in the face of fluctuating environments, making it invaluable in an era of rapidly advancing technology and automation.
Challenges and Future Directions
Though promising, integrating reinforcement learning with fluid dynamics is not without its challenges.
One of the primary issues is the balance between precision and computational resources, as RL models can be complex and require significant amounts of data.
Another challenge is in the interpretability of RL solutions.
While an algorithm might find an effective solution to a fluid dynamic challenge, understanding the rationale behind the solution can be difficult.
This issue highlights the importance of coupling RL tools with domain expertise to bridge the gap between automated solutions and practical applications.
As technology evolves, new avenues for incorporating AI and machine learning in fluid dynamics are likely to emerge.
The future holds the promise of integrated systems where RL models work in tandem with traditional CFD approaches, offering greater efficiency and insights into complex fluid systems.
The Road Ahead
The collaboration between fluid dynamics and reinforcement learning is just beginning.
With continuous advancements in machine learning algorithms and computational power, the potential for development is vast.
Further research and development are needed to handle more complex dynamic systems and improve the precision and scalability of RL models.
As progress continues, the hope is that these integrated systems will lead to breakthroughs across multiple sectors, enhancing performance, efficiency, and sustainability.
In summary, the partnership between fluid dynamics and reinforcement learning is opening new possibilities for prediction and optimal control, offering powerful tools to tackle both current challenges and those yet to emerge.
資料ダウンロード
QCD管理受発注クラウド「newji」は、受発注部門で必要なQCD管理全てを備えた、現場特化型兼クラウド型の今世紀最高の受発注管理システムとなります。
ユーザー登録
受発注業務の効率化だけでなく、システムを導入することで、コスト削減や製品・資材のステータス可視化のほか、属人化していた受発注情報の共有化による内部不正防止や統制にも役立ちます。
NEWJI DX
製造業に特化したデジタルトランスフォーメーション(DX)の実現を目指す請負開発型のコンサルティングサービスです。AI、iPaaS、および先端の技術を駆使して、製造プロセスの効率化、業務効率化、チームワーク強化、コスト削減、品質向上を実現します。このサービスは、製造業の課題を深く理解し、それに対する最適なデジタルソリューションを提供することで、企業が持続的な成長とイノベーションを達成できるようサポートします。
製造業ニュース解説
製造業、主に購買・調達部門にお勤めの方々に向けた情報を配信しております。
新任の方やベテランの方、管理職を対象とした幅広いコンテンツをご用意しております。
お問い合わせ
コストダウンが利益に直結する術だと理解していても、なかなか前に進めることができない状況。そんな時は、newjiのコストダウン自動化機能で大きく利益貢献しよう!
(β版非公開)