- お役立ち記事
- For leaders of research and development departments! Analysis and interpretation of experimental data using Bayesian statistics
For leaders of research and development departments! Analysis and interpretation of experimental data using Bayesian statistics
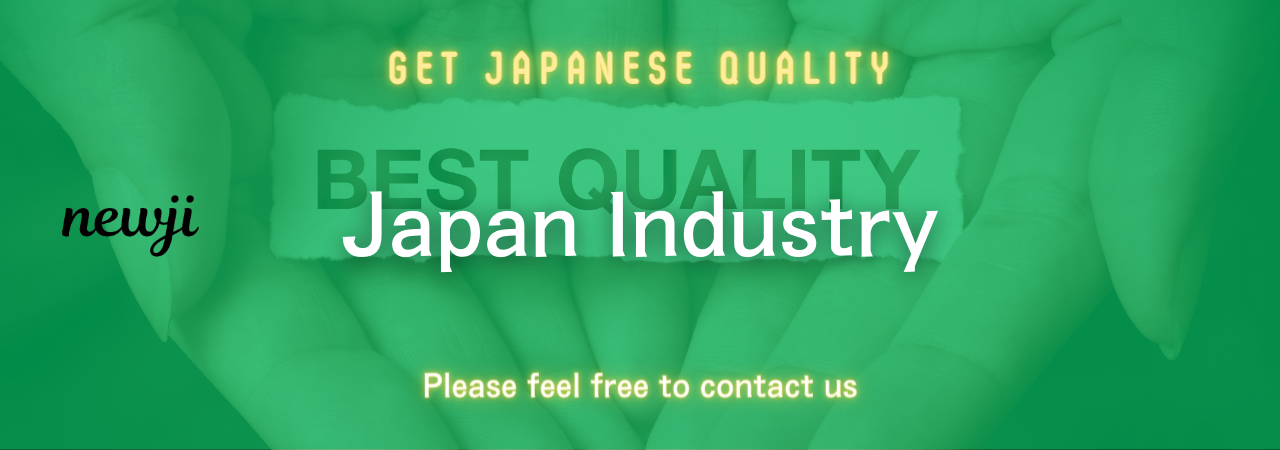
目次
Understanding Bayesian Statistics
Bayesian statistics is a powerful method that enables researchers to analyze and interpret experimental data more effectively.
This statistical approach provides a formalism for updating the probability of a hypothesis as more evidence or data becomes available.
It is based on Bayes’ theorem, which describes the probability of an event, based on prior knowledge of conditions that might be related to the event.
For leaders of research and development departments, understanding and applying Bayesian statistics can lead to more informed decision-making, better predictions, and more robust experimental designs.
Bayes’ Theorem: The Core Concept
At the heart of Bayesian statistics is Bayes’ Theorem, which can be expressed in the following formula:
P(A|B) = [P(B|A) * P(A)] / P(B)
This formula helps calculate conditional probabilities.
Here, P(A|B) is the probability of event A given that B is true.
P(B|A) is the probability of event B, given that A is true, P(A) and P(B) are the probabilities of observing A and B independently of each other.
Understanding this formula allows researchers to adjust their predictions or hypotheses based on new data.
This adaptability makes Bayesian methods particularly useful in fields where data is continually evolving, like healthcare, technology, and scientific research.
Bayesian vs. Frequentist Statistics
Before delving deeper into Bayesian statistics, it is essential to distinguish it from frequentist statistics, which is the traditional approach.
Frequentist statistics interpret probability as the long-term frequency of events.
It focuses on the likelihood of observing data given a fixed parameter but does not incorporate prior information.
In contrast, Bayesian statistics treats parameters as random variables with prior distributions.
This means it can incorporate prior knowledge or beliefs into the analysis, making it more flexible and applicable in situations where prior data or expert opinion is available.
Thus, while frequentist methods are rigid and often limited to current data, Bayesian approaches provide a richer framework for data analysis.
Application in R&D: Advantages of Bayesian Methods
Bayesian statistics offers several advantages that make it particularly appealing for research and development (R&D) departments:
1. **Incorporating Prior Knowledge:**
One of the key benefits of Bayesian analysis is its ability to incorporate pre-existing data or expert opinions.
In R&D, past experimental results or industry expertise can be used to build informative prior distributions, leading to more accurate and credible outcomes.
2. **Handling Complex Models:**
Bayesian methods excel in handling complex models that involve multiple variables and intricate relationships.
This can be particularly useful in experimental settings that involve numerous interacting components.
3. **Adapting to New Data:**
As new data becomes available, Bayesian analysis updates the model to reflect this.
This feature is invaluable in fields where experiments are ongoing and subject to change.
4. **Probabilistic Interpretation:**
Bayesian results are given as probability distributions, allowing for a more intuitive understanding of potential outcomes.
This probabilistic nature is key for decision-making under uncertainty, a common scenario in R&D projects.
5. **Flexibility and Robustness:**
Bayesian approaches can tackle challenges such as missing or small sample sizes, where traditional methods might struggle.
This flexibility enhances its robustness in generating meaningful inferences even when data conditions are less than ideal.
Implementing Bayesian Methods in R&D
For leaders looking to implement Bayesian statistics in their departments, several steps can be taken:
– **Training and Education:**
Educate team members on Bayesian principles and methodologies through workshops, seminars, or courses.
Understanding the foundational concepts is essential for effective application.
– **Software and Tools:**
Utilize statistical software such as R, Python, or specialized tools like WinBUGS and Stan, which can perform Bayesian analysis easily.
– **Collaborative Efforts:**
Engage with statisticians or data scientists who specialize in Bayesian methods.
Collaborations can help bridge knowledge gaps and facilitate a smoother integration into R&D processes.
– **Iterative Analysis:**
Encourage an iterative approach where models are continuously refined and adjusted as new data comes in.
This aligns with the Bayesian mindset of adaptation and refinement based on emerging evidence.
Challenges and Considerations
While Bayesian statistics offers many benefits, there are considerations to keep in mind:
– **Complexity:**
The methodology can be complex to understand and implement, with higher computational demands compared to frequentist methods.
– **Subjectivity:**
Priors are subjective and can introduce bias if not chosen carefully, but this also allows for the integration of expertise.
– **Resource Intensive:**
Bayesian analysis can be resource-intensive, requiring specialized software and skilled personnel.
Despite these challenges, the potential for insightful and innovative results makes Bayesian statistics a worthy investment for forward-thinking R&D teams.
Conclusion
Bayesian statistics is a pivotal tool for leaders in research and development aiming to enhance the interpretation and analysis of experimental data.
By enabling the incorporation of prior knowledge, handling complex models, and adapting to new information, it offers a powerful framework for navigating the complexities of modern R&D efforts.
Although challenges exist, with proper implementation and collaboration, Bayesian methods can significantly enhance the decision-making process, leading to robust and credible research outcomes.
Embracing this statistical perspective can position a department at the forefront of innovative and data-driven discovery.
資料ダウンロード
QCD調達購買管理クラウド「newji」は、調達購買部門で必要なQCD管理全てを備えた、現場特化型兼クラウド型の今世紀最高の購買管理システムとなります。
ユーザー登録
調達購買業務の効率化だけでなく、システムを導入することで、コスト削減や製品・資材のステータス可視化のほか、属人化していた購買情報の共有化による内部不正防止や統制にも役立ちます。
NEWJI DX
製造業に特化したデジタルトランスフォーメーション(DX)の実現を目指す請負開発型のコンサルティングサービスです。AI、iPaaS、および先端の技術を駆使して、製造プロセスの効率化、業務効率化、チームワーク強化、コスト削減、品質向上を実現します。このサービスは、製造業の課題を深く理解し、それに対する最適なデジタルソリューションを提供することで、企業が持続的な成長とイノベーションを達成できるようサポートします。
オンライン講座
製造業、主に購買・調達部門にお勤めの方々に向けた情報を配信しております。
新任の方やベテランの方、管理職を対象とした幅広いコンテンツをご用意しております。
お問い合わせ
コストダウンが利益に直結する術だと理解していても、なかなか前に進めることができない状況。そんな時は、newjiのコストダウン自動化機能で大きく利益貢献しよう!
(Β版非公開)