- お役立ち記事
- For managers of design departments in the automobile industry! How to optimize autonomous driving algorithms using artificial intelligence
For managers of design departments in the automobile industry! How to optimize autonomous driving algorithms using artificial intelligence
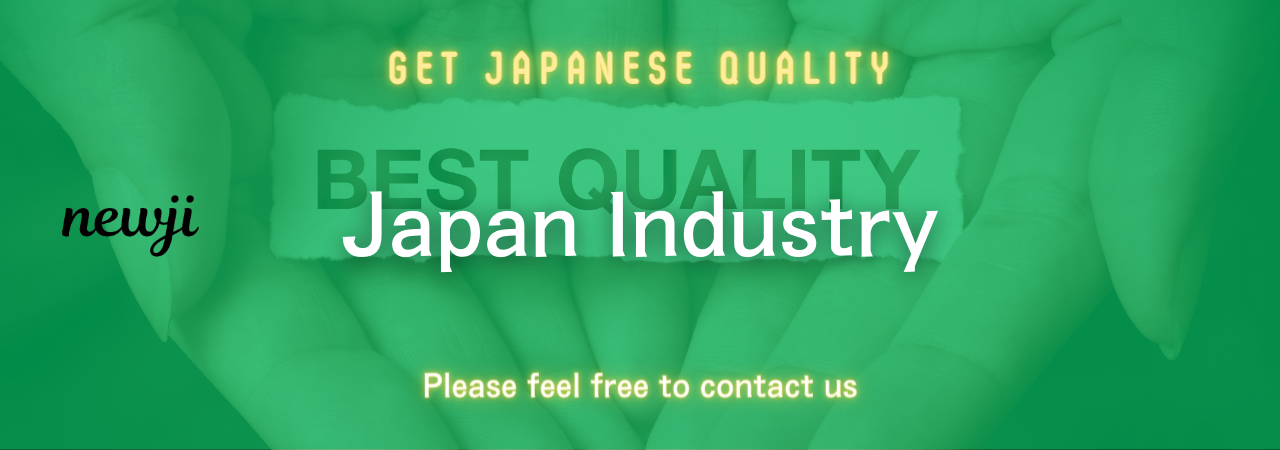
目次
Understanding Autonomous Driving Algorithms
The world of autonomous driving is rapidly evolving, with artificial intelligence playing a pivotal role in shaping how vehicles operate independently.
For managers in the design departments of the automobile industry, understanding how to optimize these algorithms can significantly enhance vehicle performance and safety.
Autonomous driving algorithms are essentially the brains behind a self-driving car, helping to make real-time decisions based on input from various sensors and data sources.
These algorithms rely on a combination of machine learning, computer vision, and sensor technologies to perceive their environment, plan routes, and execute driving maneuvers.
The goal is to mimic or exceed human driving capabilities by processing vast amounts of data quickly and accurately.
The Role of Artificial Intelligence in Autonomous Driving
Artificial intelligence is at the heart of autonomous driving technology.
AI systems are capable of handling complex tasks such as recognizing objects, predicting the actions of other road users, and making decisions that keep the car on the right path.
Through deep learning and neural networks, AI systems can continuously learn and improve from the data they collect, allowing for more refined decision-making processes.
AI not only interprets the data but also ensures that the vehicle can adapt to new and unforeseen conditions on the road.
By optimizing these AI-driven algorithms, automobiles can become safer, more efficient, and intelligent, offering a better overall driving experience.
Steps to Optimize Autonomous Driving Algorithms
Managers looking to enhance their design department’s approach to autonomous driving should consider several key steps in algorithm optimization.
Data Collection and Management
The foundation of an effective autonomous driving algorithm is data.
Tons of data from sensors like cameras, radar, and LIDAR need to be continuously gathered and efficiently managed.
Proper data labeling and classification are crucial for ensuring that the machine learning models can train without issues.
To streamline processes, invest in cloud-based data management systems that provide scalability and easy access.
Additionally, establishing a rigorous data validation routine can help in filtering out any anomalies or errors that could potentially corrupt the learning process.
Enhancing Machine Learning Models
Once a robust dataset is accumulated, the next step is to refine the machine learning models.
Enhancements in these models can lead to improved accuracy in object recognition, decision making, and environment mapping.
Experimenting with different algorithms, such as reinforcement learning or convolutional neural networks, can bring notable improvements.
Also, consider adopting transfer learning techniques to leverage existing models trained on similar tasks, saving time while improving the algorithm’s performance.
Regularly updating and retraining these models with new data ensures that they remain relevant and effective in real-world scenarios.
Simulation and Testing
Before deploying new algorithms into a vehicle, extensive testing in a simulated environment is vital.
Simulations offer a safe and controlled method to test how algorithms perform under various driving conditions without risking safety.
These virtual environments can replicate different weather conditions, road layouts, and traffic scenarios to assess the robustness of the algorithms.
Feedback from simulation tests is invaluable for identifying weaknesses and providing data-driven insights to help refine the algorithms further.
Collaborating with software developers who specialize in simulation technology can offer deeper insights and support in improving these neural networks.
Continuous Improvement and Collaboration
In the fast-evolving landscape of autonomous vehicles, continuous improvement is imperative.
Encouraging a culture of innovation and collaboration within design teams can drive significant advancements in algorithm development.
Cross-disciplinary teams that include software engineers, data scientists, and automotive experts can offer diverse perspectives and expertise.
Regular workshops, knowledge-sharing sessions, and hackathons can promote the exchange of ideas and motivate teams to challenge the status quo.
Keeping abreast of the latest industry trends and technological developments ensures that your team remains at the forefront of autonomous vehicle design.
The Importance of Safety and Ethics
Safety is a top priority when it comes to autonomous vehicle design.
Ethical considerations also play a crucial role in how algorithms make decisions, especially in scenarios where human lives could be impacted.
Ensuring that your algorithms prioritize safety without compromising ethical standards is essential.
Establishing guidelines and frameworks that your teams can reference when developing algorithms can foster safe and ethical practices.
By incorporating these standards, your team can develop autonomous systems that are reliable, trustworthy, and widely accepted by the public.
Conclusion
Optimizing autonomous driving algorithms with artificial intelligence is a multifaceted task requiring attention to data management, model enhancement, and rigorous testing.
For managers in the design departments of the automobile industry, embracing these practices can lead to significant improvements in vehicle autonomy.
By fostering a collaborative and innovative working environment, maintaining a focus on safety and ethics, and staying informed on technological advancements, managers can ensure their teams are well-equipped to lead in the autonomous vehicle revolution.
AI technology continues to offer exciting possibilities, and with strategic optimization, the future of self-driving cars is promising and bright.
資料ダウンロード
QCD調達購買管理クラウド「newji」は、調達購買部門で必要なQCD管理全てを備えた、現場特化型兼クラウド型の今世紀最高の購買管理システムとなります。
ユーザー登録
調達購買業務の効率化だけでなく、システムを導入することで、コスト削減や製品・資材のステータス可視化のほか、属人化していた購買情報の共有化による内部不正防止や統制にも役立ちます。
NEWJI DX
製造業に特化したデジタルトランスフォーメーション(DX)の実現を目指す請負開発型のコンサルティングサービスです。AI、iPaaS、および先端の技術を駆使して、製造プロセスの効率化、業務効率化、チームワーク強化、コスト削減、品質向上を実現します。このサービスは、製造業の課題を深く理解し、それに対する最適なデジタルソリューションを提供することで、企業が持続的な成長とイノベーションを達成できるようサポートします。
オンライン講座
製造業、主に購買・調達部門にお勤めの方々に向けた情報を配信しております。
新任の方やベテランの方、管理職を対象とした幅広いコンテンツをご用意しております。
お問い合わせ
コストダウンが利益に直結する術だと理解していても、なかなか前に進めることができない状況。そんな時は、newjiのコストダウン自動化機能で大きく利益貢献しよう!
(Β版非公開)