- お役立ち記事
- Fracture surface analysis using the basics of material mechanics/fatigue strength and machine learning
Fracture surface analysis using the basics of material mechanics/fatigue strength and machine learning
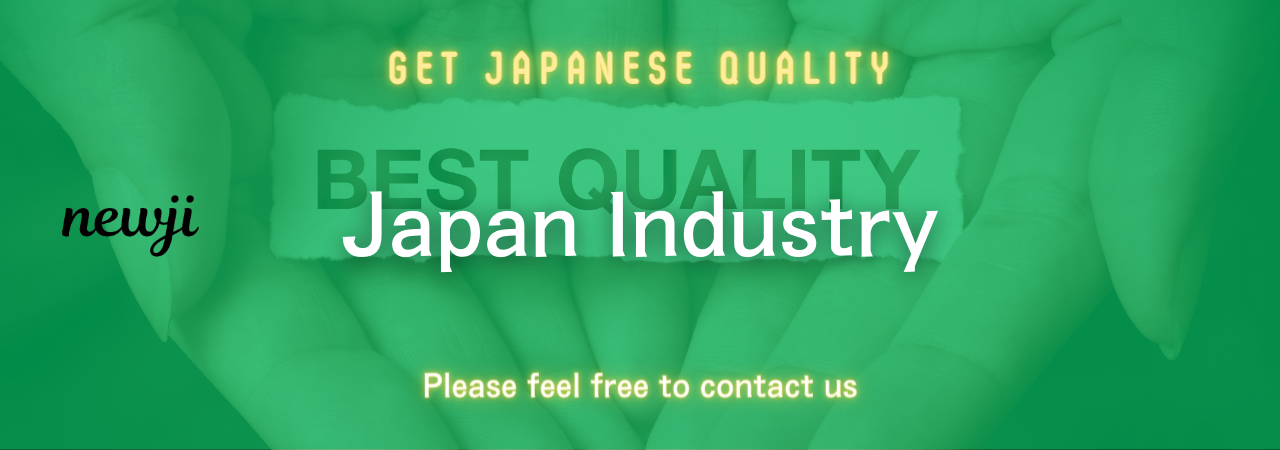
Fracture surface analysis is a critical aspect of understanding material behavior and enhancing fatigue strength in engineering applications.
In recent years, advancements in machine learning have revolutionized the approach to fracture surface analysis, providing more accurate and reliable insights.
Leveraging the fundamentals of material mechanics, researchers and engineers are now better equipped to predict and improve the fatigue strength of materials across various industries.
目次
Understanding Material Mechanics and Fatigue Strength
Material mechanics is the study of materials’ behavior under various forces and conditions.
It involves understanding how materials deform, break, or fail under stress and strain.
Fatigue strength, a key concept within material mechanics, refers to a material’s ability to withstand repeated loading and unloading cycles without failing.
This is crucial for components that experience fluctuating forces during their service life, such as in automotive, aerospace, and structural engineering sectors.
Fatigue failure is a progressive structural damage that occurs when a material is subjected to cyclic loading.
It usually begins with a small crack at the material’s surface, propagating gradually with continued stress, leading to eventual fracture.
Understanding and predicting this process is vital to enhance component longevity and safety.
The Role of Fracture Surface Analysis
Fracture surface analysis is a technique used to examine the surface where a material has fractured.
By studying the fracture surfaces, engineers can gain insights into the causes of failure, the mode of fracture, and the conditions under which the failure occurred.
Typical analysis involves observations with optical microscopes, scanning electron microscopes (SEM), and other advanced imaging techniques to assess the topography and features of the fracture surface.
This analysis helps identify whether the fracture was brittle or ductile, fatigue-induced, or resulted from other failure modes.
Information gleaned from fracture surface analysis is invaluable for diagnosing failure causes and improving design strategies to enhance fatigue strength.
Machine Learning in Fracture Surface Analysis
Machine learning (ML), a subset of artificial intelligence, brings a transformative approach to analyzing fracture surfaces.
ML algorithms can process vast amounts of data, identify patterns, and make predictions far more efficiently than traditional methods.
Integrating ML with material mechanics allows for a more precise evaluation of fatigue strength and fracture characteristics.
Techniques and Applications
One application of machine learning in fracture surface analysis is image recognition.
ML algorithms can be trained to recognize and categorize fracture surface features from microscopic images.
These algorithms learn to distinguish between different fracture modes, such as brittle, ductile, or fatigue-induced fractures, based on the unique patterns and textures identified in the images.
Deep learning, a more advanced branch of ML, enhances this process by utilizing neural networks for automated feature extraction and classification.
This eliminates the need for extensive manual input and potential human error, facilitating faster and more accurate analysis.
Another ML application is predictive modeling of fatigue life.
By analyzing historical material data, ML models can predict the fatigue life of materials under specific conditions.
Such models help in selecting materials and designing components with optimized fatigue strength for specific applications.
Benefits of Machine Learning in Fracture Analysis
The integration of machine learning offers several benefits to fracture surface analysis:
1. **Increased Accuracy and Consistency**: ML algorithms provide consistent analysis, reducing the variability inherent in human interpretation.
2. **Enhanced Predictive Capability**: By leveraging historical data, ML models can accurately predict material behavior under varying loads and conditions.
3. **Time and Cost Efficiency**: Automated analysis reduces the time and cost associated with traditional manual methods.
4. **Data-Driven Insights**: ML provides valuable insights that can lead to the discovery of new materials and improved design strategies.
The Future of Fracture Surface Analysis with ML
The convergence of material mechanics, fracture surface analysis, and machine learning heralds a new era of material science and engineering.
This synergy promises significant advancements in fatigue strength and material performance.
As machine learning algorithms continue to evolve, they will offer even more sophisticated analysis techniques, such as multi-modal data processing.
This involves integrating different types of data, like thermal, mechanical, and microstructural, to provide a comprehensive understanding of material behavior.
Moreover, with the increasing adoption of ML, industries will be able to implement real-time monitoring systems for components in service.
Such systems will predict potential failures before they occur, allowing for proactive maintenance, enhancing safety, and reducing downtime.
In academia, educational programs are increasingly incorporating machine learning into engineering curriculum, preparing future engineers to leverage these tools in fracture analysis and material design.
Conclusion
Fracture surface analysis is essential for understanding material failure and improving fatigue strength.
The application of machine learning in this domain enhances the efficiency, accuracy, and predictive power of analysis, leading to better material performance and safety standards.
As industries continue to harness the power of machine learning, the opportunities for innovation and improvement in material mechanics and fatigue analysis are boundless.
By embracing these advancements, engineers and researchers can push the boundaries of what is possible in material science, leading to more robust and reliable engineering solutions.
資料ダウンロード
QCD調達購買管理クラウド「newji」は、調達購買部門で必要なQCD管理全てを備えた、現場特化型兼クラウド型の今世紀最高の購買管理システムとなります。
ユーザー登録
調達購買業務の効率化だけでなく、システムを導入することで、コスト削減や製品・資材のステータス可視化のほか、属人化していた購買情報の共有化による内部不正防止や統制にも役立ちます。
NEWJI DX
製造業に特化したデジタルトランスフォーメーション(DX)の実現を目指す請負開発型のコンサルティングサービスです。AI、iPaaS、および先端の技術を駆使して、製造プロセスの効率化、業務効率化、チームワーク強化、コスト削減、品質向上を実現します。このサービスは、製造業の課題を深く理解し、それに対する最適なデジタルソリューションを提供することで、企業が持続的な成長とイノベーションを達成できるようサポートします。
オンライン講座
製造業、主に購買・調達部門にお勤めの方々に向けた情報を配信しております。
新任の方やベテランの方、管理職を対象とした幅広いコンテンツをご用意しております。
お問い合わせ
コストダウンが利益に直結する術だと理解していても、なかなか前に進めることができない状況。そんな時は、newjiのコストダウン自動化機能で大きく利益貢献しよう!
(Β版非公開)