- お役立ち記事
- Fundamentals and applications of data-driven prediction and control (FRIT to V-Tiger)
月間77,185名の
製造業ご担当者様が閲覧しています*
*2025年2月28日現在のGoogle Analyticsのデータより
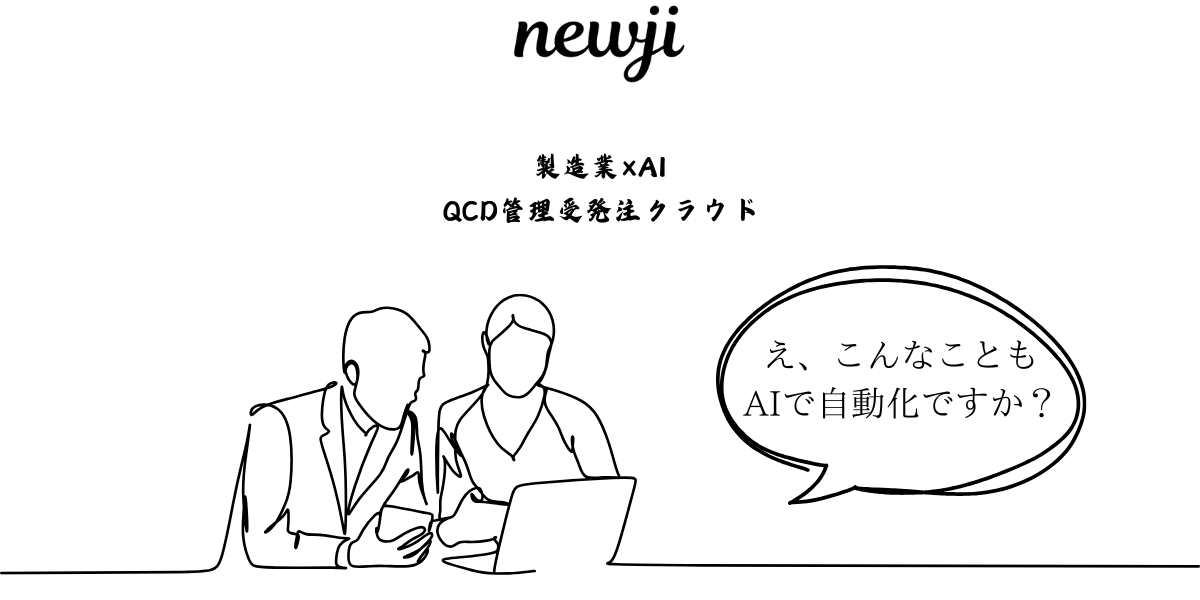
Fundamentals and applications of data-driven prediction and control (FRIT to V-Tiger)
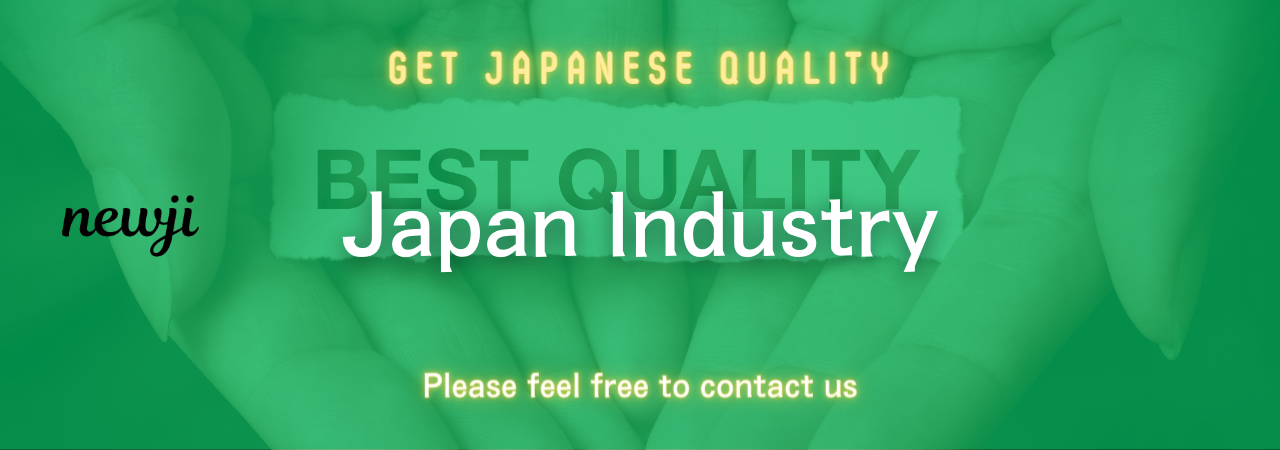
目次
Understanding Data-Driven Prediction and Control
Data-driven prediction and control refer to techniques that utilize data to predict future outcomes and control systems accordingly.
The importance of data in today’s technology-driven world cannot be overstated.
With the vast amount of data generated daily, the ability to harness and analyze this information has become crucial in various fields.
From finance to healthcare, data-driven methods offer significant advantages in making informed decisions.
The Basics of Data-Driven Prediction
Data-driven prediction involves using historical data to forecast future events or outcomes.
The process typically relies on statistical algorithms and machine learning models.
These models are trained on existing data to identify patterns, relationships, and trends that can help predict future events.
For instance, in weather forecasting, historical climate data is used to predict future weather conditions.
There are several key steps involved in creating data-driven predictive models:
1. **Data Collection**: Gathering relevant data is the first step.
This could include numerical data, text, images, or other forms of data that could impact future predictions.
2. **Data Preprocessing**: Preparing the data is crucial.
This may involve cleaning the data, handling missing values, and transforming it into a format suitable for analysis.
3. **Model Selection**: Choosing the right model is pivotal.
Depending on the nature of the data and the desired outcome, different algorithms might be more applicable.
4. **Training and Validation**: Training involves feeding the model with data and adjusting it to minimize the prediction error.
Validation helps in assessing the model’s accuracy and performance on unseen data.
5. **Deployment and Monitoring**: Once the model is developed, it is deployed for real-world use.
Continuous monitoring ensures the model maintains its accuracy over time, adjusting for changes in data patterns.
Applications of Data-Driven Prediction
The scope of data-driven prediction is vast, and its applications are evident in multiple domains:
Healthcare
In healthcare, predictive models can anticipate patient needs, improving preventative care and reducing costs.
For example, predicting patient admission rates can help hospitals manage their resources better, ensuring that necessary staff and facilities are available.
Finance
Financial institutions leverage data-driven prediction for risk management and fraud detection.
By analyzing transaction patterns, they can predict fraudulent actions and take preventative measures accordingly.
Retail and Marketing
Retailers use predictive analytics to tailor marketing strategies based on customer behavior.
Understanding what consumers are likely to purchase enables businesses to create personalized shopping experiences.
Manufacturing
In manufacturing, predictive maintenance is a significant application.
By forecasting equipment failures before they occur, companies can reduce downtime and save costs associated with unexpected maintenance.
Diving Into Data-Driven Control
While prediction focuses on anticipating future events, data-driven control is about taking action based on predictions.
This involves automating decisions to achieve desired outcomes.
The process is heavily dependent on real-time data and feedback systems.
Key Components of Data-Driven Control
1. **Data Acquisition**: Continuously collecting data in real-time is essential for effective control.
This includes sensory data, environmental conditions, and user inputs.
2. **Decision-Making Algorithms**: These algorithms determine the best course of action based on current data and predicted outcomes.
The complexity of these algorithms can range from simple rule-based systems to advanced artificial intelligence.
3. **Actuation**: The system must be able to execute decisions.
This could involve mechanical responses, alerts, or even changes in a software environment.
4. **Feedback Loops**: Continuous feedback allows the system to evaluate its performance and make necessary adjustments.
This adaptability is crucial for maintaining efficient control under dynamic conditions.
Applications of Data-Driven Control
The impact of data-driven control is visible in various sectors, enhancing efficiency and effectiveness:
Automotive Industry
In modern automobiles, data-driven control systems automate functions like adaptive cruise control and lane-keeping assistance.
These systems rely on real-time data from sensors to make driving safer and more comfortable.
Smart Homes
Home automation systems use data-driven control to manage lighting, heating, and security systems.
These systems adjust based on user habits and preferences, enhancing energy efficiency and comfort.
Industrial Automation
In factories, data-driven control systems optimize production lines, reducing waste and improving quality.
By adjusting machinery operations in real-time, companies can achieve higher productivity levels.
Challenges and Future Prospects
Despite the evident benefits, implementing data-driven prediction and control systems is not without challenges.
Data privacy and security concerns are paramount, as these systems often handle sensitive information.
Additionally, the quality and accuracy of predictions depend heavily on the availability and integrity of data.
Looking toward the future, advances in AI and machine learning offer promising prospects for enhancing these systems.
The integration of the Internet of Things (IoT) is likely to play a significant role, providing more data sources and enabling smarter, more responsive control mechanisms.
As technology continues to evolve, the potential for data-driven prediction and control will only expand.
Understanding these concepts and their applications can provide a competitive advantage across various fields, driving innovation and efficiency in unprecedented ways.
資料ダウンロード
QCD管理受発注クラウド「newji」は、受発注部門で必要なQCD管理全てを備えた、現場特化型兼クラウド型の今世紀最高の受発注管理システムとなります。
ユーザー登録
受発注業務の効率化だけでなく、システムを導入することで、コスト削減や製品・資材のステータス可視化のほか、属人化していた受発注情報の共有化による内部不正防止や統制にも役立ちます。
NEWJI DX
製造業に特化したデジタルトランスフォーメーション(DX)の実現を目指す請負開発型のコンサルティングサービスです。AI、iPaaS、および先端の技術を駆使して、製造プロセスの効率化、業務効率化、チームワーク強化、コスト削減、品質向上を実現します。このサービスは、製造業の課題を深く理解し、それに対する最適なデジタルソリューションを提供することで、企業が持続的な成長とイノベーションを達成できるようサポートします。
製造業ニュース解説
製造業、主に購買・調達部門にお勤めの方々に向けた情報を配信しております。
新任の方やベテランの方、管理職を対象とした幅広いコンテンツをご用意しております。
お問い合わせ
コストダウンが利益に直結する術だと理解していても、なかなか前に進めることができない状況。そんな時は、newjiのコストダウン自動化機能で大きく利益貢献しよう!
(β版非公開)