- お役立ち記事
- Fundamentals and implementation of deep learning and applied programming using Python
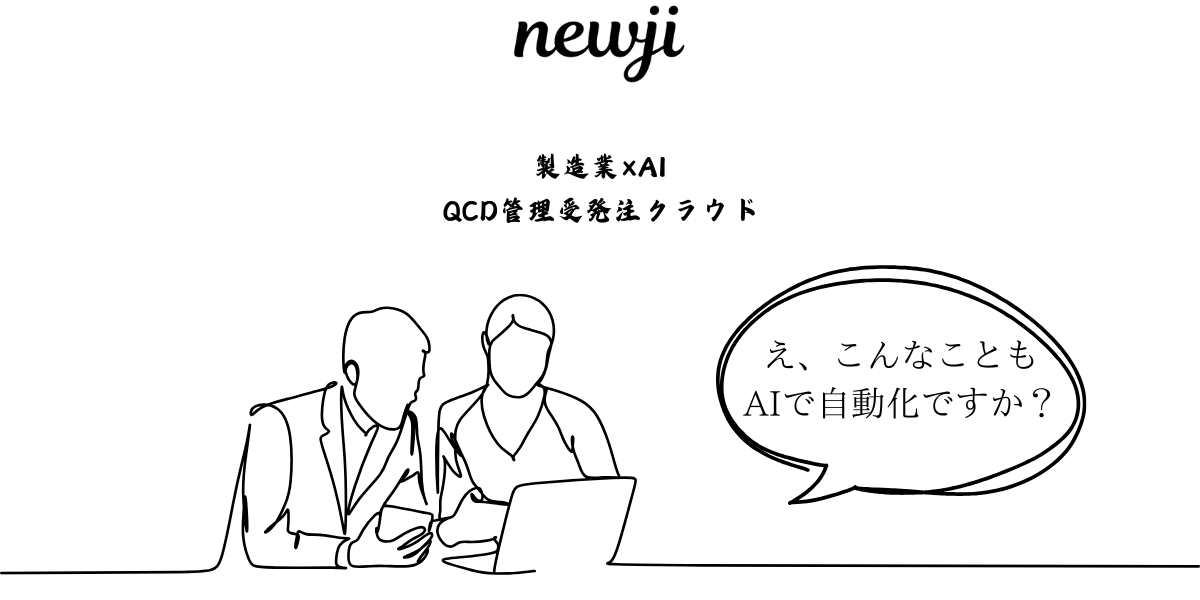
Fundamentals and implementation of deep learning and applied programming using Python
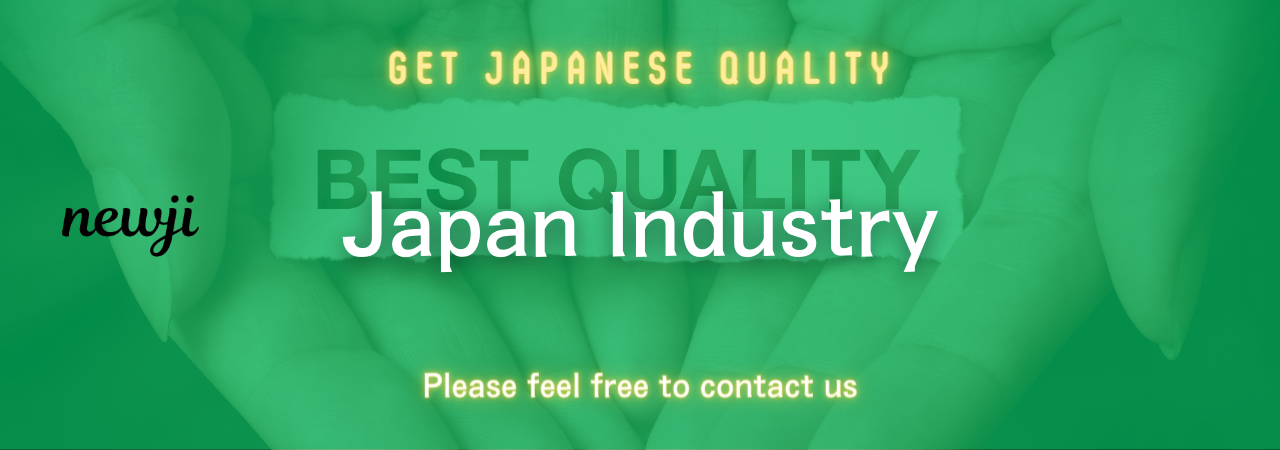
目次
Understanding Deep Learning
Deep learning is a subset of machine learning that has gained significant attention in recent years due to its ability to process and analyze vast amounts of data with remarkable accuracy.
At its core, deep learning involves the use of neural networks, which are computational models designed to mimic the human brain’s way of processing information.
These neural networks consist of layers of interconnected nodes, or “neurons,” that work together to recognize patterns and make decisions based on input data.
The Basics of Neural Networks
Neural networks are structured in layers: an input layer, one or more hidden layers, and an output layer.
The input layer receives the raw data.
The hidden layers process the data through weighted connections, and the output layer generates the final prediction or classification.
Each node in a layer is connected to every node in the next layer, allowing for complex information processing.
The power of deep learning lies in its multi-layered architecture, which enables the model to learn intricate patterns and representations in the data.
Training Neural Networks
Training a neural network involves feeding it a large amount of labeled data and adjusting the weights of the connections between nodes to minimize prediction errors.
This process is called backpropagation, where the model analyzes the difference between the predicted output and the actual output.
The weights are then adjusted to reduce this error.
Through repeated iterations, or epochs, the model gradually improves its accuracy and becomes more adept at recognizing patterns in the data.
Applications of Deep Learning
Deep learning has a wide range of applications across various industries due to its ability to process complex and high-dimensional data.
In healthcare, deep learning models are used to analyze medical images, enabling the early detection of diseases such as cancer.
In the automotive industry, deep learning powers self-driving cars, allowing them to interpret real-time data from sensors and make decisions on the road.
In finance, deep learning is employed for fraud detection and algorithmic trading, helping to identify suspicious activities and optimize investment strategies.
Natural Language Processing (NLP)
One of the most exciting applications of deep learning is in natural language processing (NLP), where models are trained to understand and generate human language.
NLP technologies are used in virtual assistants like Siri and Alexa, enabling them to understand and respond to voice commands.
Deep learning models can also translate text, perform sentiment analysis, and even generate human-like text, as seen in applications like chatbots and content creation.
Computer Vision
Computer vision is another prominent area where deep learning excels.
It involves the development of models that can process and analyze visual data, such as images and videos.
Deep learning algorithms are used in facial recognition systems, object detection, and image classification.
These models are capable of recognizing faces in a crowd, identifying objects in real-time, and accurately classifying images based on their contents.
Implementing Deep Learning with Python
Python is one of the most popular programming languages for developing deep learning applications due to its simplicity and the vast array of libraries and frameworks available.
Frameworks like TensorFlow, PyTorch, and Keras provide easy-to-use interfaces for building and deploying deep learning models.
Setting Up Your Environment
To start with deep learning in Python, you’ll need to set up your development environment.
This typically involves installing Python along with packages such as NumPy, pandas, and Matplotlib for data manipulation and visualization.
Additionally, you’ll need a deep learning framework like TensorFlow or PyTorch.
These frameworks provide pre-built functions and features that simplify the process of creating and training deep learning models.
Building a Simple Neural Network
Once your environment is set up, you can begin building a simple neural network.
Start by importing the necessary libraries and preparing your dataset.
For simplicity, we’ll use a dataset from a pre-existing library like scikit-learn.
The next step is to define the architecture of your neural network, specifying the number of layers and nodes in each layer.
You’ll then compile your model, specifying the optimizer and loss function to be used during training.
Training and Evaluating the Model
With your model defined, you can proceed to training.
Feed the model your dataset, specify the number of epochs, and let the training process begin.
The framework will automatically handle the backpropagation and weight updates for you.
Once training is complete, evaluate the model’s performance using a separate test set to ensure it can generalize to new data.
Fine-tune the model by adjusting hyperparameters or adding more layers to improve its accuracy and robustness.
Challenges and Future Directions
Despite its successes, deep learning still faces several challenges, such as the need for large amounts of labeled data and significant computational resources.
Moreover, deep learning models are often seen as “black boxes” due to their lack of interpretability, making it difficult to understand how they arrive at certain decisions.
Researchers are actively working on developing techniques to address these issues, such as transfer learning, which allows models to leverage knowledge from related tasks, and model interpretability methods that provide insights into the decision-making process.
Looking ahead, the future of deep learning is promising.
As computational power increases and more sophisticated algorithms are developed, we can expect even more groundbreaking applications in areas like personalized medicine, autonomous systems, and climate modeling, to name a few.
Deep learning has the potential to revolutionize how we interact with technology and solve complex problems, making it an exciting field with endless possibilities.
資料ダウンロード
QCD調達購買管理クラウド「newji」は、調達購買部門で必要なQCD管理全てを備えた、現場特化型兼クラウド型の今世紀最高の購買管理システムとなります。
ユーザー登録
調達購買業務の効率化だけでなく、システムを導入することで、コスト削減や製品・資材のステータス可視化のほか、属人化していた購買情報の共有化による内部不正防止や統制にも役立ちます。
NEWJI DX
製造業に特化したデジタルトランスフォーメーション(DX)の実現を目指す請負開発型のコンサルティングサービスです。AI、iPaaS、および先端の技術を駆使して、製造プロセスの効率化、業務効率化、チームワーク強化、コスト削減、品質向上を実現します。このサービスは、製造業の課題を深く理解し、それに対する最適なデジタルソリューションを提供することで、企業が持続的な成長とイノベーションを達成できるようサポートします。
オンライン講座
製造業、主に購買・調達部門にお勤めの方々に向けた情報を配信しております。
新任の方やベテランの方、管理職を対象とした幅広いコンテンツをご用意しております。
お問い合わせ
コストダウンが利益に直結する術だと理解していても、なかなか前に進めることができない状況。そんな時は、newjiのコストダウン自動化機能で大きく利益貢献しよう!
(Β版非公開)