- お役立ち記事
- Fundamentals and implementation of GAN (Generative Adversarial Network) and application to system development
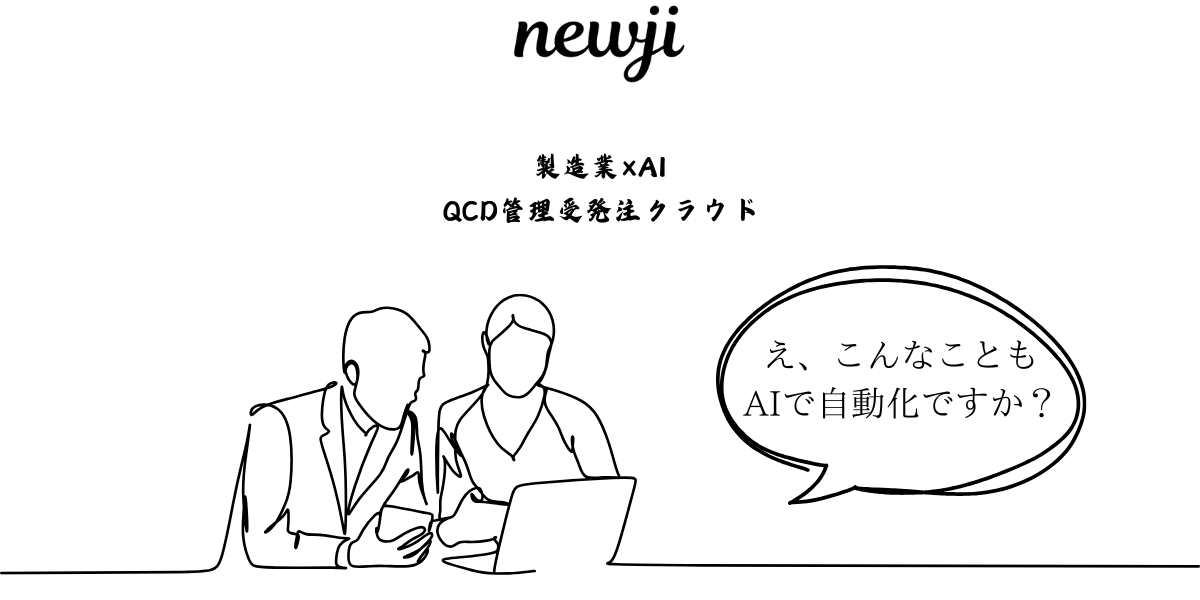
Fundamentals and implementation of GAN (Generative Adversarial Network) and application to system development
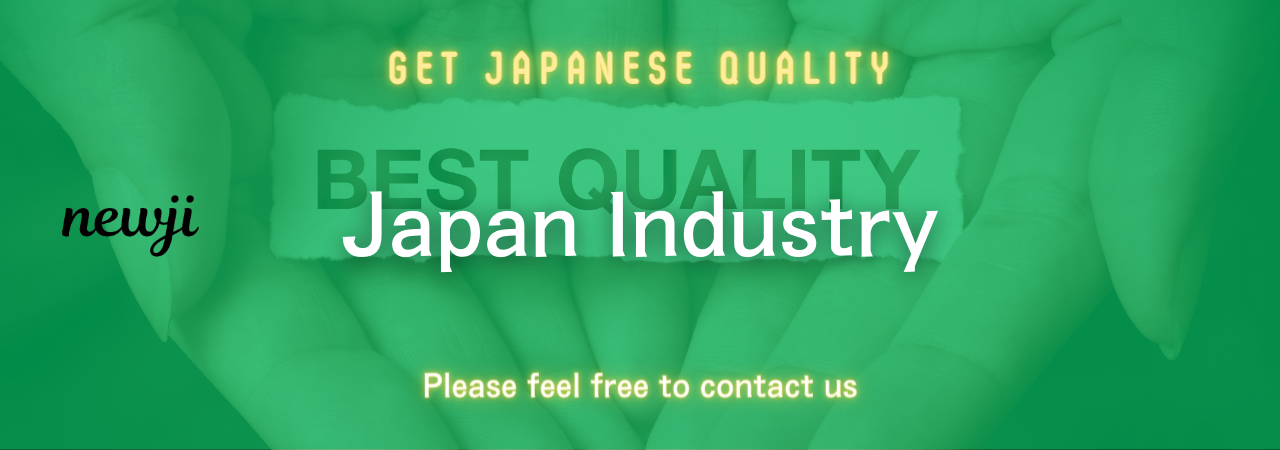
目次
Introduction to Generative Adversarial Networks (GANs)
Generative Adversarial Networks, commonly known as GANs, have become a pillar in the field of machine learning and artificial intelligence.
These networks are capable of generating data that mimics real-world data, making them a powerful tool for various applications.
Developed by Ian Goodfellow and his colleagues in 2014, GANs have seen rapid advancements and diversification in their applications.
The fundamental concept behind GANs is the interaction between two neural networks — the generator and the discriminator.
These two networks are set up in a continuous and competitive process aiming for improvement.
This introduction will explore the fundamentals and implementation of GANs, as well as their application to system development.
The Basic Structure of GAN
GANs are built on a relatively simple yet profoundly effective structure consisting of two primary components: the generator and the discriminator.
The Generator
The generator’s role is to create data.
It begins by taking in random noise and transforming it into data instances that mimic real-world data.
The goal for the generator is not only to produce data that resembles real data but also to constantly improve its output to fool the discriminator.
The generator learns and refines the data it produces by understanding the weaknesses identified by the discriminator.
The Discriminator
On the other hand, the discriminator serves as a watchdog, evaluating the data created by the generator along with actual data from the real world.
Its task is to distinguish between real and synthetic data, acting as a binary classifier.
The discriminator sends back feedback to the generator, which helps the generator improve its output.
This adversarial process between generator and discriminator continues, leading to enhanced data generation quality.
Training Process of GAN
The training process of a GAN can be perceived as a minimax game where the generator aims to maximize the probability of the discriminator making a mistake, while the discriminator seeks to minimize its error rate.
Step 1: Initialization
Initially, both the generator and the discriminator are randomly initialized.
This means they start without any idea of what they should produce or discriminate.
Step 2: Discriminator Training
During this step, the discriminator is trained using both genuine data and falsely generated data.
Its primary goal is to differentiate between the two and minimize the classification error.
Step 3: Generator Training
Once the discriminator reaches a level of accuracy, the generator is trained to maximize the error rate of the discriminator.
Through backpropagation and optimization, the generator adjusts its parameters to produce more convincing data.
Step 4: Iterative Improvement
The generator and discriminator are trained alternately.
This iterative cycle continues until the generator produces high-quality synthetic data that the discriminator cannot easily distinguish from real data.
Applications of GANs in System Development
The extensive capabilities of GANs find applications across various domains, influencing how systems are developed and optimized.
Image Generation
One of the most common applications of GANs is in image generation.
GANs can create high-quality and realistic images, which have applications in areas such as entertainment, art, and design.
They aid in tasks like developing video game environments or creating movie graphics.
Data Augmentation
GANs are effective for data augmentation, particularly in situations where collecting data is expensive or challenging.
By generating synthetic data, GANs can supplement existing datasets, enhancing performance for machine learning models.
This application is valuable in fields like medical imaging, where annotated data may be limited.
Style Transfer and Enhancement
In the realm of photography and videography, GANs are used for style transfer and image enhancement.
They can modify images by transferring the style of a specific artwork onto a photograph, offering creative tools for artists.
GANs also improve image resolution, helping in upscaling images without losing quality.
Text-to-Image Synthesis
GANs facilitate text-to-image synthesis, where they are employed to create images based on textual descriptions.
This technology has implications in improving accessibility and creating visual content from text in varied industries.
Challenges and Considerations
Despite their innovative capabilities, GANs present specific challenges and considerations.
Training Instability
Training GANs can be unstable, often oscillating between outcomes without reaching a convergence.
The delicate competition between the generator and discriminator can lead to situations where one network dominates the other, hindering progress.
Mode Collapse
Mode collapse is another challenge where the generator produces limited diverse outputs, restricting the range of data it generates.
Improving diversity and coverage in outputs remains an ongoing research focus.
Resource Intensive
Implementing GANs requires substantial computational power and resources.
Given their complex interactions and high-dimensional data processing, they necessitate significant support in terms of hardware and time.
Conclusion
Generative Adversarial Networks have revolutionized how we think about data generation and system development.
From creating realistic images to enhancing datasets, their applications continue to grow and evolve.
Despite challenges in training and implementation, GANs offer vibrant opportunities to innovate within various industries.
As research progresses, GANs are poised to become even more integrated into the future of artificial intelligence and system development.
資料ダウンロード
QCD調達購買管理クラウド「newji」は、調達購買部門で必要なQCD管理全てを備えた、現場特化型兼クラウド型の今世紀最高の購買管理システムとなります。
ユーザー登録
調達購買業務の効率化だけでなく、システムを導入することで、コスト削減や製品・資材のステータス可視化のほか、属人化していた購買情報の共有化による内部不正防止や統制にも役立ちます。
NEWJI DX
製造業に特化したデジタルトランスフォーメーション(DX)の実現を目指す請負開発型のコンサルティングサービスです。AI、iPaaS、および先端の技術を駆使して、製造プロセスの効率化、業務効率化、チームワーク強化、コスト削減、品質向上を実現します。このサービスは、製造業の課題を深く理解し、それに対する最適なデジタルソリューションを提供することで、企業が持続的な成長とイノベーションを達成できるようサポートします。
オンライン講座
製造業、主に購買・調達部門にお勤めの方々に向けた情報を配信しております。
新任の方やベテランの方、管理職を対象とした幅広いコンテンツをご用意しております。
お問い合わせ
コストダウンが利益に直結する術だと理解していても、なかなか前に進めることができない状況。そんな時は、newjiのコストダウン自動化機能で大きく利益貢献しよう!
(Β版非公開)