- お役立ち記事
- Fundamentals and implementation of image processing/image recognition technology and application to automation systems
Fundamentals and implementation of image processing/image recognition technology and application to automation systems
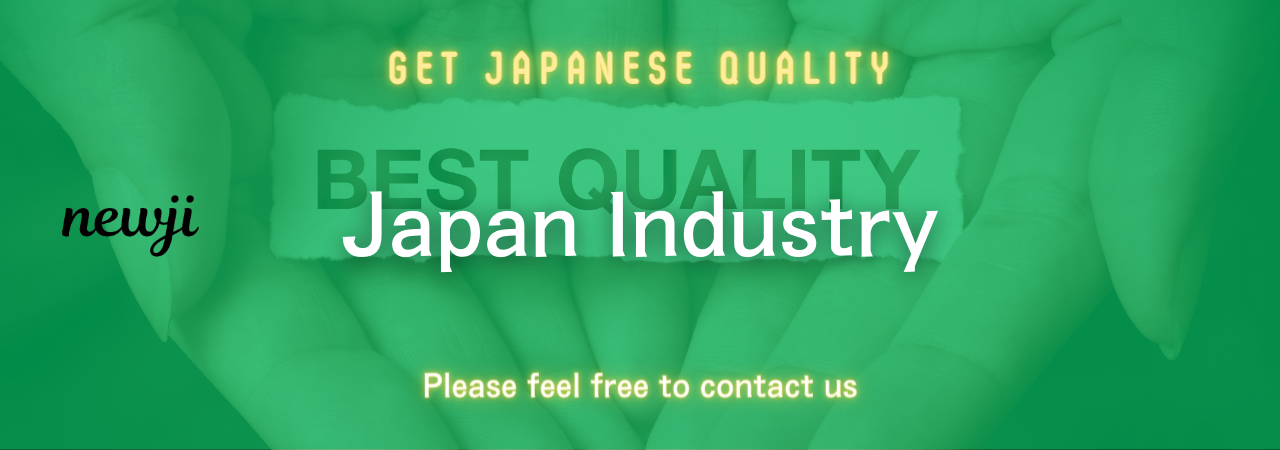
目次
Understanding Image Processing and Image Recognition
Image processing and image recognition are interconnected technologies widely used in various applications today.
While image processing refers to the manipulation of images to enhance or extract information, image recognition involves identifying and classifying objects within these images.
Both technologies have advanced significantly, enabling numerous practical applications and revolutionizing sectors like healthcare, security, and automotive industries.
Basics of Image Processing
In its essence, image processing involves a series of techniques applied to digital images to perform various operations.
These operations may include improving the visual appearance of an image, extracting important features, or converting images into a format suitable for analysis.
The process begins by acquiring an image through devices like cameras or sensors.
Once the image is obtained, image enhancement techniques such as contrast adjustment or noise reduction can be applied to improve quality.
Image segmentation then follows, dividing the image into parts or regions for easier analysis.
Advanced processes like edge detection and filtering further refine the image by identifying distinct boundaries and removing unwanted elements, respectively.
Finally, feature extraction focuses on identifying specific patterns in the image, which are critical for interpretation and analysis.
Image Recognition: How It Works
Image recognition is the logical continuation of image processing.
With the refined image data, image recognition systems can classify and label objects within the image.
This technology utilizes machine learning algorithms, especially neural networks, to process the extracted features and compare them to a vast database of known patterns.
The model, once trained, can recognize objects with a certain level of accuracy and confidence.
Deep learning models like Convolutional Neural Networks (CNNs) are particularly successful in image recognition tasks due to their capability to handle large datasets and their architectural design that mimics the human brain’s processing.
Applications in Automation Systems
The integration of image processing and recognition technologies into automation systems has driven significant advancements across numerous industries.
Here are some key areas where these technologies are making a difference:
Healthcare
In healthcare, image processing and recognition are crucial in medical imaging techniques such as X-rays, MRIs, and CT scans.
These technologies assist in diagnosing diseases by enhancing image quality and automatically detecting abnormalities.
Automation systems equipped with image recognition can thus reduce diagnostic errors and expedite the examination process, benefiting both healthcare providers and patients.
Security and Surveillance
Security systems have substantially improved with the implementation of image processing and recognition.
These systems are capable of real-time monitoring and identifying suspicious activities or individuals.
Facial recognition technology, a subset of image recognition, has been instrumental in enhancing security protocols in sensitive areas such as airports and public events.
Automotive Industry
In the automotive sector, image processing and recognition play pivotal roles in the development of autonomous vehicles.
These technologies allow vehicles to “see” and understand their environment, recognizing traffic signals, obstacles, and pedestrians.
Automated systems rely heavily on these capabilities to navigate roads safely and efficiently.
Manufacturing and Quality Control
Automation systems in manufacturing utilize image processing for quality control and inspection processes.
Products are scanned and analyzed to detect defects or inconsistencies, ensuring high standards of quality are maintained.
This not only enhances efficiency but also reduces waste and production costs.
Implementing Image Processing and Recognition
Implementing these technologies involves both hardware and software components.
On the hardware side, high-resolution cameras and sensors are integral for capturing detailed images.
On the software side, robust algorithms and models are developed to process and analyze image data.
The implementation process begins with data collection, during which a diverse set of images is gathered to train machine learning models.
Once the models are trained, they are integrated into automation systems for real-time processing and decision-making.
Developers must consider several factors, such as computational power and data privacy.
High computational capacity is needed to manage the vast amount of data and complex algorithms used in image recognition tasks.
Simultaneously, data privacy regulations must be adhered to, ensuring that sensitive information is protected throughout the processing and recognition steps.
Challenges and Future Prospects
Despite the significant advancements, challenges remain in the implementation of image processing and recognition technologies.
Issues like image resolution, lighting conditions, and object occlusion can affect the accuracy and reliability of these systems.
Furthermore, ethical considerations, especially concerning surveillance and data privacy, pose additional challenges.
Balancing technological development with societal norms and privacy rights is crucial for the responsible deployment of these technologies.
Looking to the future, ongoing research and development promise even more sophisticated and capable systems.
Innovations in hardware, computing power, and algorithmic approaches will likely overcome current limitations, paving the way for broader applications.
Image processing and recognition are set to further intertwine with artificial intelligence, leading to smarter and more autonomous systems that could transform our daily lives and work processes.
The prospects for these technologies are immense, with potential applications we have yet to imagine.
資料ダウンロード
QCD調達購買管理クラウド「newji」は、調達購買部門で必要なQCD管理全てを備えた、現場特化型兼クラウド型の今世紀最高の購買管理システムとなります。
ユーザー登録
調達購買業務の効率化だけでなく、システムを導入することで、コスト削減や製品・資材のステータス可視化のほか、属人化していた購買情報の共有化による内部不正防止や統制にも役立ちます。
NEWJI DX
製造業に特化したデジタルトランスフォーメーション(DX)の実現を目指す請負開発型のコンサルティングサービスです。AI、iPaaS、および先端の技術を駆使して、製造プロセスの効率化、業務効率化、チームワーク強化、コスト削減、品質向上を実現します。このサービスは、製造業の課題を深く理解し、それに対する最適なデジタルソリューションを提供することで、企業が持続的な成長とイノベーションを達成できるようサポートします。
オンライン講座
製造業、主に購買・調達部門にお勤めの方々に向けた情報を配信しております。
新任の方やベテランの方、管理職を対象とした幅広いコンテンツをご用意しております。
お問い合わせ
コストダウンが利益に直結する術だと理解していても、なかなか前に進めることができない状況。そんな時は、newjiのコストダウン自動化機能で大きく利益貢献しよう!
(Β版非公開)