- お役立ち記事
- Fundamentals and practical points of model predictive control and its know-how
Fundamentals and practical points of model predictive control and its know-how
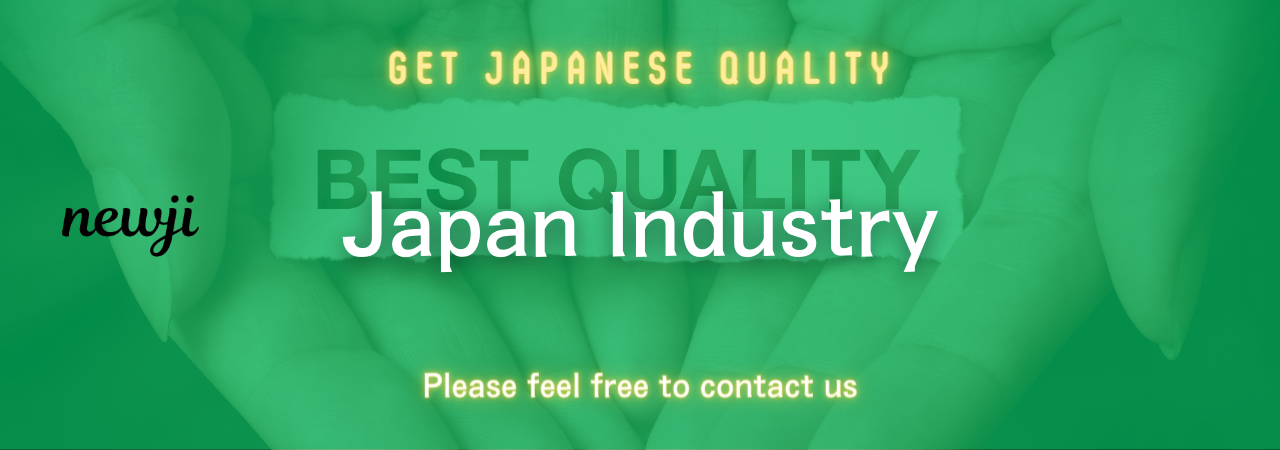
目次
Understanding Model Predictive Control (MPC): An Introduction
Model Predictive Control, often abbreviated as MPC, is an advanced control strategy that has gained significant popularity in various industries due to its ability to handle multivariable control problems and constraints.
The basic idea behind MPC is to predict the future behavior of a system using a mathematical model and optimize the control actions to achieve a specific objective, such as minimizing energy consumption or reducing fluctuations in process variables.
To understand MPC, it’s essential to grasp its fundamental components and approach.
At its core, MPC involves three primary steps: prediction, optimization, and control implementation.
The system model is used to predict future outputs over a specified horizon, typically several time steps into the future.
An optimization algorithm then determines the optimal control actions that achieve the desired objectives while satisfying system constraints.
Finally, only the first control action is applied to the system, and the process is repeated at the next time step.
The Importance of Model Predictive Control
The rise in popularity of MPC can be attributed to its flexibility and efficacy in complex, real-world scenarios.
One of its most significant advantages is the ability to handle multiple inputs and outputs simultaneously, making it particularly useful for processes with interacting variables.
This capability is crucial in industries like petrochemicals, automotive, and energy, where multivariable control is a norm rather than the exception.
Furthermore, MPC can effectively manage constraints that are often inherent in industrial processes.
For instance, safety or operational limits on variables such as pressure, temperature, or flow rate can be explicitly incorporated into the control strategy.
This ensures that the process operates safely and efficiently within its allowable bounds.
Additionally, MPC’s predictive nature allows it to anticipate future disturbances or changes in operating conditions, leading to more proactive control strategies.
This results in improved performance compared to traditional control methods that react to changes after they occur.
Key Concepts in Model Predictive Control
The Role of the System Model
A critical component of MPC is the system model, which serves as the foundation for predicting future behavior.
The model can vary in complexity, ranging from simple linear models to more intricate nonlinear ones, depending on the process being controlled.
Developing an accurate model is vital, as any discrepancies can lead to suboptimal control and performance issues.
Prediction Horizon and Control Horizon
Two important concepts in MPC are the prediction horizon and the control horizon.
The prediction horizon refers to the number of future time steps over which predictions are made, while the control horizon is the number of time steps for which control actions are optimized.
Selecting appropriate horizons is crucial, as they influence both the performance and computational load of the MPC algorithm.
Optimization Techniques
At the core of MPC is an optimization problem solved at each time step.
Various optimization techniques can be employed, ranging from simple linear programming for linear systems to more complex nonlinear programming for systems with nonlinear dynamics.
The choice of optimization technique impacts both the execution speed and the accuracy of the MPC solution.
Implementing Model Predictive Control: Practical Tips
Successfully implementing MPC requires a sound understanding of both theoretical concepts and practical considerations.
Model Accuracy
As highlighted earlier, the accuracy of the system model is crucial.
Invest time in model identification and validation to ensure it closely represents the actual process.
This may involve collecting extensive process data and employing techniques such as system identification or first-principle modeling.
Handling Constraints
Constraints are a fundamental aspect of MPC, but they can also be a source of complexity.
Clearly define and prioritize constraints based on process requirements.
Incorporate both hard constraints (absolute limits) and soft constraints (preferential limits) to balance operational objectives and flexibility.
Computational Considerations
MPC algorithms can be computationally demanding, particularly for large-scale or fast systems.
To ensure real-time implementation, focus on efficient coding and optimization methods.
Consider utilizing specialized MPC software or hardware accelerators for high-speed applications.
Tuning and Testing
Like any control strategy, MPC requires careful tuning to achieve the desired performance.
Start with a comprehensive testing phase using simulations to evaluate and adjust parameters such as control horizons, weights in the cost function, and model accuracy.
Incorporate robust testing protocols to account for variability in process conditions and disturbances.
Advanced Topics and Future Trends in MPC
While the principles of MPC remain consistent, the field continues to evolve with advancements in technology and research.
Integrating machine learning approaches to improve model accuracy and adapt to process changes is an emerging trend.
Combining MPC with data-driven methods allows for more adaptive and resilient control strategies.
Robust MPC is another area of active research, focusing on handling system uncertainties and external disturbances.
This involves developing algorithms that can provide reliable control actions in the face of unknowns, making MPC more robust and applicable to a broader range of industries.
Finally, the extension of MPC into decentralized and distributed control frameworks is gaining traction.
As industrial systems become more interconnected and large-scale, the ability to coordinate control actions across multiple subsystems becomes increasingly valuable.
In summary, Model Predictive Control offers a powerful framework for addressing complex control challenges in numerous industrial applications.
Understanding its fundamentals, practical implementation aspects, and keeping abreast of ongoing advancements will equip practitioners with the tools needed to harness the full potential of MPC.
資料ダウンロード
QCD調達購買管理クラウド「newji」は、調達購買部門で必要なQCD管理全てを備えた、現場特化型兼クラウド型の今世紀最高の購買管理システムとなります。
ユーザー登録
調達購買業務の効率化だけでなく、システムを導入することで、コスト削減や製品・資材のステータス可視化のほか、属人化していた購買情報の共有化による内部不正防止や統制にも役立ちます。
NEWJI DX
製造業に特化したデジタルトランスフォーメーション(DX)の実現を目指す請負開発型のコンサルティングサービスです。AI、iPaaS、および先端の技術を駆使して、製造プロセスの効率化、業務効率化、チームワーク強化、コスト削減、品質向上を実現します。このサービスは、製造業の課題を深く理解し、それに対する最適なデジタルソリューションを提供することで、企業が持続的な成長とイノベーションを達成できるようサポートします。
オンライン講座
製造業、主に購買・調達部門にお勤めの方々に向けた情報を配信しております。
新任の方やベテランの方、管理職を対象とした幅広いコンテンツをご用意しております。
お問い合わせ
コストダウンが利益に直結する術だと理解していても、なかなか前に進めることができない状況。そんな時は、newjiのコストダウン自動化機能で大きく利益貢献しよう!
(Β版非公開)