- お役立ち記事
- Fundamentals of accelerated reliability test data/Weibull analysis and practical points for estimating service life
Fundamentals of accelerated reliability test data/Weibull analysis and practical points for estimating service life
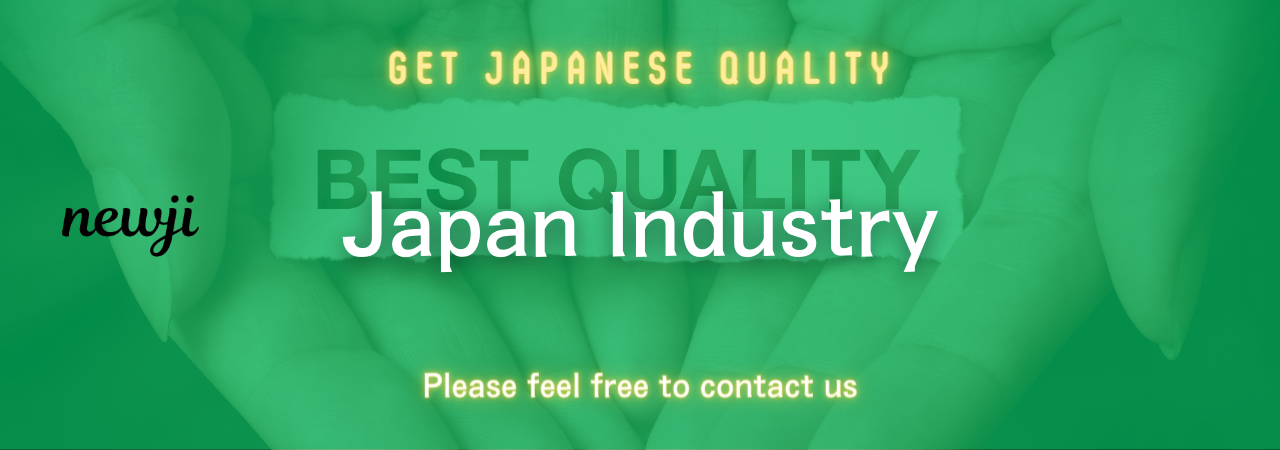
目次
Understanding Accelerated Reliability Test Data
Reliability testing plays a critical role in ensuring that products meet the required standards of performance and durability.
Accelerated reliability tests are designed to predict the lifespan of a product by exposing it to conditions that are more extreme than normal usage.
This helps manufacturers identify potential weaknesses and improve product designs before they reach the market.
In essence, accelerated reliability test data provides valuable insights into how products will perform over time.
Through analyzing this data, businesses can make informed decisions about product improvements and quality assurance strategies.
The process typically involves simulating years of regular use in a much shorter timeframe by increasing stress factors such as temperature, humidity, or vibration.
Weibull Analysis: A Key Tool
Weibull analysis is a statistical technique used in reliability engineering to analyze life data and model the time-to-failure of products.
Named after Swedish mathematician Waloddi Weibull, this method helps in understanding the distribution of failures and predicting future performance.
By using a Weibull distribution model, engineers can estimate the probability of a product failing at any given time and draw important conclusions about its reliability.
Weibull analysis is particularly valuable because of its flexibility.
It can model various types of failure rates such as increasing, constant, or decreasing, depending on the data.
This adaptability makes it applicable across a broad range of industries, from electronics to automotive and beyond.
Parameters of the Weibull Distribution
The Weibull distribution is characterized by two main parameters: the shape parameter (beta) and the scale parameter (eta).
These parameters determine the behavior of the failure rate.
1. **Shape Parameter (Beta)**: This parameter indicates the failure rate behavior.
– If beta is less than 1, the failure rate decreases over time, which is typical for early-life failures.
– A beta equal to 1 indicates a constant failure rate, often seen in random failures.
– When beta is greater than 1, the failure rate increases over time, common for wear-out failures.
2. **Scale Parameter (Eta)**: This parameter represents the characteristic life, indicating the time by which 63.2% of the population will have failed.
It is crucial for comparing the relative lifespan of products.
Steps in Conducting Weibull Analysis
Conducting Weibull analysis involves several steps:
1. **Data Collection**: Gather life data from accelerated tests or field tests, noting running hours, cycles, or other relevant metrics until failure.
2. **Data Plotting**: Plot the life data on a Weibull probability plot to assess the linearity and fit of the model.
This involves transforming data to a continuous scale, allowing for easier identification of trends.
3. **Parameter Estimation**: Use statistical software to fit the Weibull distribution to the data and estimate the beta and eta parameters.
Maximum likelihood estimation is a common technique used for this purpose.
4. **Goodness-of-Fit Testing**: Evaluate the fitted model’s suitability through goodness-of-fit tests to ensure the analysis is reliable and accurate.
5. **Result Interpretation**: Analyze the shape and scale parameters to understand the failure mechanisms and predict future reliability.
Estimating Service Life: Practical Points
One of the primary objectives of accelerated reliability testing and Weibull analysis is to estimate the service life of a product.
Understanding how to do this effectively involves several practical considerations.
Driving Conditions and Stress Levels
In accelerated testing, it’s crucial to simulate actual usage conditions as closely as possible, while also increasing stress levels to observe failures more quickly.
This balance ensures that the data gathered is relevant and that the predictions align with real-world conditions.
Selecting appropriate stress factors is key—these might include temperature cycling, high humidity exposure, mechanical loading, or electrical voltage increases.
Data Censoring and Treatment
Not all data points will equally inform the reliability analysis.
Some data may be right-censored (tests that end without failure) or left-censored (failures that occur outside the observed window).
Properly accounting for these censored data points enhances the accuracy of the analysis.
Techniques like Kaplan-Meier or the use of specialized software can help in this process.
Lifestyle Variability
Different users may subject products to varying usage patterns, which can affect service life estimates.
Understanding and accounting for this variability ensures that reliability predictions are more robust and applicable to diverse scenarios.
For example, a smartphone might see heavy use by one individual, while another user may use it sparingly.
Incorporating this understanding can refine estimates and improve product designs.
Model Validation
Once predictions are made, it is crucial to validate the model’s accuracy against actual field performance.
This step helps to refine predictions and enhance the reliability model for future products.
Field data collection and analysis should be an ongoing process, allowing adjustments as needed to improve accuracy and relevance.
Conclusion
Accelerated reliability test data and Weibull analysis are invaluable tools for predicting product lifespan and ensuring quality.
By understanding the underlying principles and practical considerations, businesses can improve their products and enhance their competitive edge.
Accurate service life estimation not only supports better product development but also bolsters consumer trust through dependable performance.
In an ever-evolving market, leveraging these techniques can be a significant step towards achieving long-term success.
資料ダウンロード
QCD調達購買管理クラウド「newji」は、調達購買部門で必要なQCD管理全てを備えた、現場特化型兼クラウド型の今世紀最高の購買管理システムとなります。
ユーザー登録
調達購買業務の効率化だけでなく、システムを導入することで、コスト削減や製品・資材のステータス可視化のほか、属人化していた購買情報の共有化による内部不正防止や統制にも役立ちます。
NEWJI DX
製造業に特化したデジタルトランスフォーメーション(DX)の実現を目指す請負開発型のコンサルティングサービスです。AI、iPaaS、および先端の技術を駆使して、製造プロセスの効率化、業務効率化、チームワーク強化、コスト削減、品質向上を実現します。このサービスは、製造業の課題を深く理解し、それに対する最適なデジタルソリューションを提供することで、企業が持続的な成長とイノベーションを達成できるようサポートします。
オンライン講座
製造業、主に購買・調達部門にお勤めの方々に向けた情報を配信しております。
新任の方やベテランの方、管理職を対象とした幅広いコンテンツをご用意しております。
お問い合わせ
コストダウンが利益に直結する術だと理解していても、なかなか前に進めることができない状況。そんな時は、newjiのコストダウン自動化機能で大きく利益貢献しよう!
(Β版非公開)