- お役立ち記事
- Fundamentals of autonomous mobility technology and applications to autonomous driving/robot surrounding environment recognition and behavior prediction technology
Fundamentals of autonomous mobility technology and applications to autonomous driving/robot surrounding environment recognition and behavior prediction technology
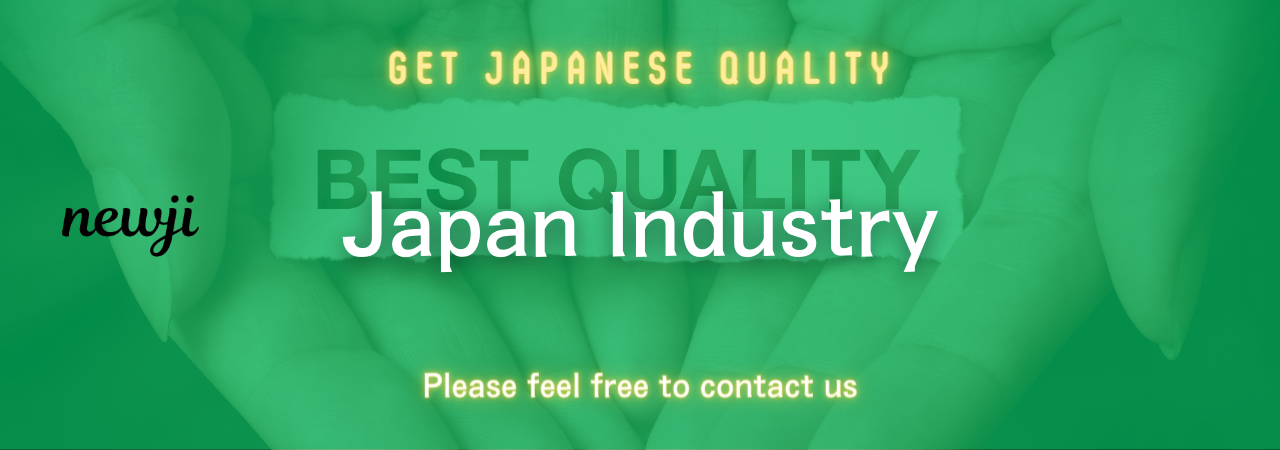
目次
Understanding Autonomous Mobility Technology
Autonomous mobility technology is paving the way for significant advancements in various sectors, particularly within autonomous driving systems.
This technology encompasses a wide range of innovations that enable vehicles to operate without human intervention.
From intricate software algorithms to sophisticated hardware components, autonomous mobility technology is designed to enhance safety, efficiency, and convenience in transportation.
The main components of autonomous mobility technology include sensors, actuators, machine learning algorithms, and connectivity systems.
Each of these elements plays a crucial role in achieving the goal of fully autonomous vehicles.
The Role of Sensors in Autonomous Vehicles
Sensors are fundamental to the functionality of autonomous vehicles.
They serve as the primary means for these vehicles to perceive and interpret their surrounding environment.
There are several types of sensors used in autonomous systems, including cameras, radar, lidar, and ultrasonic sensors.
Cameras are used to capture visual information from the environment, providing data that can be analyzed for object recognition and classification.
Radar sensors are particularly useful for detecting objects at long distances and are not affected by weather conditions like fog or rain.
Lidar sensors, which use laser beams to map the environment, provide high-resolution 3D images, making them essential for detailed environmental modeling.
Ultrasonic sensors are employed for short-range detection, particularly useful in parking scenarios.
Actuators and Their Importance
Actuators are the components in autonomous vehicles that convert electronic signals from the control system into physical actions.
They are responsible for controlling mechanisms such as steering, braking, and acceleration.
The smooth operation of actuators is crucial for the accurate execution of driving commands and ensuring passenger safety.
To achieve this, actuators are integrated with advanced control systems that adjust their responses based on real-time data from the vehicle’s sensors.
This system of sensors and actuators working in harmony forms the backbone of autonomous driving technology.
Machine Learning and Autonomous Decision-Making
Machine learning is at the core of autonomous decision-making processes.
It involves training systems to interpret data from sensors and make decisions based on that information.
Through extensive exposure to data, machine learning models can learn to identify patterns and predict potential scenarios on the road.
These models employ various techniques such as deep learning, reinforcement learning, and neural networks to enhance their ability to learn and make predictions.
For instance, neural networks can be designed to recognize stop signs, traffic lights, and pedestrians, aiding in the development of a comprehensive driving strategy.
Reinforcement learning algorithms help vehicles improve their decision-making skills by simulating trial-and-error processes.
By learning from mistakes and successes in simulations, these algorithms can optimize driving strategies for real-world applications.
Applications in Autonomous Driving
The application of autonomous mobility technology in autonomous driving has led to the development of vehicles that can navigate complex environments with minimal human oversight.
These vehicles can manage tasks such as lane changing, traffic sign recognition, and obstacle avoidance, all thanks to the integration of advanced technologies.
One significant application is in ride-sharing services, where autonomous vehicles can provide transportation without human drivers.
This not only increases efficiency but also reduces operational costs for service providers.
Additionally, autonomous technology is being leveraged in public transport systems to enhance accessibility and safety.
Surrounding Environment Recognition
Recognizing the surrounding environment is a critical task for autonomous vehicles.
This involves accurately identifying and understanding various elements such as road signs, obstacles, and traffic conditions.
Sophisticated algorithms process the data collected by sensors, transforming it into actionable insights for vehicle navigation.
Autonomous systems employ techniques like simultaneous localization and mapping (SLAM) to create and update maps of their environments.
These maps help vehicles to determine their positions and make informed decisions about their navigation paths.
Behavior Prediction Technology
Behavior prediction technology enables autonomous vehicles to anticipate the actions of other road users, such as vehicles, cyclists, and pedestrians.
By analyzing the behavior patterns of these entities, autonomous vehicles can predict their movements and adjust their actions accordingly to avoid collisions and ensure smooth traffic flow.
For example, if a vehicle detects a pedestrian approaching a crosswalk, it can predict that the pedestrian may cross the street and slow down or stop to allow safe passage.
This predictive capability is essential for the seamless integration of autonomous vehicles into existing traffic systems.
Future Prospects and Challenges
The future of autonomous mobility technology is promising, with continuous advancements on the horizon.
However, significant challenges remain that need to be addressed to realize fully autonomous driving.
One of the main challenges is ensuring the safety and reliability of autonomous systems under diverse and unpredictable conditions.
Rigorous testing and validation procedures are required to guarantee the performance of these systems in various scenarios.
Moreover, public acceptance and regulatory frameworks need to evolve alongside technological developments.
Establishing clear regulations and building public trust are crucial steps in facilitating the widespread adoption of autonomous vehicles.
In conclusion, autonomous mobility technology is revolutionizing the way we perceive and interact with transportation systems.
From enhancing road safety to offering more sustainable and efficient modes of transport, the impact of autonomous technology is profound.
With continued research and development, the vision of fully autonomous vehicles is steadily becoming a reality.
資料ダウンロード
QCD調達購買管理クラウド「newji」は、調達購買部門で必要なQCD管理全てを備えた、現場特化型兼クラウド型の今世紀最高の購買管理システムとなります。
ユーザー登録
調達購買業務の効率化だけでなく、システムを導入することで、コスト削減や製品・資材のステータス可視化のほか、属人化していた購買情報の共有化による内部不正防止や統制にも役立ちます。
NEWJI DX
製造業に特化したデジタルトランスフォーメーション(DX)の実現を目指す請負開発型のコンサルティングサービスです。AI、iPaaS、および先端の技術を駆使して、製造プロセスの効率化、業務効率化、チームワーク強化、コスト削減、品質向上を実現します。このサービスは、製造業の課題を深く理解し、それに対する最適なデジタルソリューションを提供することで、企業が持続的な成長とイノベーションを達成できるようサポートします。
オンライン講座
製造業、主に購買・調達部門にお勤めの方々に向けた情報を配信しております。
新任の方やベテランの方、管理職を対象とした幅広いコンテンツをご用意しております。
お問い合わせ
コストダウンが利益に直結する術だと理解していても、なかなか前に進めることができない状況。そんな時は、newjiのコストダウン自動化機能で大きく利益貢献しよう!
(Β版非公開)