- お役立ち記事
- Fundamentals of Bayesian inference and applications to prediction and data analysis
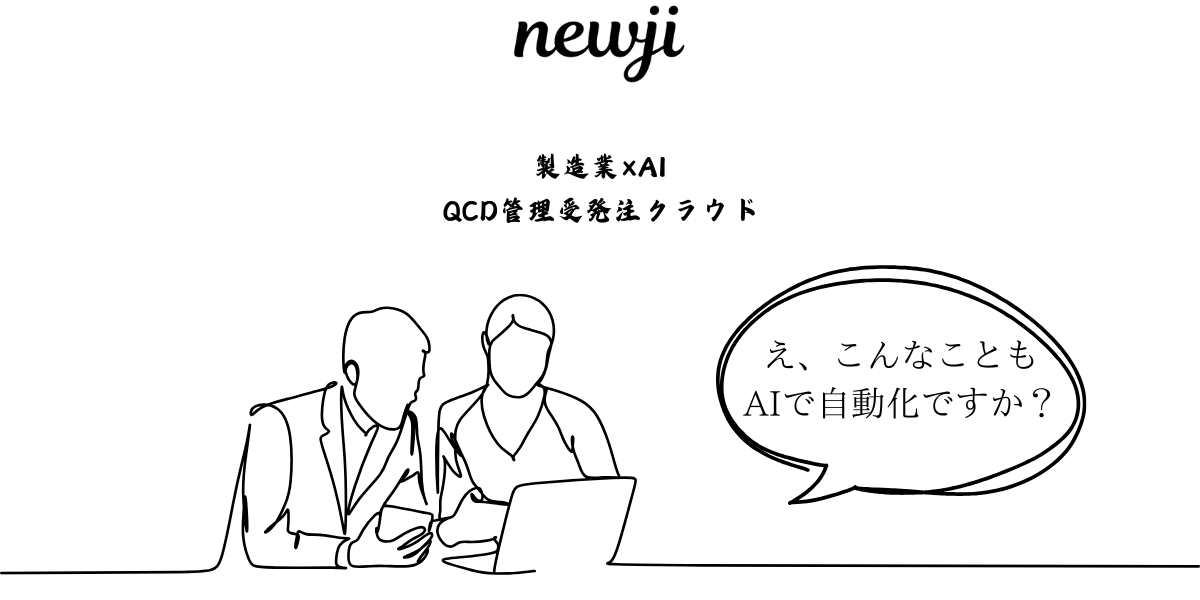
Fundamentals of Bayesian inference and applications to prediction and data analysis
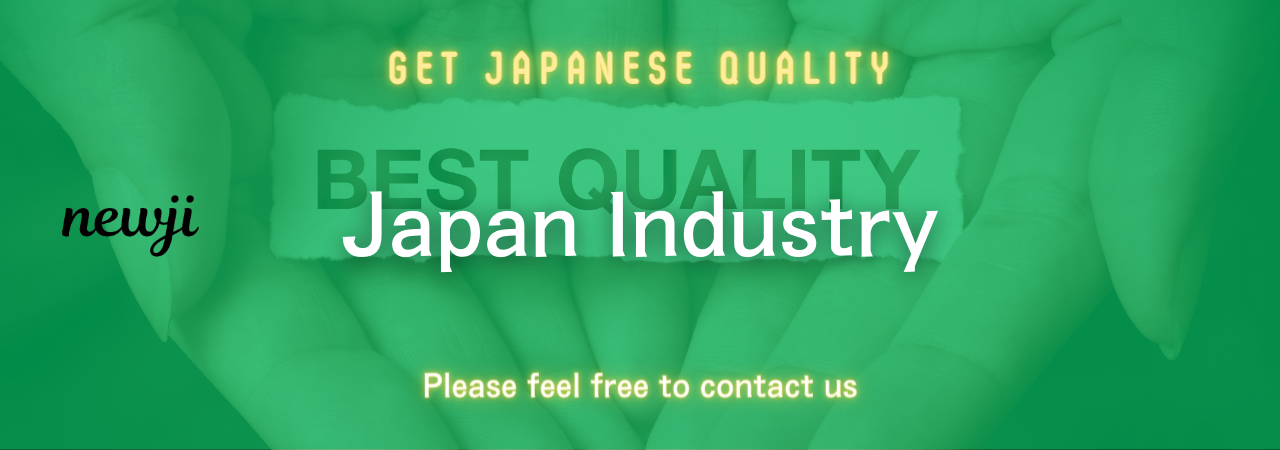
目次
Understanding Bayesian Inference
Bayesian inference is a statistical method that allows us to update our beliefs about an event’s probability based on new evidence.
This powerful approach is rooted in Bayes’ Theorem, a fundamental concept in probability theory.
Bayes’ Theorem describes the probability of an event, based on prior knowledge of conditions related to the event.
The core idea behind Bayesian inference is to start with an initial belief, known as the prior probability, and then adjust this belief when new data is available.
This updated belief is called the posterior probability.
This method stands in contrast to classical, or frequentist, inference, which relies solely on the data at hand without incorporating prior information.
Bayes’ Theorem Explained
Bayes’ Theorem can be expressed mathematically using the following equation:
P(A|B) = [P(B|A) * P(A)] / P(B)
Here, P(A|B) is the posterior probability— the probability of event A occurring given that B is true.
P(B|A) is the likelihood— the probability of B occurring if A is true.
P(A) is the prior probability, reflecting our initial belief about the probability of A.
P(B) is the marginal likelihood, a normalizing constant ensuring the probabilities sum to one.
Through this theorem, Bayesian inference provides a framework to update our understanding and make predictions about future events.
The Role of Prior Probability
In Bayesian inference, prior probability plays a critical role in shaping the results.
It reflects what is known or believed about a parameter before new data is considered.
Choosing an appropriate prior can be subjective and depends on the context or past experiences.
In some cases, an uninformative or non-informative prior is used when there’s no strong prior belief, aiming to minimize bias.
In other situations, informative priors incorporate specific historical data or expert knowledge.
The choice of prior can significantly affect the results, especially with a small data sample.
As more data becomes available, the influence of the prior diminishes, and the likelihood becomes the principal component in determining the posterior probability.
Updating Beliefs with New Data
The beauty of Bayesian inference lies in its ability to update probabilities dynamically as new data becomes available.
This iterative process involves recalculating the posterior probability repeatedly as new evidence is introduced.
This continuous updating reflects the real-world process of learning and making decisions based on accumulating information.
For instance, consider a doctor diagnosing a patient.
Initially, the doctor has some prior belief about the presence of a disease based on the prevalence rate.
As test results (new data) are gathered, the doctor’s belief (posterior probability) is updated, refining the diagnosis.
Bayesian Inference in Machine Learning
In the realm of machine learning, Bayesian inference is used in various models, such as Gaussian processes, Bayesian networks, and Bayesian linear regression.
These models benefit from the ability to incorporate prior knowledge and adjust predictions based on incoming data.
Bayesian approaches are particularly valuable in situations with limited data, where incorporating expert knowledge can improve model performance.
Applications in Prediction and Data Analysis
Bayesian inference is widely used in various fields, owing to its flexibility and robustness.
Its applications span prediction, data analysis, and decision-making processes.
Predictive Modeling
Bayesian methods are particularly useful in predictive modeling, where there’s uncertainty about the underlying processes generating the data.
By incorporating prior knowledge, Bayesian models can provide more accurate predictions and account for various sources of uncertainty.
For example, in weather forecasting, Bayesian inference can integrate historical data and current observations with physical models of atmospheric behavior, leading to more reliable forecasts than traditional methods.
Data Analysis
In data analysis, Bayesian inference is valuable for hypothesis testing, parameter estimation, and model selection.
By evaluating the likelihood of different hypotheses or models given the data, analysts can make more informed decisions regarding the best explanation of the observed phenomena.
In fields like bioinformatics, Bayesian methods are employed to analyze complex biological data, providing insights into genetic interactions and evolutionary processes.
Decision Making
Bayesian inference supports decision-making processes by quantifying uncertainty and incorporating various data sources.
This is crucial in sectors like finance, where investment decisions must consider both historical performance and current market conditions.
In medical decision-making, Bayesian approaches can help determine the optimal treatment strategy by integrating evidence from clinical trials and patient data.
Challenges and Considerations
While Bayesian inference offers numerous advantages, it also presents challenges.
One primary concern is the computational complexity involved in calculating posterior probabilities, especially with large datasets or complex models.
Advancements in computational techniques, such as Markov Chain Monte Carlo (MCMC) methods, have helped mitigate these challenges, making Bayesian methods more accessible.
Another consideration is the selection of priors, which can introduce subjectivity and bias if not carefully chosen.
Balancing the influence of prior beliefs and new evidence is essential for ensuring objective and reliable results.
Conclusion
Bayesian inference is a powerful tool for understanding uncertainty and making predictions based on available data and prior knowledge.
It offers a flexible approach to modeling complex systems and processes, enabling more informed decision-making across various domains.
Despite computational challenges and the need for careful prior selection, the benefits of incorporating Bayesian methods into prediction and data analysis are significant.
As technology and computational methods continue to advance, the scope and impact of Bayesian inference will undoubtedly expand, offering deeper insights into the complexities of the world around us.
資料ダウンロード
QCD調達購買管理クラウド「newji」は、調達購買部門で必要なQCD管理全てを備えた、現場特化型兼クラウド型の今世紀最高の購買管理システムとなります。
ユーザー登録
調達購買業務の効率化だけでなく、システムを導入することで、コスト削減や製品・資材のステータス可視化のほか、属人化していた購買情報の共有化による内部不正防止や統制にも役立ちます。
NEWJI DX
製造業に特化したデジタルトランスフォーメーション(DX)の実現を目指す請負開発型のコンサルティングサービスです。AI、iPaaS、および先端の技術を駆使して、製造プロセスの効率化、業務効率化、チームワーク強化、コスト削減、品質向上を実現します。このサービスは、製造業の課題を深く理解し、それに対する最適なデジタルソリューションを提供することで、企業が持続的な成長とイノベーションを達成できるようサポートします。
オンライン講座
製造業、主に購買・調達部門にお勤めの方々に向けた情報を配信しております。
新任の方やベテランの方、管理職を対象とした幅広いコンテンツをご用意しております。
お問い合わせ
コストダウンが利益に直結する術だと理解していても、なかなか前に進めることができない状況。そんな時は、newjiのコストダウン自動化機能で大きく利益貢献しよう!
(Β版非公開)