- お役立ち記事
- Fundamentals of Bayesian modeling and application examples to statistical inference and pattern recognition
月間76,176名の
製造業ご担当者様が閲覧しています*
*2025年3月31日現在のGoogle Analyticsのデータより
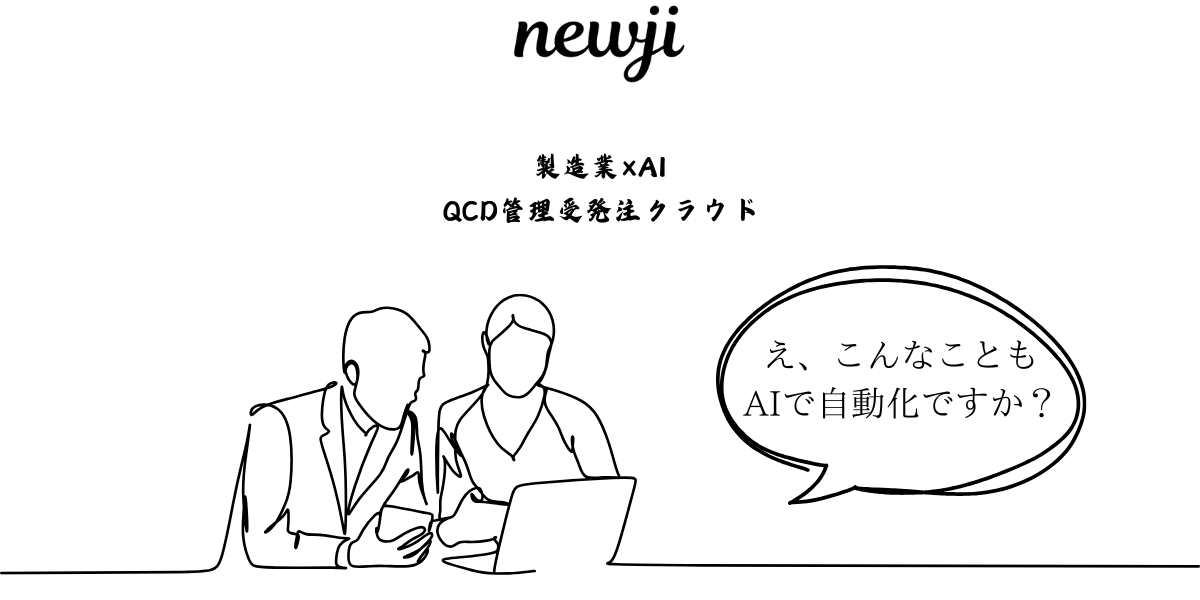
Fundamentals of Bayesian modeling and application examples to statistical inference and pattern recognition
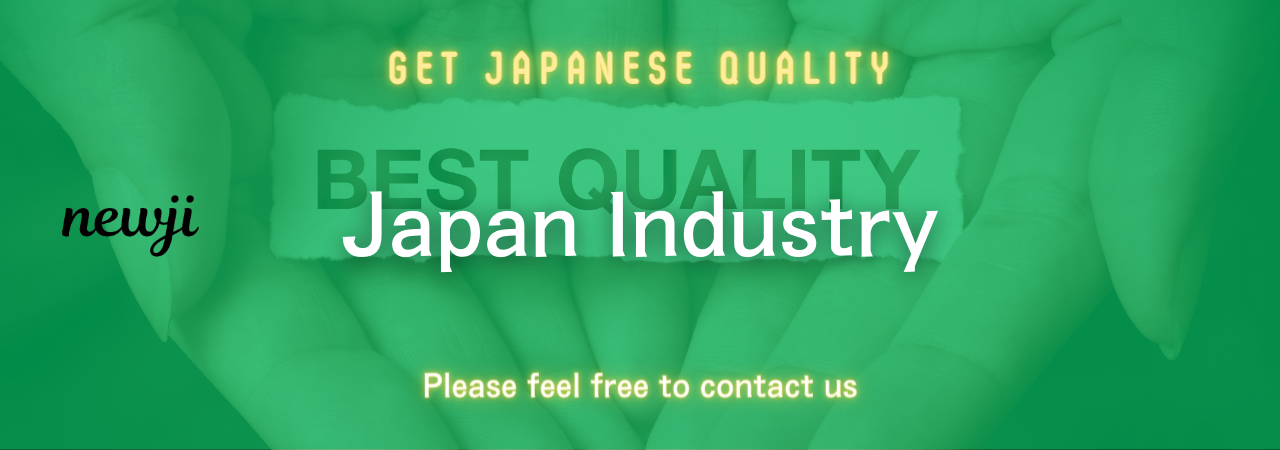
目次
Understanding Bayesian Modeling
Bayesian modeling is a statistical approach that relies on Bayes’ Theorem.
It’s a method used to update the probability for a hypothesis as more evidence or information becomes available.
In essence, Bayesian modeling provides a framework for decision-making under uncertainty, which is ubiquitous in both theoretical and applied statistics.
One of the key aspects of Bayesian modeling is its ability to incorporate prior knowledge or beliefs into the analysis.
This is done through the use of a “prior distribution” that reflects our understanding of the parameters before observing the data.
As new data is obtained, the prior distribution is updated to form a “posterior distribution,” which gives us a revised understanding of those parameters.
Bayes’ Theorem in Action
Bayes’ Theorem is the backbone of Bayesian modeling.
The theorem states the following relationship:
P(H|E) = (P(E|H) * P(H)) / P(E)
Here, P(H|E) is the probability of the hypothesis H given the evidence E.
P(E|H) is the probability of the evidence E under the condition that H is true, also known as the likelihood.
P(H) is the prior probability of the hypothesis before seeing the evidence, called the prior.
P(E) is the probability of the evidence.
Through this formula, we see how pre-existing beliefs are updated by new evidence.
This continuous update process is invaluable in fields that require evolving data analysis.
Applications in Statistical Inference
Bayesian modeling is extensively used in statistical inference.
One major application is parameter estimation.
In Bayesian parameter estimation, we derive the posterior distribution of a parameter given the observed data and prior distribution.
This approach is beneficial in that it provides a full distribution of parameter estimates, allowing for more comprehensive uncertainty quantification.
For example, in medical research, Bayesian methods can be more robust in estimating treatment effects over small samples compared to frequentist methods.
The integration of prior knowledge about the treatment can lead to better decision-making despite limited data.
Hypothesis Testing
In traditional hypothesis testing, we often reject or fail to reject a null hypothesis based on a p-value.
Bayesians use posterior probabilities to make these decisions, offering a different perspective.
The Bayesian approach provides the probability of a hypothesis directly, given the data, rather than simply deciding based on whether a statistic falls within a critical region.
This direct computation of probabilities often aligns more closely with intuitive decision-making.
Pattern Recognition with Bayesian Models
Pattern recognition involves identifying regularities within data.
Bayesian models are powerful in this realm due to their probabilistic framework.
They allow the model to express uncertainty about patterns, which can be strategically adjusted with the acquisition of more data.
Machine Learning and Classification
Bayesian models are foundational in machine learning, particularly in classification tasks.
Naive Bayes classifiers, for instance, are straightforward yet effective probabilistic classifiers.
Despite the simplifying assumption that features are independent given the class label, Naive Bayes performs surprisingly well in practice.
It is used in email spam detection, document classification, and sentiment analysis.
Beyond Naive Bayes, Bayesian neural networks are gaining traction.
In these networks, weights are treated as distributions rather than fixed values, offering advantages in capturing uncertainty over predictions.
This is especially beneficial in critical applications like autonomous driving or healthcare, where understanding the confidence of predictions is paramount.
Time Series and Dynamic Systems
Time series prediction is another area where Bayesian methods shine.
They are adept at handling dynamically changing environments.
Markov Chain Monte Carlo (MCMC) methods and Kalman filters are popular tools used in the Bayesian framework for these applications.
They allow for sequential updates to predictions as new time points are added, maintaining robustness over time.
Bayesian models are also employed in dynamic systems like robotics, where the understanding of the environment needs to be continuously updated with sensor data.
These models help in navigating uncertainties in real-world applications, ensuring smoother and more reliable operations.
Advantages and Challenges
The advantages of Bayesian modeling are numerous.
Its ability to incorporate prior knowledge can result in more informed predictions than pure data-driven models in situations with limited data.
Bayesian models also offer a principled approach to handling uncertainty, which can guide decision-making processes more effectively.
However, there are challenges as well.
The choice of prior can be subjective, and poorly chosen priors can lead to misleading results.
Moreover, Bayesian computation can be intensive, particularly in complex models, though advances in computational power and algorithms like MCMC have made these models more accessible.
Conclusion
Bayesian modeling serves as a versatile tool in the statistician’s toolkit.
Its frameworks offer robust methodologies for updating beliefs and making predictions in spite of uncertainty.
With applications spanning from statistical inference to sophisticated pattern recognition systems, Bayesian methods are integral to modern data analysis.
As computational techniques continue to evolve, the scope and efficiency of Bayesian modeling will likely expand, further embedding it into diverse scientific and practical domains.
資料ダウンロード
QCD管理受発注クラウド「newji」は、受発注部門で必要なQCD管理全てを備えた、現場特化型兼クラウド型の今世紀最高の受発注管理システムとなります。
ユーザー登録
受発注業務の効率化だけでなく、システムを導入することで、コスト削減や製品・資材のステータス可視化のほか、属人化していた受発注情報の共有化による内部不正防止や統制にも役立ちます。
NEWJI DX
製造業に特化したデジタルトランスフォーメーション(DX)の実現を目指す請負開発型のコンサルティングサービスです。AI、iPaaS、および先端の技術を駆使して、製造プロセスの効率化、業務効率化、チームワーク強化、コスト削減、品質向上を実現します。このサービスは、製造業の課題を深く理解し、それに対する最適なデジタルソリューションを提供することで、企業が持続的な成長とイノベーションを達成できるようサポートします。
製造業ニュース解説
製造業、主に購買・調達部門にお勤めの方々に向けた情報を配信しております。
新任の方やベテランの方、管理職を対象とした幅広いコンテンツをご用意しております。
お問い合わせ
コストダウンが利益に直結する術だと理解していても、なかなか前に進めることができない状況。そんな時は、newjiのコストダウン自動化機能で大きく利益貢献しよう!
(β版非公開)