- お役立ち記事
- Fundamentals of Bayesian signal processing and applications to noise removal
Fundamentals of Bayesian signal processing and applications to noise removal
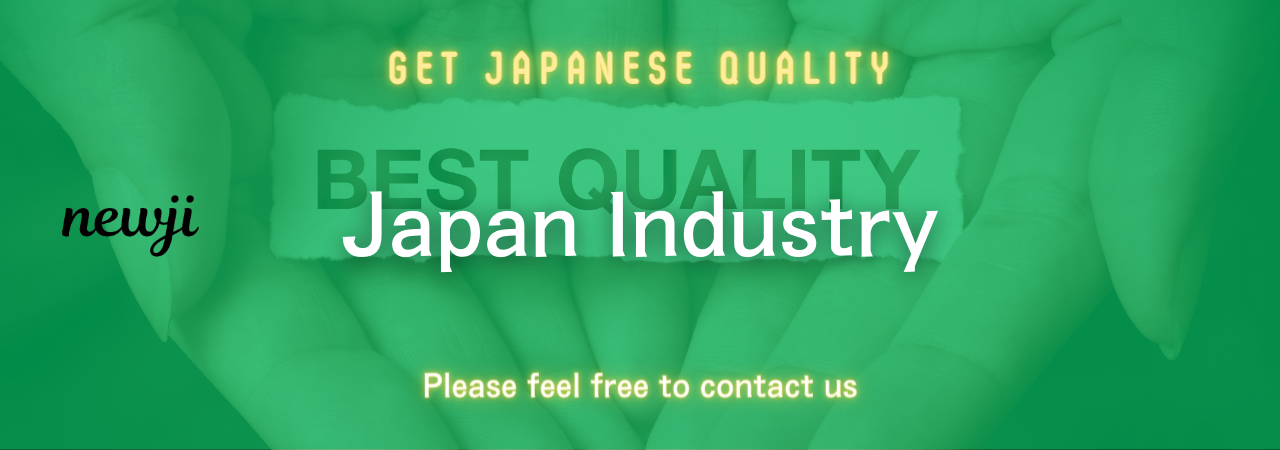
目次
Understanding Bayesian Signal Processing
Bayesian signal processing is a powerful method used in various fields to analyze and interpret complex data.
At its core, Bayesian signal processing is about applying the principles of Bayesian probability to signal processing tasks.
By doing so, it provides a clear framework for dealing with uncertainties and making informed decisions based on observed data.
In Bayesian signal processing, we work with prior probability distributions to model our initial beliefs about the data.
As new data is observed, we update these beliefs using Bayes’ theorem to obtain a posterior probability distribution.
This approach allows us to incorporate prior knowledge and observed evidence into our analysis, improving the accuracy and reliability of our findings.
Core Concepts and Tools
Several fundamental concepts and tools are central to understanding Bayesian signal processing.
One key element is the use of probability distributions to represent uncertainty.
These distributions, such as Gaussian, Poisson, or uniform, help in modeling the randomness inherent in signal data.
Bayes’ theorem, which underpins Bayesian signal processing, is a mathematical formula that relates prior probability to posterior probability.
It updates our belief in a hypothesis as more evidence becomes available.
This dynamic updating process is what allows Bayesian methods to be so effective in signal processing tasks.
Markov Chain Monte Carlo (MCMC) methods are another crucial tool in Bayesian signal processing.
MCMC algorithms are used to sample from complex probability distributions that are otherwise difficult to compute.
These methods enable the estimation of posterior distributions, making it possible to infer parameters and predict future outcomes.
Noise in Signal Processing
In the realm of signal processing, noise is an unwanted alteration of the original signal.
Noise can degrade the quality and reliability of the information extracted from data.
Common sources of noise include measurement errors, environmental factors, and electronic interference.
Understanding the type and source of noise is crucial in designing effective noise removal techniques.
Noise can be categorized as either random or structured.
Random noise is unpredictable and varies in an irregular manner, while structured noise has a recognizable pattern that can potentially be identified and removed.
Applications of Bayesian Methods in Noise Removal
Bayesian signal processing offers sophisticated techniques for mitigating the effects of noise.
These techniques are widely used in a variety of applications, including communications, radar, and medical imaging.
One common application is in the field of communications, where Bayesian methods are used to clean up noisy signals for more accurate data transmission.
In radar systems, Bayesian algorithms help in detecting and isolating true targets from cluttered environments.
In medical imaging, noise removal is essential for obtaining clear images that aid in accurate diagnosis and treatment planning.
Bayesian Filtering Techniques
Bayesian filtering is a key technique in removing noise from signals.
It involves estimating the true state of a system over time, given partially observed and noisy data.
This is achieved by using recursive filtering methods such as the Kalman filter and the Particle filter.
The Kalman filter is ideal for linear systems and assumes that both the process and observation noises are Gaussian.
It operates in two steps: prediction and update.
First, it predicts the next state of the system and then updates this prediction based on the new observed data.
The Particle filter, on the other hand, is suitable for non-linear systems where noise may not be Gaussian.
It uses a set of particles to represent the state distribution, allowing for more flexibility in handling complex noise patterns.
The Particle filter updates these particles through weighting and resampling processes, effectively tracking the true state of the system over time.
Advantages of Bayesian Signal Processing
One of the significant advantages of Bayesian signal processing is its ability to provide a comprehensive framework for integrating prior knowledge and observational data.
This makes the resulting analysis both robust and adaptable to new information.
Another benefit is its inherent capacity to quantify uncertainty.
By working with probability distributions, Bayesian methods allow practitioners to assess the confidence in their estimates and make decisions accordingly.
Moreover, the adaptability of Bayesian methods to cope with a variety of noise types and distributions is invaluable.
This flexibility enables their application across diverse fields, ensuring effective noise removal even in challenging environments.
Challenges and Considerations
Despite its benefits, Bayesian signal processing also presents several challenges.
Computational complexity is a major concern, especially when dealing with large-scale problems and high-dimensional data.
Techniques like MCMC can be computationally intensive, requiring careful optimization to be feasible in real-time applications.
Additionally, selecting appropriate prior distributions is crucial.
Inaccurate priors can lead to biased results, so it’s essential that they reflect realistic assumptions about the data.
Finally, ensuring convergence in iterative methods such as MCMC and Particle filters can be challenging.
Users must verify that the algorithms are accurately representing the underlying probability distributions and are robust to the data’s variability.
Future Directions
The future of Bayesian signal processing is promising, with research focused on improving computational efficiency and algorithm robustness.
There is an increasing interest in developing hybrid methods that combine Bayesian techniques with machine learning and deep learning algorithms.
These novel approaches aim to handle large datasets and enhance noise removal capabilities further.
Furthermore, as sensors and data collection technologies advance, Bayesian methods will play an essential role in processing and interpreting increasingly complex data.
The continued evolution of these techniques promises to drive innovations across various sectors, propelling us toward new levels of data analysis and insight.
In conclusion, Bayesian signal processing provides a rich and robust framework for understanding and removing noise from signals.
Its application extends across various fields, offering reliable solutions for improving data quality and accuracy.
While challenges remain, ongoing research and development continue to strengthen its capabilities and potential for future advancements.
資料ダウンロード
QCD調達購買管理クラウド「newji」は、調達購買部門で必要なQCD管理全てを備えた、現場特化型兼クラウド型の今世紀最高の購買管理システムとなります。
ユーザー登録
調達購買業務の効率化だけでなく、システムを導入することで、コスト削減や製品・資材のステータス可視化のほか、属人化していた購買情報の共有化による内部不正防止や統制にも役立ちます。
NEWJI DX
製造業に特化したデジタルトランスフォーメーション(DX)の実現を目指す請負開発型のコンサルティングサービスです。AI、iPaaS、および先端の技術を駆使して、製造プロセスの効率化、業務効率化、チームワーク強化、コスト削減、品質向上を実現します。このサービスは、製造業の課題を深く理解し、それに対する最適なデジタルソリューションを提供することで、企業が持続的な成長とイノベーションを達成できるようサポートします。
オンライン講座
製造業、主に購買・調達部門にお勤めの方々に向けた情報を配信しております。
新任の方やベテランの方、管理職を対象とした幅広いコンテンツをご用意しております。
お問い合わせ
コストダウンが利益に直結する術だと理解していても、なかなか前に進めることができない状況。そんな時は、newjiのコストダウン自動化機能で大きく利益貢献しよう!
(Β版非公開)