- お役立ち記事
- Fundamentals of Bayesian statistics and applications to data analysis
月間76,176名の
製造業ご担当者様が閲覧しています*
*2025年3月31日現在のGoogle Analyticsのデータより
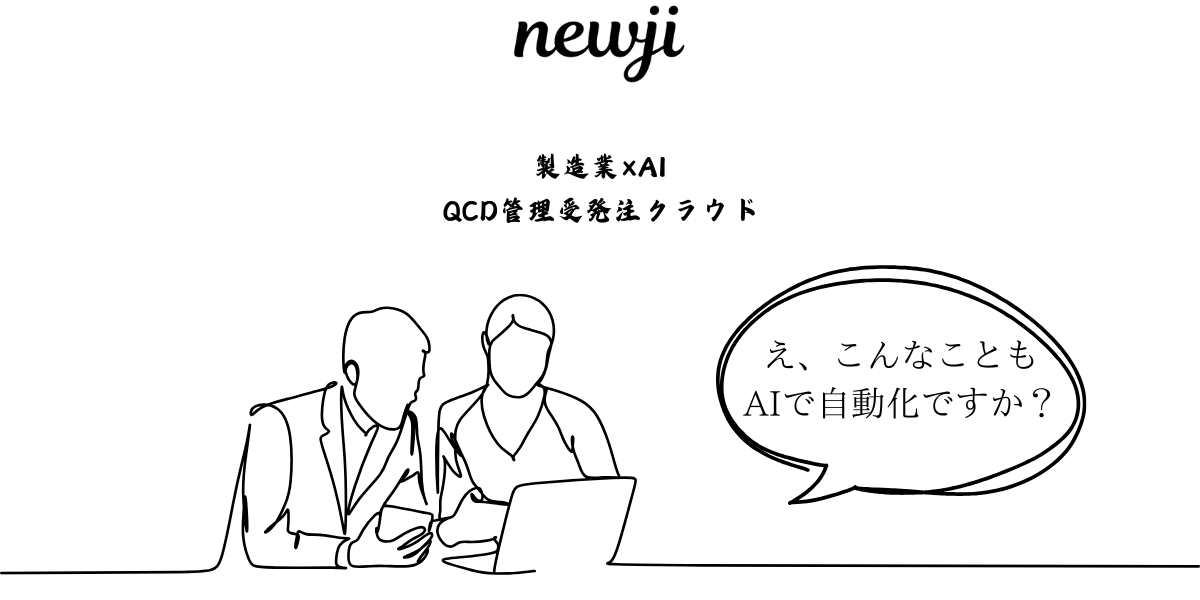
Fundamentals of Bayesian statistics and applications to data analysis
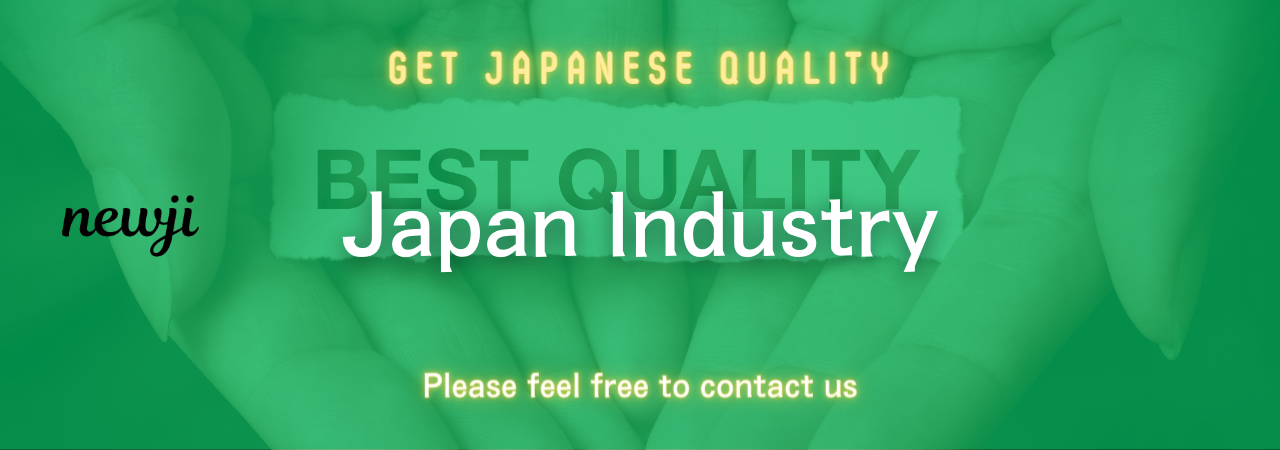
目次
Introduction to Bayesian Statistics
Bayesian statistics is a powerful and versatile approach to statistical analysis that allows for the incorporation of prior information into the data analysis process.
Unlike traditional frequentist methods, which rely solely on observed data, Bayesian statistics combines prior beliefs and empirical evidence to make inferences about unknown parameters.
This flexible framework is widely used in various fields, from medicine to machine learning, offering a robust way to handle uncertainty and make probabilistic predictions.
Understanding Bayes’ Theorem
At the heart of Bayesian statistics lies Bayes’ theorem, a fundamental principle that links prior knowledge with new evidence.
Bayes’ theorem can be expressed as:
\[ P(\theta | D) = \frac{P(D | \theta) \cdot P(\theta)}{P(D)} \]
Here, \( P(\theta | D) \) is the posterior probability of the parameter \( \theta \) given the data \( D \).
This represents our updated belief about the parameter after observing the data.
\( P(D | \theta) \) is the likelihood, which is the probability of observing the data given the parameter.
\( P(\theta) \) is the prior probability of the parameter, representing our initial belief before seeing the data.
Finally, \( P(D) \) is the marginal likelihood, acting as a normalizing constant to ensure the posterior is a valid probability distribution.
The Role of Prior Distributions
One of the key aspects of Bayesian statistics is the choice of prior distribution.
The prior reflects our initial beliefs about the parameter before observing the data.
Depending on the amount and quality of prior information, the choice of the prior can have a significant impact on the posterior distribution.
In some cases, where we have little prior information, a non-informative or weak prior may be used, effectively allowing the data to speak for itself.
Conversely, when prior knowledge is rich and reliable, a more informative prior can be used to guide the analysis.
Selecting an appropriate prior is both an art and a science, often requiring the expertise and judgment of the analyst.
Fortunately, Bayesian methods allow for sensitivity analysis, where different priors can be tested to assess their impact on the results.
Computational Methods in Bayesian Analysis
Bayesian statistics relies heavily on computational methods to derive the posterior distribution, especially when analytical solutions are difficult to obtain.
Modern Bayesian analysis often employs methods such as Markov Chain Monte Carlo (MCMC), variational inference, and other numerical approximations.
MCMC, in particular, is widely used for sampling from complex posterior distributions.
By generating a sequence of samples, MCMC methods such as the Metropolis-Hastings algorithm or the Gibbs sampler approximate the posterior distribution over time.
These samples can then be used to estimate parameters, make predictions, and quantify uncertainty.
Applications in Real-World Scenarios
Bayesian statistics shines in a variety of real-world applications where uncertainty is prevalent and data is complex.
Medicine and Healthcare
In medicine, Bayesian methods are frequently used to assess the effectiveness of new treatments.
By incorporating prior clinical trial data and expert opinions, Bayesian clinical trials can provide more efficient and ethical study designs.
Additionally, Bayesian statistics aids in diagnostic testing, adjusting probabilities of disease presence given prior information and test results.
Machine Learning and Artificial Intelligence
In machine learning, Bayesian inference facilitates the development of robust predictive models.
Bayesian neural networks, for instance, incorporate uncertainty in their predictions, leading to more reliable decision-making in critical applications such as autonomous vehicles and medical diagnosis.
Finance and Economics
Economists and financial analysts utilize Bayesian techniques to model uncertainties in markets, estimate dynamic models, and make forecasts.
By incorporating prior data and beliefs, Bayesian econometrics provides a framework for more accurate and adaptive financial models.
The Advantages of Bayesian Statistics
Bayesian statistics offers several advantages over traditional frequentist methods, making it an attractive choice for many analysts and researchers.
Flexibility in Modeling
Bayesian statistics is inherently flexible, allowing for complex models that capture real-world nuances.
By integrating prior information, it accommodates expert knowledge and contextual information that might not be present in the data alone.
Quantification of Uncertainty
Bayesian methods provide full probability distributions for parameters, enabling practitioners to quantify uncertainty explicitly.
This distributional understanding is particularly valuable for risk assessment and decision-making under uncertainty.
Intuitive and Cohesive Framework
The Bayesian approach aligns closely with the scientific method of updating beliefs based on new evidence.
This intuitive process allows for a cohesive interpretation of results that can be easily communicated to stakeholders.
Challenges and Considerations
Despite its advantages, Bayesian statistics is not without challenges.
One of the major hurdles is the computational complexity involved, particularly for large datasets or intricate models.
However, advancements in computational power and efficient algorithms continue to alleviate these concerns.
Moreover, the subjective nature of prior selection can be a point of contention, especially when priors significantly influence results.
Transparent reporting and sensitivity analyses help address these challenges.
Conclusion
Bayesian statistics offers a comprehensive framework for data analysis, combining prior beliefs with observed data to make informed inferences.
Its applications span numerous fields, from medicine to machine learning, reinforcing its importance in modern data-driven decision-making.
By embracing the uncertainty inherent in data and leveraging prior information, Bayesian methods provide richer insights and a robust approach to statistical analysis.
資料ダウンロード
QCD管理受発注クラウド「newji」は、受発注部門で必要なQCD管理全てを備えた、現場特化型兼クラウド型の今世紀最高の受発注管理システムとなります。
ユーザー登録
受発注業務の効率化だけでなく、システムを導入することで、コスト削減や製品・資材のステータス可視化のほか、属人化していた受発注情報の共有化による内部不正防止や統制にも役立ちます。
NEWJI DX
製造業に特化したデジタルトランスフォーメーション(DX)の実現を目指す請負開発型のコンサルティングサービスです。AI、iPaaS、および先端の技術を駆使して、製造プロセスの効率化、業務効率化、チームワーク強化、コスト削減、品質向上を実現します。このサービスは、製造業の課題を深く理解し、それに対する最適なデジタルソリューションを提供することで、企業が持続的な成長とイノベーションを達成できるようサポートします。
製造業ニュース解説
製造業、主に購買・調達部門にお勤めの方々に向けた情報を配信しております。
新任の方やベテランの方、管理職を対象とした幅広いコンテンツをご用意しております。
お問い合わせ
コストダウンが利益に直結する術だと理解していても、なかなか前に進めることができない状況。そんな時は、newjiのコストダウン自動化機能で大きく利益貢献しよう!
(β版非公開)