- お役立ち記事
- Fundamentals of Bayesian statistics and Bayesian modeling and applications and practices for data analysis and prediction
Fundamentals of Bayesian statistics and Bayesian modeling and applications and practices for data analysis and prediction
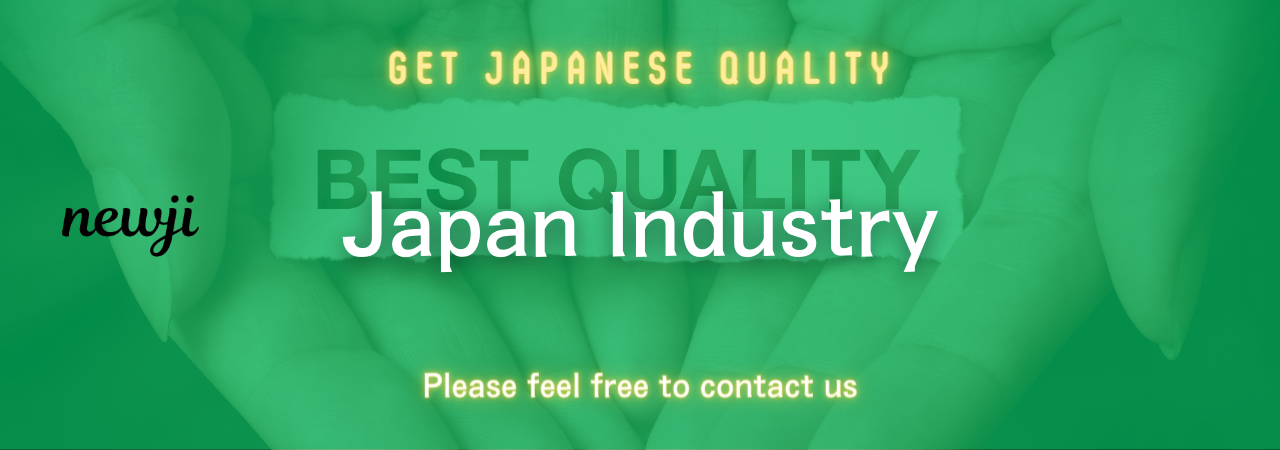
目次
Understanding the Basics of Bayesian Statistics
Bayesian statistics is a branch of statistics that interprets probability as a measure of belief or certainty rather than a frequency of occurrence.
It’s named after Thomas Bayes, an 18th-century statistician and minister.
At its core, Bayesian statistics focuses on updating the probability of a hypothesis as more evidence or information becomes available.
The fundamental theorem of Bayesian statistics is Bayes’ Theorem.
This theorem provides a mathematical formula for updating probabilities based on new data.
Given prior beliefs (known as prior probabilities) and new evidence, Bayes’ Theorem helps calculate what we call the posterior probability.
In simple terms, the theorem helps us revise our predictions or beliefs.
The Key Concepts of Bayesian Modeling
Bayesian modeling is the application of Bayesian statistics to build models that can make predictions or explain data.
These models incorporate prior knowledge and update it with new information.
In Bayesian modeling, every model parameter is considered a random variable.
This approach contrasts with classical statistics, where parameters are fixed but unknown.
Priors
In Bayesian modeling, a prior is the distribution that represents our uncertainty about a parameter before seeing the data.
The choice of prior can influence the model’s result, so it should be selected carefully.
There are several types of priors: informative, vague (or non-informative), and objective priors.
Informative priors contain substantial prior knowledge about a parameter, while vague priors specify minimal prior knowledge.
Objective priors are designed to minimize subjectivity.
Likelihood
Likelihood in Bayesian statistics is the probability of the observed data given a particular set of parameter values.
It helps us understand how plausible a set of data is within the constraints of a model.
Posterior
The posterior distribution is the updated belief after considering prior information and the likelihood from the observed data.
The posterior is what we are interested in and helps make predictions and decisions based on new evidence.
Conjugate Priors
A conjugate prior is a specific type of prior that, when combined with the likelihood, results in a posterior distribution of the same family.
Conjugate priors simplify the process of calculating the posterior, making Bayesian analysis more computationally efficient.
Applying Bayesian Statistics to Data Analysis
Bayesian statistics are powerful in various data analysis scenarios.
They provide an interpretable framework for incorporating prior knowledge and making inferences.
Predictive Modeling
Bayesian methods are highly effective in predictive modeling due to their ability to update predictions with new data iteratively.
For example, in weather forecasting, Bayesian models can integrate past climate data and current atmospheric conditions to predict future weather events.
Machine Learning
In machine learning, Bayesian approaches are prevalent for creating robust algorithms.
Bayesian inference can help in models such as Bayesian networks, Gaussian processes, and Bayesian neural networks.
These applications benefit from the adaptive nature of Bayesian learning, which naturally handles uncertainty and incorporates prior knowledge.
Decision Making
Bayesian statistics are useful for decision-making under uncertainty.
For instance, in drug development, Bayesian methods can assess the probability of a new drug’s efficacy based on clinical trial data, helping leaders make crucial go/no-go decisions.
Practical Applications of Bayesian Modeling
Bayesian modeling has applications across various industries, enhancing both analysis and prediction accuracy.
Healthcare
In healthcare, Bayesian models are used for personalized medicine.
They help doctors predict disease progression and tailor treatments to individual patients.
Bayesian methods can integrate genetic data, medical history, and lifestyle factors to inform treatment plans.
Finance
Bayesian modeling in finance aids in portfolio management and risk assessment.
Traders use these models to forecast stock prices based on historical data and market trends, adjusting strategies in real time.
Engineering
In engineering, particularly in systems like aerospace and robotics, Bayesian models predict system reliability and performance.
They can analyze sensor data to predict maintenance needs, optimizing resource allocation and reducing downtime.
Social Sciences
Researchers in social sciences use Bayesian analysis to explore complex datasets that include demographic, behavioral, and attitudinal variables.
Bayesian models provide insights into social behaviors and trends while accounting for uncertainty and variability.
Challenges and Considerations in Bayesian Statistics
Despite its advantages, Bayesian statistics also present some challenges.
A major consideration is the selection of priors, which can be subjective and influence results.
Moreover, computational complexity can be a hurdle, especially with large datasets or complex models.
Using sophisticated algorithms like Markov Chain Monte Carlo (MCMC), researchers can overcome computational limitations, allowing for efficient sampling from posterior distributions.
Bayesian statistics require a careful balance of prior knowledge and evidence, and understanding this balance is essential for accurate modeling.
Conclusion
Bayesian statistics and modeling provide a robust framework for data analysis and prediction.
By incorporating prior knowledge and updating it with new data, Bayesian methods offer a flexible and adaptive approach to understanding uncertainty.
From healthcare to finance and beyond, Bayesian modeling continues to enhance decision-making and strategy by offering a probabilistic approach to data analysis.
Learning and mastering Bayesian methods can significantly improve data-driven decision-making in many fields.
Despite the challenges, the rewards of incorporating Bayesian statistics into data analysis are substantial, leading to more accurate, reliable, and insightful predictions and conclusions.
資料ダウンロード
QCD調達購買管理クラウド「newji」は、調達購買部門で必要なQCD管理全てを備えた、現場特化型兼クラウド型の今世紀最高の購買管理システムとなります。
ユーザー登録
調達購買業務の効率化だけでなく、システムを導入することで、コスト削減や製品・資材のステータス可視化のほか、属人化していた購買情報の共有化による内部不正防止や統制にも役立ちます。
NEWJI DX
製造業に特化したデジタルトランスフォーメーション(DX)の実現を目指す請負開発型のコンサルティングサービスです。AI、iPaaS、および先端の技術を駆使して、製造プロセスの効率化、業務効率化、チームワーク強化、コスト削減、品質向上を実現します。このサービスは、製造業の課題を深く理解し、それに対する最適なデジタルソリューションを提供することで、企業が持続的な成長とイノベーションを達成できるようサポートします。
オンライン講座
製造業、主に購買・調達部門にお勤めの方々に向けた情報を配信しております。
新任の方やベテランの方、管理職を対象とした幅広いコンテンツをご用意しております。
お問い合わせ
コストダウンが利益に直結する術だと理解していても、なかなか前に進めることができない状況。そんな時は、newjiのコストダウン自動化機能で大きく利益貢献しよう!
(Β版非公開)