- お役立ち記事
- Fundamentals of Bayesian statistics/Bayesian inference and applications to data analysis
Fundamentals of Bayesian statistics/Bayesian inference and applications to data analysis
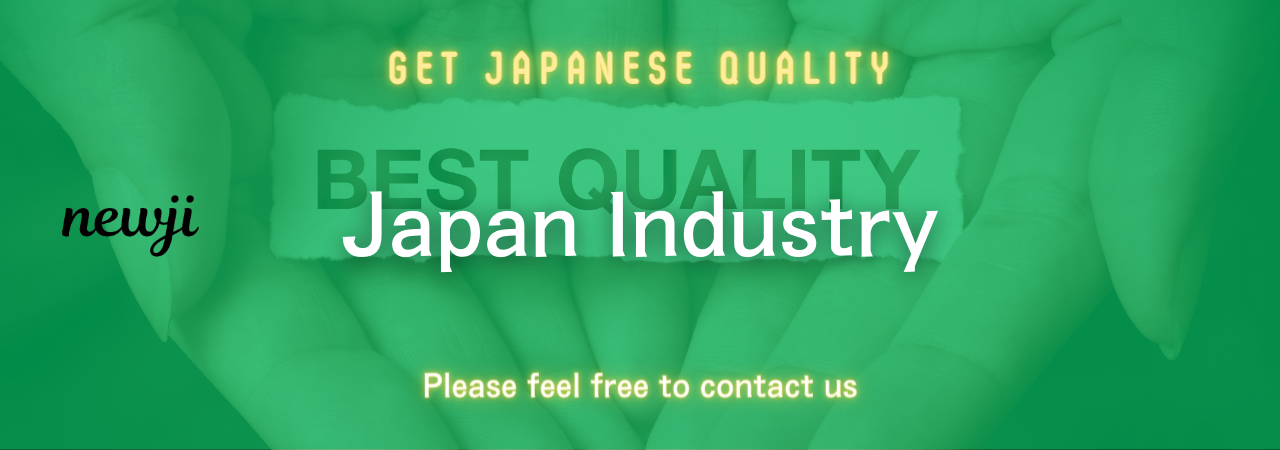
目次
Understanding the Basics of Bayesian Statistics
Bayesian statistics is a unique approach to statistics that incorporates prior knowledge along with current evidence to make informed decisions.
Named after the 18th-century mathematician Thomas Bayes, it’s a method gaining traction in various fields, notably in data analysis.
To comprehend Bayesian statistics, it’s essential to explore its foundational elements and how they can be applied to data analysis.
What is Bayesian Statistics?
At its core, Bayesian statistics revolves around Bayes’ Theorem, a mathematical formula that calculates conditional probabilities.
In more straightforward terms, it updates the probability for a hypothesis as more evidence or information becomes available.
This contrasts with traditional statistics, which typically rely solely on frequency data from observations.
Bayes’ Theorem can be represented as:
\[ P(A|B) = \frac{P(B|A) * P(A)}{P(B)} \]
Where:
– \( P(A|B) \) is the posterior probability: the probability of hypothesis A given the evidence B.
– \( P(B|A) \) is the likelihood: the probability of evidence B given that hypothesis A is true.
– \( P(A) \) is the prior probability: the initial probability of hypothesis A.
– \( P(B) \) is the marginal likelihood: the total probability of the evidence.
The Importance of Prior Knowledge
A distinct feature of Bayesian methodology is the incorporation of prior knowledge.
This prior knowledge, or prior probability, can be subjective or based on previous empirical data.
The process begins by establishing the prior probability, which is then adjusted using the likelihood of the observed data to form the posterior probability.
This means that Bayesian inference considers both the new data and the prior understanding, allowing for more flexible and dynamic decision-making.
This adaptability is especially significant in fields where prior information can heavily influence outcomes, such as in medical diagnoses or financial predictions.
Applying Bayesian Inference to Data Analysis
Bayesian inference provides a powerful framework for data analysis by allowing a more nuanced interpretation of data.
Unlike traditional methods, Bayesian inference accommodates uncertainty and variability, making it particularly useful for real-world applications.
Prediction and Decision Making
One of the most compelling applications of Bayesian inference in data analysis is its ability to make predictions and support decision-making under uncertainty.
For instance, in the financial sector, Bayesian models can be used to predict stock trends by updating the probability of different outcomes as new market data becomes available.
Similarly, in healthcare, Bayesian methods can help diagnose diseases more accurately by factoring in a patient’s history along with current test results.
Model Comparison and Validation
With Bayesian statistics, comparing and validating different models becomes more manageable.
Instead of relying on a single model, analysts can assess multiple hypotheses and incorporate new data to determine which model best explains the observed outcomes.
This process, known as model comparison, involves calculating the posterior probabilities of different models and selecting the one with the highest probability.
In practice, Bayesian model validation offers a robust approach to overcoming overfitting, a common issue in statistical modeling where a model performs well on training data but fails on unseen data.
By integrating prior knowledge, Bayesian statistics can provide insights into model performance that traditional methods might overlook.
Updating Beliefs with New Evidence
A hallmark of Bayesian inference is its iterative nature – beliefs or hypotheses are continually updated as new evidence emerges.
This is particularly advantageous in fields that involve rapidly changing data environments, such as technology and environmental sciences.
Consider climate modeling, where Bayesian methods can be employed to continuously refine projections based on the latest climate data.
As new data is integrated, predictions can be adjusted to reflect a more comprehensive and current understanding, ultimately leading to more accurate forecasts.
Real-World Applications of Bayesian Statistics
Bayesian statistics is not confined to theoretical discussions but finds extensive application across diverse industries.
Healthcare and Medicine
In healthcare, Bayesian statistics are used to improve the diagnosis process and treatment planning.
By incorporating patient history and test results, doctors can make more informed decisions about patient care.
For example, Bayesian models are used in clinical trials to continually assess the efficacy of a new drug and adapt the trial design as more data is collected.
Business and Economics
Businesses use Bayesian inference for market research and risk management.
By integrating market trends and consumer behavior data, companies can predict product demand and optimize marketing strategies.
In economics, Bayesian techniques enhance forecasting models, offering more accurate predictions of economic indicators like inflation or GDP growth.
Artificial Intelligence and Machine Learning
Bayesian methods are pivotal in training algorithms within artificial intelligence and machine learning.
In the data-driven landscape, these methods enhance machine learning applications by using prior distributions to make predictions even with limited data sets.
They help refine algorithms by updating the learning processes as additional data becomes available, improving the accuracy and reliability of AI systems.
Conclusion
Bayesian statistics, through its use of Bayes’ Theorem, offers a powerful and flexible framework for data analysis, inherently accommodating uncertainty and evolving information.
Its ability to incorporate prior knowledge alongside new data provides a comprehensive toolkit for decision-making across various domains including healthcare, business, and AI.
As data becomes increasingly central to our world, understanding and applying Bayesian statistics will remain an invaluable skill for researchers, analysts, and decision-makers alike.
資料ダウンロード
QCD調達購買管理クラウド「newji」は、調達購買部門で必要なQCD管理全てを備えた、現場特化型兼クラウド型の今世紀最高の購買管理システムとなります。
ユーザー登録
調達購買業務の効率化だけでなく、システムを導入することで、コスト削減や製品・資材のステータス可視化のほか、属人化していた購買情報の共有化による内部不正防止や統制にも役立ちます。
NEWJI DX
製造業に特化したデジタルトランスフォーメーション(DX)の実現を目指す請負開発型のコンサルティングサービスです。AI、iPaaS、および先端の技術を駆使して、製造プロセスの効率化、業務効率化、チームワーク強化、コスト削減、品質向上を実現します。このサービスは、製造業の課題を深く理解し、それに対する最適なデジタルソリューションを提供することで、企業が持続的な成長とイノベーションを達成できるようサポートします。
オンライン講座
製造業、主に購買・調達部門にお勤めの方々に向けた情報を配信しております。
新任の方やベテランの方、管理職を対象とした幅広いコンテンツをご用意しております。
お問い合わせ
コストダウンが利益に直結する術だと理解していても、なかなか前に進めることができない状況。そんな時は、newjiのコストダウン自動化機能で大きく利益貢献しよう!
(Β版非公開)