- お役立ち記事
- Fundamentals of brain morphic computing systems and next-generation edge AI and their realization technologies
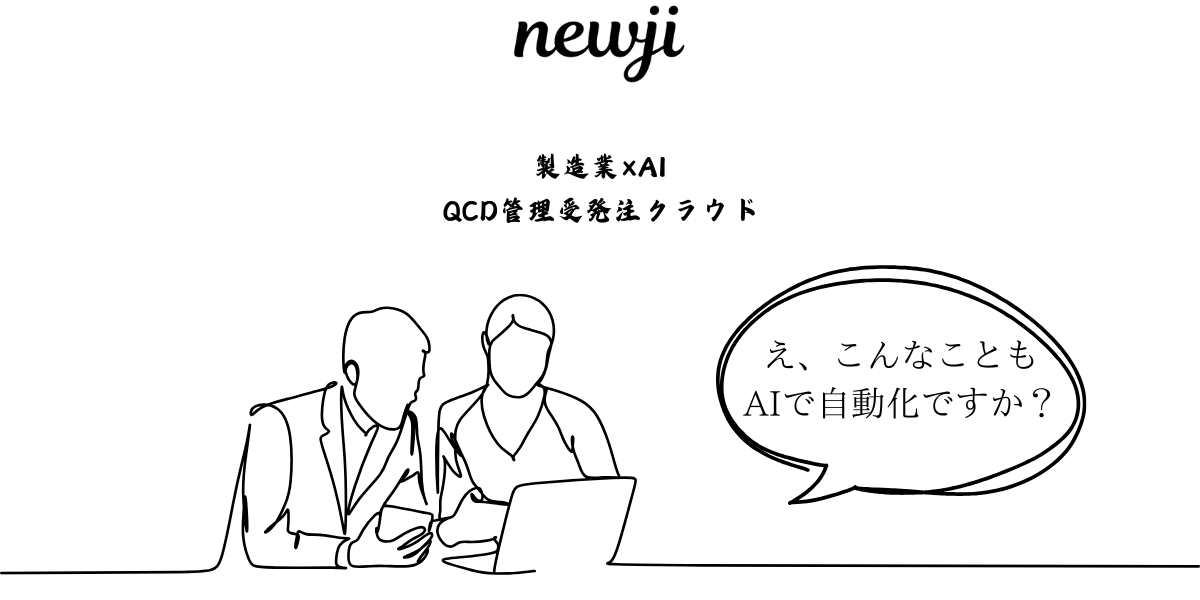
Fundamentals of brain morphic computing systems and next-generation edge AI and their realization technologies
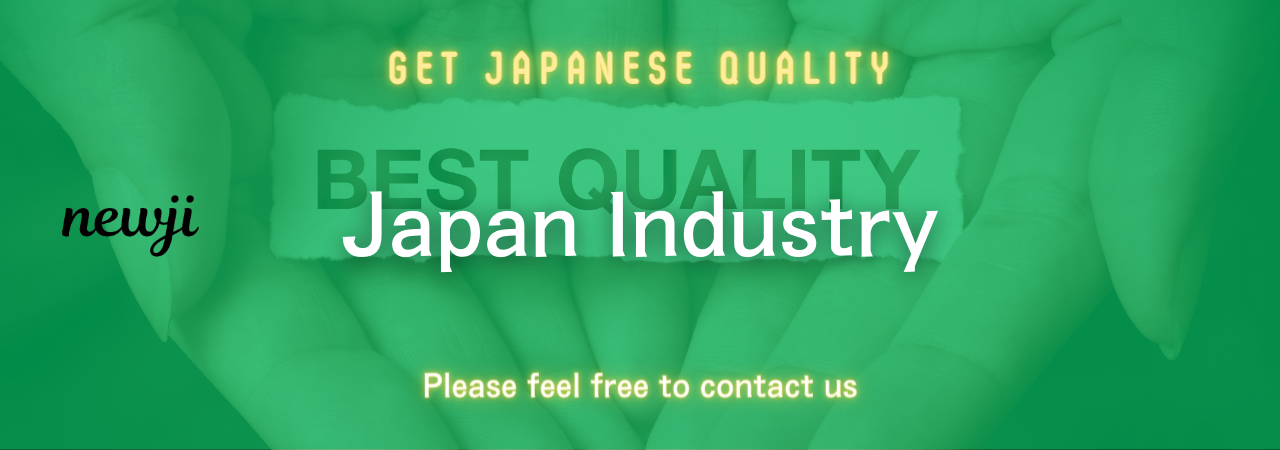
目次
Understanding Brain Morhic Computing Systems
Brain morphic computing systems are a novel approach to artificial intelligence (AI) that mimic the operations of the human brain.
This innovative technology is designed to enhance the capabilities of AI by incorporating the efficiency and adaptability of neural processing.
The main idea behind brain morphic computing is to create systems that can learn and adapt on their own, much like the brain does.
Brain morphic systems are not merely artificial neural networks; they go beyond by integrating the dynamic and parallel processing capabilities of biological neurons.
These systems aim to process information in a way that is more similar to natural intelligence, offering improvements in speed, efficiency, and energy consumption.
As the field of AI continues to progress, the importance of brain morphic computing grows, particularly in addressing complex real-world problems.
The Architecture of Brain Morhic Systems
The architecture of brain morphic systems is inspired by the structure and functionality of the human brain.
These systems consist of neurons and synapses that form networks capable of complex processing tasks.
Unlike traditional computing architectures, brain morphic systems utilize spiking neural networks (SNNs), which simulate the way real neurons communicate through electrical spikes.
Spiking neural networks offer several advantages, including higher processing speeds and reduced energy requirements.
These networks operate on the principle that neurons are only active when they have to be, significantly saving resources and increasing efficiency.
In addition, SNNs are inherently more robust and can handle noisy data with greater ease than conventional models, making them suitable for real-time applications.
Key Components of Brain Morhic Systems
The core components of brain morphic systems are neurons, synapses, and the network connectivity that mirrors biological systems.
Neurons in a brain morphic machine are units that process and transmit information through electrical signals.
These neurons are organized in layers, allowing for multi-level processing similar to how the human brain operates.
Synapses are the connections between neurons, enabling the transmission of signals.
In brain morphic systems, synapses can strengthen or weaken over time, allowing the system to learn from experience.
Through this plasticity, brain morphic systems can adapt to new tasks and environments with minimal additional programming.
The Role of Edge AI in Brain Morhic Computing
Edge AI refers to processing AI algorithms on local devices rather than relying on centralized cloud services.
Combining edge AI with brain morphic computing results in powerful and efficient systems capable of performing complex computations locally.
This integration is essential for applications requiring low latency and high responsiveness, such as autonomous vehicles and real-time monitoring systems.
The union of edge AI and brain morphic systems ensures that data is processed where it is collected, reducing communication delays and enhancing the system’s ability to act swiftly.
With the ongoing development of IoT devices and the emphasis on preserving privacy, edge AI becomes even more significant.
By processing data locally, sensitive information can be kept closer to its source, reducing risks associated with data breaches.
Benefits of Combining Brain Morhic Systems with Edge AI
The combination of brain morphic systems and edge AI boasts numerous advantages, chief among them being increased processing speed and reduced energy consumption.
These systems can handle tasks that require immediate data processing and decision-making without the need for extensive data transfers to a central server.
One of the most significant benefits is the ability to operate efficiently in environments with limited connectivity.
Since processing occurs locally, these systems are less reliant on robust internet connections, making them ideal for use in remote or resource-constrained areas.
Additionally, the reduced energy consumption of brain morphic systems aligns well with edge AI’s goal of sustainability, supporting eco-friendly energy usage.
Realizing Next-Generation AI Technologies
To realize the full potential of brain morphic computing systems and edge AI, significant advancements in hardware and software technologies are necessary.
Developing specialized hardware that can efficiently mimic the brain’s operations is crucial to supporting the unique requirements of these systems.
Hardware innovations such as neuromorphic chips and low-power microprocessors play a pivotal role in the success of brain morphic systems.
These chips are designed to replicate the brain’s architecture, enabling real-time data processing and learning capabilities directly on the device.
On the software side, the development of robust algorithms and programming paradigms that capitalize on brain morphic computing is imperative.
These algorithms must be adaptive, capable of handling diverse and dynamic data, and be optimized for resource-efficient execution.
The collaboration between hardware and software engineers is key to advancing this next-generation AI technology.
Future Prospects and Challenges
While the promise of brain morphic computing systems and edge AI is immense, it presents several challenges that must be overcome.
One of the primary challenges lies in the complexity of replicating the dynamics of biological neural systems.
Designing hardware and software systems that can seamlessly integrate these dynamics involves substantial research and experimentation.
Furthermore, there is the challenge of creating scalable brain morphic models that can function across various domains.
Ensuring that these systems are adaptable and versatile enough to tackle a wide range of applications must be a key focus for developers.
Nevertheless, the benefits of overcoming these challenges could revolutionize how AI technologies are deployed and utilized in the future.
Conclusion
The exploration of brain morphic computing systems combined with edge AI paves the way for innovative approaches to artificial intelligence.
By emulating the human brain’s ability to process and adapt, these systems offer promising advancements in speed, efficiency, and sustainability.
As technologies continue to evolve, the potential applications of brain morphic computing are vast, from autonomous systems to healthcare and beyond.
Investing in research and development of these promising technologies is pivotal as we move towards a future where intelligent systems play an increasingly central role in our lives.
As we address the technical challenges, the integration of brain morphic computing and edge AI will likely lead to a profound transformation in the landscape of AI and computing at large.
資料ダウンロード
QCD調達購買管理クラウド「newji」は、調達購買部門で必要なQCD管理全てを備えた、現場特化型兼クラウド型の今世紀最高の購買管理システムとなります。
ユーザー登録
調達購買業務の効率化だけでなく、システムを導入することで、コスト削減や製品・資材のステータス可視化のほか、属人化していた購買情報の共有化による内部不正防止や統制にも役立ちます。
NEWJI DX
製造業に特化したデジタルトランスフォーメーション(DX)の実現を目指す請負開発型のコンサルティングサービスです。AI、iPaaS、および先端の技術を駆使して、製造プロセスの効率化、業務効率化、チームワーク強化、コスト削減、品質向上を実現します。このサービスは、製造業の課題を深く理解し、それに対する最適なデジタルソリューションを提供することで、企業が持続的な成長とイノベーションを達成できるようサポートします。
オンライン講座
製造業、主に購買・調達部門にお勤めの方々に向けた情報を配信しております。
新任の方やベテランの方、管理職を対象とした幅広いコンテンツをご用意しております。
お問い合わせ
コストダウンが利益に直結する術だと理解していても、なかなか前に進めることができない状況。そんな時は、newjiのコストダウン自動化機能で大きく利益貢献しよう!
(Β版非公開)